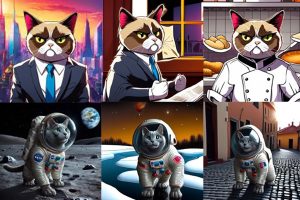
In a galaxy not so far, far away—Johns Hopkins’ Whiting School of Engineering—researchers have created JeDi, a new approach to personalized text-to-image generation that uses AI to allow users to produce customized images simply by typing what they want to see. What sets JeDi (Joint-Image Diffusion) apart from other such tools is its ability to remember details across the creation of multiple scenes, producing consistent, quality images quickly and efficiently.
“If you ask JeDI to create a picture of a Golden Retriever sleeping in front of the fireplace and another of a Golden Retriever catching a frisbee in the park, JeDi makes sure it is the same dog—same size, fur, etc.—in both images,” said co-creator Yu Zeng, a graduate student in the Department of Electrical and Computer Engineering.
The team presented “JeDi: Joint-Image Diffusion Models for Finetuning-Free Personalized Text-to-Image Generation” at the 2024 IEEE/CVF Conference on Computer Vision and Pattern Recognition last month.
A key factor behind JeDi’s improved performance is the way it was trained. “Whereas traditional text-to-image systems learn from single images, JeDi is trained on a special dataset called S3 (Synthetic Same-Subject), which contains sets of images of the same subjects in different poses and contexts,” says co-creator Vishal Patel, associate professor of electrical and computer engineering and instructor in the Engineering for Professionals’ ECE program.
“As a result, JeDi learns to understand the relationships between multiple descriptions and pictures of the same subject. For example, it learns from various images of red apples, along with different descriptions, such as ‘a shiny red apple’ and ‘a Red Delicious apple.’ This helps JeDi generate sets of related images, rather than just single images,” Patel said.
Experimental results show JeDi achieves state-of-the-art quality, surpassing existing methods—whether they use specialized training or not—even when given few reference images to learn from.
“Future research aims to combine JeDi with SceneComposer to simplify image creation,” said Zeng. “Users will be able to create images by ‘painting’ with brushes defined by text prompts or example images. Additionally, combining JeDi with fine-tuning could improve personalized image creation, especially with many example images, making the process more efficient and effective.”
Other contributors to the study included Haochen Wang from the Toyota Technological Institute, with Xun Huang, Ting-Chun Wang, Ming-Yu Liu, and Yogesh Balaji from NVIDIA Research.