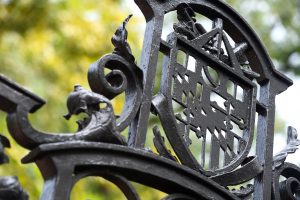
Antibodies—antigen-binding proteins widely used as therapeutics and as research tools—represent a huge field in biological research. Second-year master’s student Xiyao Long is among those best versed in one very particular corner of antibody research, as she creates machine learning models to predict the structural classes of the antigen-binding regions of the antibodies.
Long, who hails from the city of Shenzhen in China’s Guangdong province, earned her bachelor’s degree in biochemistry at the Illinois Institute of Technology and came to Johns Hopkins in pursuit of an engineering-based advanced degree. She hopes that her research will better refine the structural modeling of antibodies. Researchers often use this computational modeling in lieu of investing the time and money that physical experiments require.
“The effectiveness of the research comes from accurate bookkeeping of all the approaches I tried,” Long says. Other researchers have developed numerous methods to encode amino acids, but greater accuracy of the structural class prediction remains elusive. Long’s data set contains many cases in the antibody’s majority structure class and only a few cases in the small class, which challenges the application of machine learning. Long aims to find the methods that best circumvent those challenges.
“The most important part is that I defined the specific problems in current approaches to share with others,” she says.
Following her master’s degree, Long hopes to enter a PhD program in computational biology and then start a career in a computational-related technical field. From her experience at ChemBE, she expects to bring with her a hard-won approach of defining problems clearly and keeping good records; even when a method has not worked well, it can provide useful comparison data down the road, she points out.
Outside of the lab, Long can be found swimming, participating in a fitness class, or cooking. In December, she will present her poster about her research at the Women in Machine Learning (WiML) conference in Long Beach, CA.