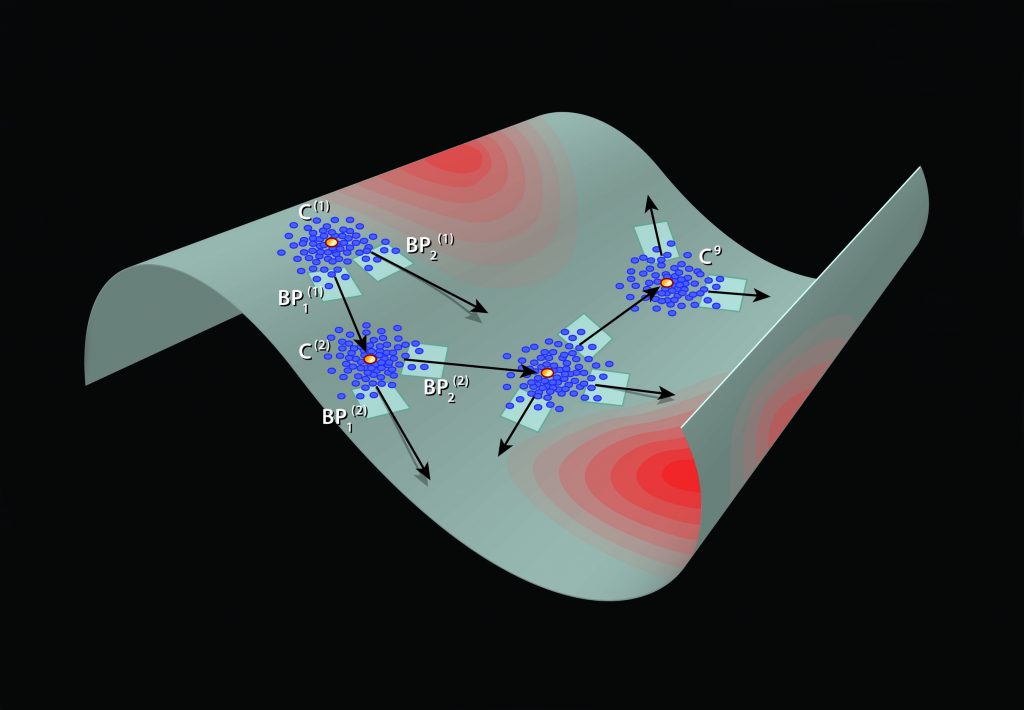
In the Middle Ages, apprentices became experts by amassing giant sets of experiences—now known as databases —and manipulating them without real understanding of the laws behind them, contends Yannis Kevrekidis, a Bloomberg Distinguished Professor with joint appointments in the Department of Chemical and Biomolecular Engineering, the Department of Applied Mathematics and Statistics, and the Department of Urology at the School of Medicine.
Kevrekidis, who came to Johns Hopkins in July after 30 years at Princeton, has set his sights on a different meaning of scientific understanding. This one can accommodate system complexity, like the interconnectedness of biological systems at the ecological and evolutionary levels that modern science has revealed.
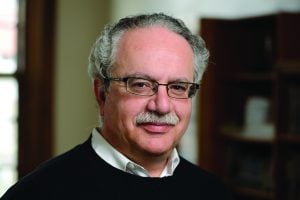
“The problem facing us now is how to find global solutions for complex systems, like integrated biological processes, when a detailed understanding in the Newtonian sense appears to be beyond the grasp of the human mind,” Kevrekidis says.
Since we may never again be able to collapse huge data sets as concisely as Isaac Newton did, Kevrekidis and his collaborators work on algorithms that exploit data to enhance, or even circumvent, conventional modeling of chemical and biological systems, and help scientists better predict system behavior—from reaction rates to materials properties. These data-driven algorithms aim to make predictions or even guide the experimental design for collection of new data, going directly from queries through data to predictions and sidestepping traditional analytical equations.
Recently, the group used machine learning techniques to intelligently bias molecular dynamics simulations that accelerate folding computations for proteins, elucidating the mechanism that controls saturated versus unsaturated lipid synthesis in yeast. In collaboration with researchers from Germany, Israel, and Yale University, the group also demonstrated the extraction of useful “quantities of interest” and dynamic equations connecting them—that is, the apparent discovery of physical laws from information-rich data—even when it was not known how the measurements correspond to physically important variables.
The process holds the promise of allowing the researchers to make data-driven predictions from sufficiently rich measurements even when a clearly understandable mechanism for the underlying physics is not available. It changes the focus from understanding physical mechanisms to understanding the algorithms that process the data to make predictions.
“While the skeleton of the modeling process remains the same, we are developing mathematical techniques that operate directly on observation data, and circumvent the need to select humanly meaningful variables and parameters and write equations,” Kevrekidis says.