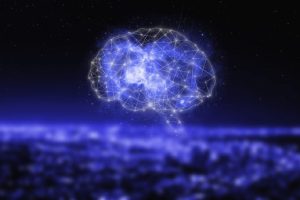
As inherently social creatures, humans can infer one another’s emotions and mental states from a range of sources: watching their actions, listening to their conversations, learning from their past behaviors, and so on. Cognitive researchers call this the “Theory of Mind,” or ToM—the ability to ascribe hidden mental states such as goals, beliefs, and desires to other individuals based on observed behavior.
Although it excels in many areas, artificial intelligence doesn’t match humans in this regard—at least not yet, according to an interinstitutional team including Tianmin Shu, an assistant professor of computer science at the Johns Hopkins Whiting School of Engineering. His team received an Outstanding Paper Award for its work, ” Multimodal Theory of Mind Question Answering”, at the 62nd Annual Meeting of the Association for Computational Linguistics last month.
“Understanding what others are thinking or feeling is crucial for developing machines that can interact with people in a socially intelligent way,” says Shu, who holds a secondary appointment in the Krieger School’s Department of Cognitive Science. “For example, a home robot needs this ability to figure out what someone might want or need so it can assist them more effectively in everyday life.”
To explore whether AI models can understand humans by using information from multiple sources, Shu and his team created the first standardized dataset that reflects the true complexities of the reasoning tasks encountered by real-world AI systems, such as AI assistants and robot caretakers. The team’s test set includes 134 videos and text descriptions of people looking for common objects in a household environment.
The researchers tested both humans and state-of-the-art large language and multimodal models on their ability to predict which objects the people in the videos wanted to find and where they believed they’d find them. The team found that humans became better at understanding others’ thoughts and intentions when they had access to varied sources of information. In contrast, even the most advanced AI models—such as OpenAI’s GPT-4V—struggled with such tasks, often confusing what was actually happening with what a person believed was happening and having difficulty tracking changes in people’s thoughts over time.
Based on these findings, the researchers created their own ToM model, which achieved far better results. Their approach first translates the video and text inputs into a type of notation it can understand, capturing the physical scene and the actions of the person within it. Then, instead of directly mapping these to the person’s beliefs and goals, the model uses a combination of Bayesian inverse planning—a cognitively grounded ToM method originally designed for visual data—and smaller language models fine-tuned on human activity data to infer the most likely possible actions given a person’s hypothetical mental state and the state of their environment.
“Our method shows promising results because it uses symbolic representations that work well across different types of information,” says Chuanyang Jin, a first-year PhD student advised by Shu. “It’s also robust thanks to an inverse planning approach that mimics human reasoning and can scale well and adapt to new scenarios because of the flexibility inherent in language models.”
All this results in a better performance on the team’s main test set and enables the model to generalize to real human behavior in situations it hasn’t been trained on. The researchers plan to expand on their work to include more diverse scenarios, human emotions, and situational constraints to better mimic the reasoning tasks that AI systems are likely to encounter in real life.
“Our research highlights important flaws in current AI models and suggests promising ways to improve them,” says Shu. “By sharing these insights, we aim to help others create AI models that can better understand and work alongside people, ultimately leading to machines that truly put humans at the center of their design.”
For more information about their work, visit the team’s project website.
Additional authors of this work hail from Harvard University; the Massachusetts Institute of Technology; the University of California, San Diego; and the University of Virginia.