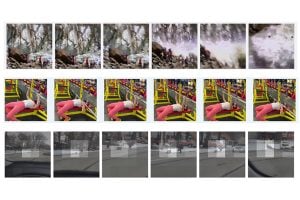
As online video content proliferates across various platforms, understanding viewers’ emotional responses has become increasingly important for a diverse group of stakeholders, from content creators and marketers to mental health experts and researchers. This insight can improve human-computer interaction, personalized services, and even support mental health initiatives. However, predicting viewers’ emotions remains a complex challenge due to the wide variety of video genres and emotional triggers involved.
Traditional video analysis methods focus on actions, expressions, and other visual cues, but they often overlook the emotional stimuli that drive human responses.
To address this gap, Johns Hopkins engineers have developed StimuVAR, an advanced AI system that analyzes videos to predict and explain how viewers might emotionally react to them. StimuVAR focuses on the specific elements in the video that are most likely to trigger emotional reactions. The paper, “StimuVAR: Spatiotemporal Stimuli-aware Video Affective Reasoning with Multimodal Large Language Models” is posted on arXiv.
“Understanding human emotional responses to videos is essential for developing socially intelligent systems,” said lead author Yuxiang Guo, a graduate student in the Whiting School of Engineering’s Department of Electrical and Computer Engineering.
Guo collaborated with Rama Chellappa, Bloomberg Distinguished Professor in electrical and computer engineering and biomedical engineering and interim co-director of Johns Hopkins Data Science and AI Institute; Yang Zhao, a graduate student from the Department of Biomedical Engineering at Johns Hopkins University; and researchers from the Honda Research Institute USA on this project.
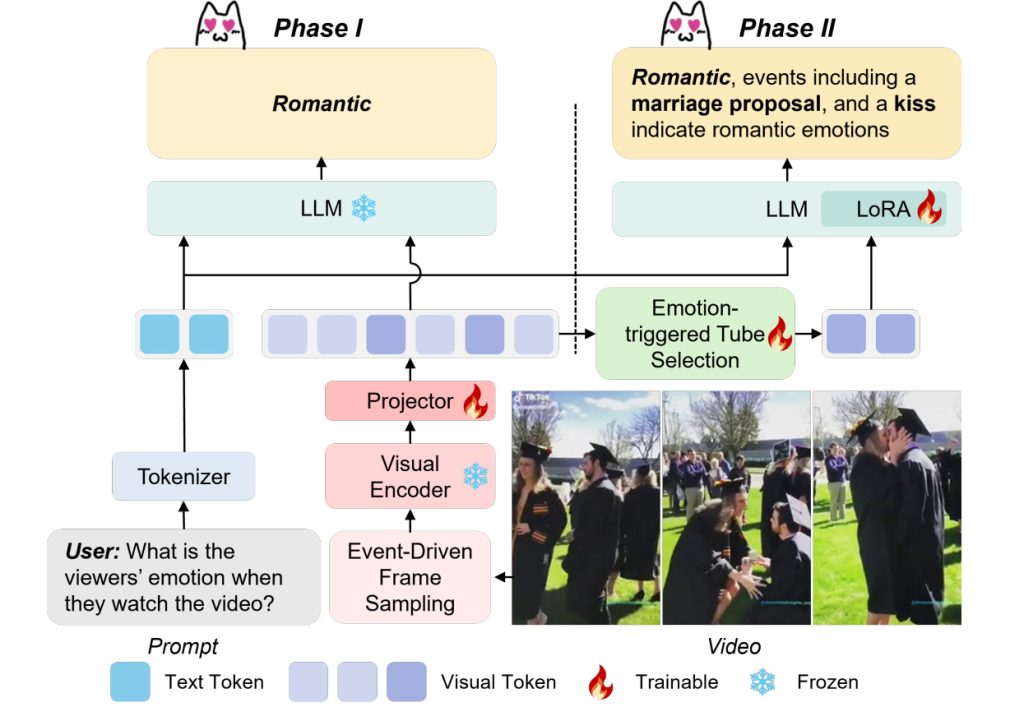
The architecture of StimuVAR. Event-driven frame sampling employs optical flow to capture emotional stimuli at the frame level, while emotion-triggered tube selection identifies key spatiotemporal areas at the token level, achieving effective and efficient video representations for VAR. To enhance affective understanding, we perform a two-phase affective training to steer MLLM’s reasoning strengths and commonsense knowledge towards an emotional focus, enabling accurate emotion predictions and plausible explanations.
One of StimuVAR’s key innovations is its affective training, which uses data designed to recognize emotional triggers. This allows StimuVAR to predict and understand emotional responses more effectively than traditional MLLMs, which focus primarily on videos’ surface-level content.
“For example, in a video of a dog reuniting with its owner, StimuVAR can identify the emotional high point—such as when the dog leaps into the owner’s arms—and explain why it triggers emotions like joy and nostalgia,” said Guo.
StimuVAR operates in two stages. First, it uses frame-level awareness to detect key emotional moments—like a surprise or heartwarming scene—in a video, treating each frame as an individual snapshot. Then, using token-level awareness, it analyzes specific details (or “tokens”) within those moments, focusing on the patterns and elements that are most likely to impact viewers’ emotions. This two-level approach enables StimuVAR to accurately pinpoint emotional triggers and provide coherent explanations for its predictions.
“StimuVAR’s ability to recognize emotional triggers has a wide range of potential applications, from helping AI assistants better understand and respond to user emotions and improving human-computer interaction to enhancing user experiences on entertainment platforms by recommending content based not just on viewing history, but also on how videos are likely to make viewers feel, recommending content that evokes specific emotions that the user wants to feel, ” said Guo.
Other contributors to the study include Faizan Siddiqui and Shao-Yuan Lo from the Honda Research Institute.