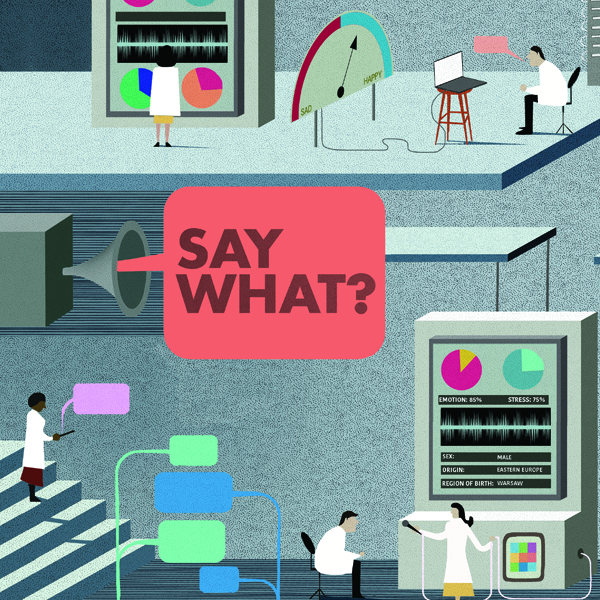
Think today’s computers are smart? Just look at what’s coming. Meet a multinational bullpen of computer scientists who are rapidly bridging the divide between humans and machines.
It all began with a plastic puppy named Radio Rex, a 2-inch bulldog with a battery in his bunk. Simply bark his name and out he would bound from his little wooden house. It was 1922.
“After Rex is in position,” read the instructions glued to the bottom of the toy, “either call sharply, REX, clap the hands, or blow a whistle of the proper pitch and Rex will respond by dashing forth, thus causing endless amusement to young and old.”
Ninety and more years ago, this must have seemed like magic—a device controlled by voice alone. (Even the invention of the amazing Clapper—“Clap on! Clap off!”—was still 60 years away.) But there was science behind the secret: The acoustic energy of the short “eh” sound, as spoken by a typical adult male at 500 hertz, triggered a spring in Rex’s rear that launched him out of his doghouse.
A century later, we hold a thin little gizmo in our hand and ask it, “Siri, what pitcher won the fifth game of the 1983 World Series for the Orioles?” Or we station a jet-black cylinder on our countertop and command it: “Alexa, send Beyoncé’s Lemonade to my sister as a birthday gift. And, by the way, we’re out of toilet paper.” And the wise and soothing genie inside the gadget makes it so.
Such is the work—and the wonder—of speech recognition and machine learning in its infancy: multiplying our fallible memories, fulfilling our mercantile wishes, and putting us on hold for miracles yet to come.
Already, we ask our automobiles to dial our telephones and to announce, turn by turn, the highway home. Move 10 years into the future and even more extraordinary advances become possible, as we and our children may live our lives accompanied by what David Yarowsky, professor of computer science at the Whiting School of Engineering and a member of the Johns Hopkins Center for Language and Speech Processing, calls “a computer and sensors presence that sees what you see, hears what you hear, knows what you read, and records every single thing that you encounter from the moment of your birth and remembers it forever.”
Picture yourself in a boat on a river and 20 years later being able to retrieve every detail of the day, every word, every sight, every splash.
“Ultimately,” says Yarowsky, “our computer assistants will be able to do more than our human assistants. I think we will become so dependent on the technology—sadly—that we won’t be able to function without them.
“We’ve already become so dependent on technology that I’ve stopped remembering phone numbers. I don’t know my own daughter’s number, and that’s scary.”
At the Johns Hopkins Center for Language and Speech Processing on the Homewood campus, a multinational bullpen of computer scientists is striving to enhance and perfect the voice-to-computer interface across a dazzling spectrum of tasks so that tomorrow’s devices will be able to discern when a combat veteran is depressed and suicidal; isolate and identify individual voices from the babel of a frenetic crime scene; retrieve every word ever spoken by or to anyone we ever encounter; and eliminate the pen, the mouse, the touchpad, and the keyboard forever, engineering a world of supercyber intelligences controlled by the spoken word alone.
Testing the Meaning of Understanding
“When I was a kid, I thought there was a lady in the radio,” Hynek Hermansky, the Julian S. Smith Endowed Professor of Electrical Engineering, says. He is the director of the CLSP and the proud owner of an original—and still working—Radio Rex toy, which he keeps on the windowsill of his office in Hackerman Hall. Today, that lady—Apple’s Siri, Amazon’s Alexa, Microsoft’s Cortana, et al.—may seems almost halfway human, but there are many problems yet to be solved before she truly is one of us.
“Human decoding of speech is really robust; we have many ways of looking at a speech signal. We can look at ones that work and happily discard the ones that don’t,” says Hermansky. “But we do not fully understand precisely what kind of errors people can make and still be understood.
“You can build a system for a computer to recognize individual words better than human beings can. But language is so flexible, so changeable, that to duplicate all that humans can do—understand context, recognize new words—is still very difficult.”
It has been more than 25 years since Hermansky, visiting his homeland (the former Czechoslovakia) just after the fall of its communist government, tried to use a rotary telephone to dial his office in the U.S. and the antiquated device was unable to connect to the relevant extension, which could only be reached by touch-tone.
The experimental speech recognition system in Hermansky’s laboratory saved the situation by being able to voice dial the required number.
Today, of course, we merely recite the number vocally and the robot operator understands and obeys.
“It was obvious even back then that we needed a better system of using our voices to control our computers,” he remembers. “Now, with Siri and Alexa and the others, I think that finally, our work is truly useful.”
At Johns Hopkins, the CLSP carries on the work of the late Frederick Jelinek, the Czech-American patriarch of computer speech processing. (It may not be a coincidence that Radio Rex responds just as eagerly to a shout of “Czechs!”) Alumni of the department have been central to the development of Google Voice, Alexa, and other dramatic advances in the inevitable marriage of humans and machines.
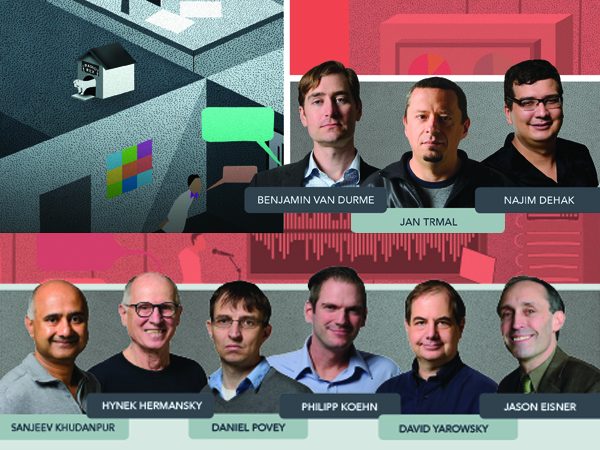
To Sanjeev Khudanpur, the Kannada-speaking native of Pune, India, and associate professor of computer science and of electrical and computer engineering, the achievements of the CLSP and its alumni “prove the supremacy of a system that rewards talent without prejudice.”
Although his role sometimes resembles that of a sheepdog trying to herd a dozen cats, Khudanpur sees in the CLSP “a combination of analytical talent and creative talent. It is a little bit like the human brain; some people are eccentric and crazy, and some people are like the glial cells—the glue that holds it all together.”
Fifty-five years ago, the pioneering American computer scientist Ida Rhodes wrote that “the heartbreaking problem that we face … is how to use the machine’s considerable speed to overcome its lack of human cognizance.” This conundrum still pulses in the heart of speech recognition.
“When someone asks, ‘Can computers really understand language?’ we have to have a test for the meaning of ‘understand,’” says Khudanpur. “Computers do not yet understand language in its fullest sense, but if we were only at the fifth-grade level before, we may be getting closer to the 10th grade now.”
One vexing problem, Khudanpur says, is the difficulty that existing speech recognition systems have in sorting out a cacophony of competing sounds; in his words, “it cannot yet be the fly on the wall, sorting out all the voices in the room, the air vent cycling on and off, the 4-year-old child crying, and the fly’s own buzzing.”
Khudanpur points to the snippet of a cellphone call that lay at the center of the Trayvon Martin shooting case in Florida in 2012 as an example of the shortcomings of the current state of his art. On the static-filled tape, a man cries “Help!” But forensic audiologists were unable to determine whether the voice was Martin’s or shooter George Zimmerman’s.
“These things still break our computers,” Khudanpur says, “but that problem is within reach. Deep neural networks (see the glossary) are starting to do things that we don’t understand. Given enough data, our computers may soon be able to figure out things that we cannot figure out. That’s very promising, isn’t it? To have a partner who can solve things that you cannot solve?”
Ultimately, solving the problem of translating one language into another with meaning, feeling, and nuance is the goal of Philipp Koehn, a German-born computer science professor at the CLSP, who is credited as being one of the inventors of a phrase-based translation approach.
“In general,” Koehn says, “people will come to expect that if you see or hear something in a foreign language, whether it is a street sign or a Facebook post or something on the radio or a YouTube subtitle, you can just click on it and have it translated.”
Currently, Koehn says, machine translation is achieving useful results for such texts as technical manuals and law books, but not poetry or literature. Asked if a computer today could deliver a workable translation of, say, a Harry Potter book, Koehn says, “It could, but there would be mistakes in every sentence.”
Code-Writing Masterminds
The work of bridging the divide between human and machine can be a deeply individual and intellectual pursuit, accomplished by code-writing masterminds working deep into the darkest hours of the night.
Among these is Czech-born Jan Trmal, an assistant research scientist who is challenged by another imperfection in automatic speech recognition: the fact that, even when a system can understand one language, it is useless when spoken to in another tongue.
Says Trmal: “The fact you have good American English ASR does not really help you in any way if you are facing the need to get a good ASR in a different language—say, Russian or Japanese. The more distant the languages grow, the more problems you would have with getting it to work. So the work on each ASR starts with collecting lots of training data,” which is time- and money-consuming.
“This is what I think would be of tremendous benefit—the ability to skip the training data collection part or, more precisely, to reduce the stage significantly,” he says. But, as any human who has tried to learn a second language knows, being fluent in Tagalog is of little value when trying to order a meal in Greek. For computers, the hurdle is even higher—to absorb and intuit not only vocabulary, but syntax and innuendo.
Jason Eisner, a computer science professor from New Jersey, wants to surmount that hurdle using machine learning. “Babies can puzzle out the structure of a language—both the pieces and how to smush them together,” he says. “They’re solving a great big statistical inference problem: ‘Why am I hearing what I’m hearing?'” Eisner tries to get his computers to crack various parts of that puzzle, and he’s developing new automated strategies each year.
Next door to Eisner, Trmal’s office mate is Assistant Research Professor Dan Povey, whose enterprise is the creation, in conjunction with computer scientists around the world, of an entirely new, open-source speech recognition programming infrastructure called Kaldi.
At its most basic level, Kaldi—and Povey—seeks to update Radio Rex by expanding the commands that he and his digital progeny can understand into the billions and beyond.
“The challenge is that the more possible inputs there are, the more difficult speech recognition becomes,” says Povey, who can be found most midnights bent over his laptop, subsisting on meals of microwaved potatoes, corn flakes, and a bottomless mug of green tea.
“Simple tasks, such as chess, are easy—‘rook to king 6’—or a Google search.
“Before deep neural networks came along, it was believed that speech recognition was at kind of a plateau. But now, with deep learning, it’s not really possible to say why a computer came to a certain conclusion, and that’s a property that it shares with the human brain.
“I don’t think we know whether there’s anything special about our brain,” Povey muses. “Maybe it’s not magic.”
This conjecture leads to a tantalizing—and controversial—question. If the human brain is not animated by some unique or divine spark, then is it possible that, by building a computer network with an equal number of connections, the brain could be copied onto silicon?
“The question is not whether it can be replicated,” Povey says. “The question is how long it will take.”
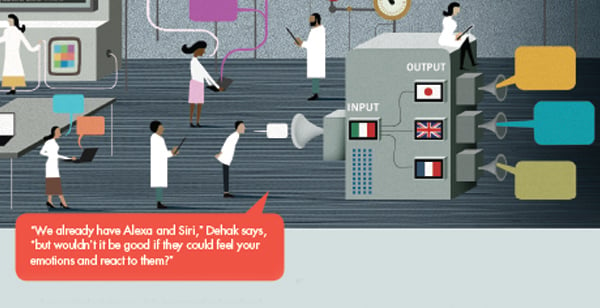
Learning to Infer
The biggest problem at this stage of speech-to-computer conversation, says Benjamin Van Durme, a native of upstate New York and assistant computer science professor, is that machines do not yet possess “a general understanding of how the world works.”
“Human beings know basic things about the world,” Van Durme notes. “We know that birds fly, that people blink and breathe and eat food and sleep. But an iPhone doesn’t know that if you push it off the desk, it will break.”
In the near term, Van Durme says, computers will become “very good at recognizing very explicit facts. But the ability to infer things that have never been described, that will take possibly 10 years, when we have these devices that follow all people around all of the time.”
The issue is the relative pokiness of the modern-day computer; according to Yarowsky, a machine needs to input 10 to 100 times more data to learn a new word or phrase than does a human child.
Says Van Durme, “As we have video cameras on every building, on every pair of glasses, on drones that are constantly over our heads—this totally pervasive video, feeding enormous amounts of data—then the computer will know.”
Siri and Alexa already can proclaim that it was pitcher Scott McGregor on the mound for the Baltimore O’s when they won the Series in ’83. But it is what they do not yet know—the human emotions that they cannot sense or feel—that is the inspiration for the work of CLSP’s Najim Dehak, a son of war-torn Algeria who is writing the code for what he calls “depression detection from speech.”
With so much of our lives now played out on social media, Dehak envisions a time when the content, keywords, and frequency of our tweets—or the tone of our voice when we order from Alexa—could serve as a trigger for professional intervention.
“If there is a soldier with PTSD,” says Dehak, an assistant professor of electrical and computer engineering, “we could create a system that can call him every day to see how he is feeling without having to wait for his next appointment.”
Dozens of machine learning specialists from around the world spent six weeks last summer at the CLSP’s famed summer workshop, started in 1997 and now called the Frederick Jelinek Memorial Workshop, discussing such hypotheses as the possibility of deducing suicidal intent from the frequency and keywords of a person’s tweets. This challenging topic—whether “clinically meaningful information can be derived from social media language”—echoes Dehak’s work on emotion recognition at the dawn of
the age of the social robot. Computers, he says, soon will learn to intuit sadness by contrasting it with billions of inputs from people who are happy.
“Wouldn’t it be good if [Alexa or Siri] could feel your emotions and react to them? We all want and need someone to talk to.” Such a system, Dehak suggests, would help people with autism as well as combat veterans. But there also is a commercial engine powering the rocket of speech recognition.
“Every company wants to know your behavior,” states Dehak. “That’s where the real money is. Imagine a single system that manages your phone, your car, your house—all controlled by your voice alone.”
Ethical Conundrums
At the CLSP, the accomplished specialists who toil away at the melding of human and artificial intelligences understand that Radio Rex is out of his doghouse—and it is too late to slide him back in. They also understand that they are building a pathway to a future of perpetual surveillance and robotic companionship—a future that no one truly can foresee.
“In about five years, we will have the capacity to capture every utterance,” predicts Van Durme. “As a scientist, yes, I would like access to that data and what it will enable. As an individual, it’s very concerning. But there’s no way around it.”
“I don’t think I want a computer to hear everything I say,” Dan Povey flatly states. “I’d want the ability to turn it off. Or maybe we could have an erase button. We are simply providing the technology to make it possible to be recorded all the time. It’s up to others to decide whether or not to use it.”
“We’re not doomed by this,” Khudanpur says. “I don’t worry about it. We will put in mechanisms. When we built nuclear weapons, we could all have been annihilated, but we’re still here.”
Will our machines know if we are lying?
“That’s a tough one,” he says. “A well-thought-out lie, I don’t think so.”
Will they be able to recognize not only what we are saying, but what we are thinking? “No,” Khudanpur replies. “What’s in your mind is only in your mind. So far.”