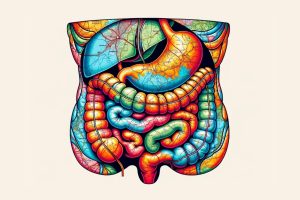
Medical segmentation, or the selection of specific regions of interest from medical scans, plays a vital role in the diagnosis and treatment of diseases like pancreatic cancer. Radiologists typically perform medical segmentation tasks manually—a laborious and time-consuming process. For instance, annotating a single abdominal organ typically takes a human radiologist anywhere from 30 minutes to an hour.
Computer scientists are training AI models to accelerate and improve this process. However, these models require a vast number of annotated medical images for proper training and testing and currently, the organ segmentation datasets available to researchers are constrained in both size and organ diversity, limiting the potential of the models trained on them.
But a group of Johns Hopkins researchers—joined by collaborators from Rutgers University, City University of Hong Kong, and NVIDIA—has developed a way to expedite this annotation process.
To prove their method’s capabilities, the researchers created AbdomenAtlas-8K, the largest multi-organ dataset in existence. At 3.2 million CT scan slices, it’s 8 times larger than the second-largest abdominal scan dataset out there. The researchers say that while a dataset of this size would have taken an experienced human annotator almost 31 years to fully annotate, they completed this task in only three weeks—with equal or even better annotation quality—by leveraging the strengths of human medical professionals and AI algorithms.
“Our proposed active learning procedure can significantly accelerate the annotation process, as demonstrated by the creation of the AbdomenAtlas-8K dataset,” say Wenxuan Li and Chongyu Qu, master’s students in the Department of Computer Science who are advised by Alan Yuille, a Bloomberg Distinguished Professor of cognitive science and computer science and the director of the Computational Cognition, Vision, and Learning group. “In turn, AbdomenAtlas-8K can help AI algorithms improve organ annotation accuracy and reduce the burden placed on human radiologists. This is part of an initiative to transform the reproducibility of AI algorithms in organ segmentation across diverse clinical settings and patient demographics.”
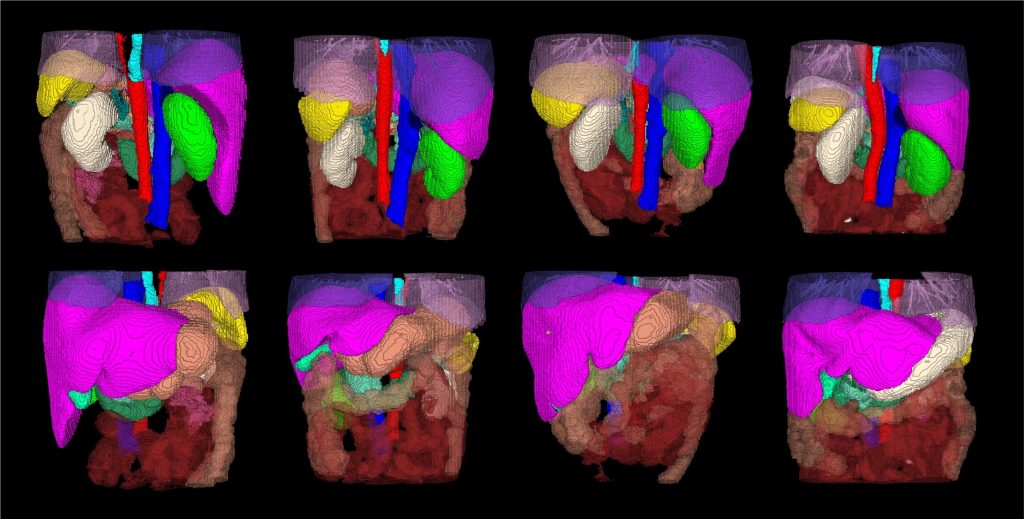
AbdomenAtlas-8K annotations.
First, the researchers trained a combination of three different AI models—to minimize any bias stemming from the models’ architectures—on public datasets of labeled abdominal scans. They then had the AI models predict annotations for several preexisting unlabeled datasets.
The team then selected only the most important sections of the models’ predictions and used color-coordinated attention maps to show human radiologists which areas to focus on in their manual review of the AIs’ work. Repeating this process—AI prediction and human review—over and over allowed them to accelerate the annotation process by an impressive factor of 533, the team says.
According to the researchers, the quality of the final AI annotations is comparable to those produced by experienced human annotators. Meanwhile, the resulting dataset itself holds promise in training other AI algorithms in tasks like cancer detection.
“Our dataset provides detailed per-voxel annotations for eight abdominal organs,” says Zongwei Zhou, a postdoctoral researcher in the Computational Cognition, Vision, and Learning group. “Any AI models trained on our dataset to automatically identify and delineate the boundaries of these organs can be easily transferred to cancer-related tasks—all with less need for manual annotations.”
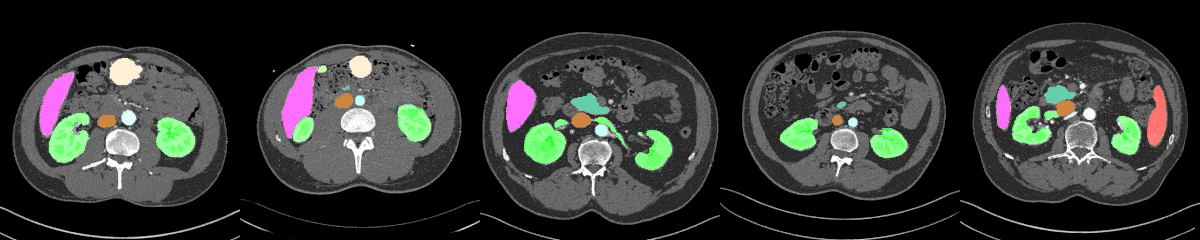
AbdomenAtlas-8K annotations throughout the abdomen.
Although the team’s current AI models falter when there are tumors present in the CT scan data, the researchers have a plan to improve their models’ performance by recruiting additional experienced radiologists for manual review, incorporating pathology reports into the human revision process, and exploiting synthetic tumor data to further train their algorithms to detect tumors large and small. To this end, the research team is actively seeking collaborations across academic institutions, industry sectors, and health care facilities, says Zhou.
The team presented its research as part of the Track on Datasets and Benchmarks at the Thirty-Seventh Conference on Neural Information Processing Systems in December, while an extended version of their work—which provides detailed annotations for 25 abdominal structures, including the pancreas—has been accepted as an oral presentation at the 12th International Conference on Learning Representations, a distinction awarded to only 1.2% of publications at the venue.
This research was also selected for an international competition hosted by the 21st IEEE International Symposium on Biomedical Imaging; the research team’s challenge asks competitors to utilize the AbdomenAtlas-8K dataset to train medical segmentation AI algorithms for generalizability and efficiency.
“As you can see, things are moving fast for our AbdomenAtlas project,” says Zhou.
Additional co-authors of this work include Tiezheng Zhang, a graduate student in the Department of Electrical Engineering; Hualin Qiao of Rutgers University; Jie Liu, a PhD student at the City University of Hong Kong; and Yucheng Tang, a research scientist at NVIDIA.