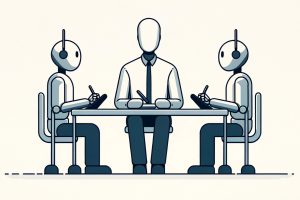
A team of Hopkins engineers has developed a method of improving cross-lingual annotation projection, a machine learning and natural language processing data creation technique that helps computers understand and process information across languages.
Cross-lingual annotation projection works by matching words that have been labeled with grammatical information (like parts of speech) in one language, such as English, with their unlabeled counterparts in a translated version of the same text, such as Spanish. It then transfers those labels to the corresponding words in the other language. This process helps the computer to learn the second language’s patterns or characteristics and how it is structured. This method of automatically creating labeled data in a second language is used in applications ranging from medical records to academic literature to web content.
Despite its potential, previous cross-lingual annotation projection methods face challenges: manual corrections to the synthetic data (those provided by humans) improves the data’s quality but are time-consuming and expensive, while fully automatic alignment, though cost-effective, lacks accuracy.
A team that includes Mahsa Yarmohammadi, an assistant research scientist in the Whiting School of Engineering’s Center for Language and Speech Processing, and Seth Ebner, a PhD student also with CLSP, propose a new, two-step strategy that addresses the trade-off between cost and quality: they first align words using two independent automatic alignment methods, then manually correct the cases in which the two automatic methods disagree. This strategy avoids the need for human annotation where automatic methods produce accurate results, optimizing cost-effectiveness and data quality.
They presented their approach, “The Effect of Alignment Correction on Cross-Lingual Annotation Projection,” at the 17th Linguistic Annotation Workshop in Toronto last summer.
“The combination of human annotations and automatic methods results in high-quality datasets and models for NLP, speech processing, and machine learning. Automatic methods ensure cost-effectiveness, while manual corrections contribute to improved accuracy,” Yarmohammadi said.
Ebner added, “Our approach reduces human effort and enhances overall data quality, which leads to a balanced trade-off between cost and model performance.”
In their study, the researchers investigated the impact of automatic and manual cross-lingual annotation projection on two tasks: understanding events and their participants discussed in sentences (shallow semantic parsing) and identifying specific kinds of entities in text (named entity recognition). Researchers compared fully automatic methods with human-labeled data in one task and used a dataset with annotations in two languages for the other. The researchers found that using models based on BERT, which utilizes the popular transformer model architecture for recognizing specific information in text and extracting details, together with human-verified data works better than relying solely on automatic methods.
“Our research highlights the need for human involvement in correcting alignment errors,” explains Yarmohammadi. “Though combining automation and manual corrections improves performance, ongoing human input is crucial for accuracy. We don’t foresee a time yet when machines can handle this task without human intervention.”
Other contributors to the study included Shabnam Behzad from Georgetown University, with Marc Marone and Benjamin Van Durme from the Whiting School of Engineering’s Center for Language and Speech Processing.