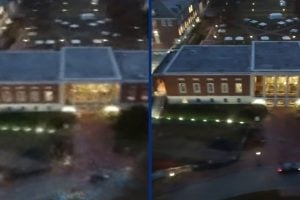
Johns Hopkins researchers have developed an efficient new method to turn blurry images into clear, sharp ones. Called Progressively Deblurring Radiance Field (PDRF), this approach deblurs images 15 times faster than previous methods while also achieving better results on both synthetic and real scenes.
“Oftentimes, images are blurry because autofocus doesn’t work properly, or the camera or the subject moves. Our method allows you to transform those blurry images into something clear and three-dimensional,” said Cheng Peng, a post-doctoral fellow in Johns Hopkins’ Artificial Intelligence for Engineering and Medicine Lab. “Applications could include everything from virtual and augmented reality applications to 3D scanning for e-commerce to movie production to robotic navigation systems — not to mention just being used to sharpen and deblur personal photos and videos.”
Peng worked with advisor Rama Chellappa, a Bloomberg Distinguished Professor in electrical and computer engineering and biomedical engineering, on the project. Their results, “PDRF: Progressively Deblurring Radiance Field for Fast Scene Reconstruction from Blurry Images,” appear in the Proceedings of the 37th Annual AAAI Conference on Artificial Intelligence.
The Milton S. Eisenhower Library at Night
Typically, the process of deblurring images involves two steps. First, the system estimates the positions of the cameras that took the blurry images, which allows it to insert the 2D images into the 3D scene. Next, the system reconstructs a more detailed 3D model of the scene pictured in the images or photos. While generally effective, these traditional methods have limitations, often resulting in artifacts—distortions and anomalies—and incomplete reconstructions. Neural Radiance Field (NeRF), a recent development in 3D image reconstruction, is successful in achieving photorealistic results but only if the input images are of good quality.
In contrast, PDRF can provide clear, clean images even with low-quality input images. The secret, Peng said, is that the new approach has the ability not only to detect and reduce blur in input photos but also to sharpen those images using what the team calls a “Progressive Blur Estimation module” before it creates 3D reconstructions of images or scenes.
“PDRF is based on neural networks and offers a fast self-supervised technique that learns from the inputted images themselves and does not require manually inputted training data. Remarkably, it addresses various types of degradation, including camera shake, object movement, and out-of-focus scenarios, showcasing its versatility,” he said. “In other words, we designed it to handle real-world situations and images.”
For instance, Peng and his team are working with researchers in the Department of Dermatology at the Johns Hopkins School of Medicine to use the new 3D modeling technology to enhance the detection of skin tumors, particularly neurofibromatosis: tumors that involve the brain, spinal cord, and nerves.
“In cases of neurofibromatosis, traditional measurement methods often prove challenging due to the tumors’ soft and deformable nature,” said Peng. “Our ongoing project seeks to address this by creating precise 3D models, allowing for accurate analysis of tumor volume, positions, and quantity. This innovative approach holds particular promise in telemedicine or telehealth scenarios, where patients can use their own cameras to capture affected areas with this method being beneficial in improving diagnostic accuracy.”
PDRF has been recognized with contract support from the Intelligence Advanced Research Projects Activity’s (IARPA) Walk-Through Rendering of Images of Varying Altitude (WRIVA) program, which aims to develop software systems to perform site modeling in scenarios where a limited volume of ground-level imagery with reliable metadata is available.
“Contracts like this allow us to apply these methods on a larger, city-wide scale. That is where we see the future direction of this going, which is large-scale reconstruction, and gets more into the mixed reality direction,” he said. “In the future, people will be able to explore faraway lands and cities in 3D based on 2D images captured by even just amateur photographers.”