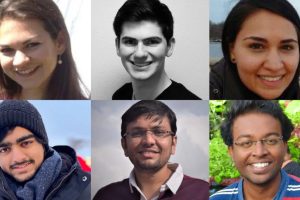
Amazon and Johns Hopkins University (JHU) today announced the first recipients of PhD fellowships and faculty research awards as part of the JHU + Amazon Initiative for Interactive AI (AI2AI).
The AI2AI initiative, launched in April and housed in JHU’s Whiting School of Engineering, is focused on driving ground-breaking AI advances with an emphasis on machine learning, computer vision, natural language understanding, and speech processing.
“We are delighted by the high quality of proposals and PhD fellowship nominations from JHU faculty and students,” said Prem Natarajan, vice president of Alexa AI. “There is no question this initiative will drive new advances in the state-of-the-art in interactive and multimodal AI.”
As part of the initiative, annual Amazon fellowships are awarded to PhD students enrolled in the Whiting School of Engineering. Amazon also funds research projects led by JHU faculty in collaboration with post-doctoral researchers, undergraduate and graduate students, and research staff. This year’s recipients mark the inaugural class.
“We are excited that our students and faculty have a chance to partner with Amazon in an area important as interactive AI,” said Larry Nagahara, Johns Hopkins University Whiting School of Engineering’s Vice Dean for Research and Translation. “Leveraging our collective expertise in this area will advance AI and bring many beneficial aspects to our society.”
Below is a list of the fellows, and their research, followed by the faculty award recipients and their research projects.
Kelly Marchisio is pursuing a PhD in computer science, studying under Philipp Koehn, a professor of computer science.
“Word embedding spaces are a critical component of modern natural language processing systems. My work focuses on understanding and exploiting embedding space geometry, with the goal of creating spaces that are smaller, more useful, and more universally applicable across languages and domains.”
Arya McCarthy is pursuing a PhD in computer science, studying under David Yarowsky, a professor of computer science.
“I call my vision for natural language processing, kilolanguage processing: not only modeling thousands of languages but also letting their collective evidence and commonality reinforce each other. To make it happen, I’ve created neural machine translation models; morphological lemmatizers, taggers, and inflectors; and even a thorough analysis of color terminology spanning thousands of languages, aiming to push those frontiers further. This vision is driven by the realities of speaker needs and how NLP fails to meet them today. There are about 7000 identified languages in the world, at least 4000 of which have a book-length digitized written presence. Despite this availability of data, standard NLP tools are available for often far fewer than 100.”
Carolina Pacheco Oñate is pursuing a PhD in biomedical engineering, studying under René Vidal, an Amazon Scholar and the Herschel Seder Professor of Biomedical Engineering.
“I am interested in advancing computer vision to domains with limited availability of data or annotations, which is relevant not only in long-tail events within traditional computer vision tasks, but also in other socially impactful areas such as biomedical sciences. I believe that the combination of deep learning with probabilistic models and domain knowledge can provide the right balance between capacity and structure, enabling learning from limited amounts of data in self- and weakly-supervised regimes.”
Desh Raj is pursuing a PhD in computer science, studying under Sanjeev Khudanpur, associate professor of electrical and computer engineering.
“Since the first automatic speech recognition systems were built more than 30 years ago, improvement in voice technology has enabled applications such as automated customer support and language learning. Through years of research on speech enhancement and robust speech processing, these systems are now deployed in diverse settings such as on home speakers and vehicle controls. Nevertheless, these present systems are passive listeners which transcribe single-speaker utterances and feed into downstream language understanding components. Conversational intelligence of the future is expected to comprise systems that can actively participate in human conversations. While such systems would require intelligence in diverse modalities — dialog systems for context handling, emotion recognition from speech and video, common sense reasoning, to name a few — their ability to recognize free-flowing multi-party conversations is a core component that needs to be solved.”
Anshul Shah is pursuing a PhD in computer science, studying under Rama Chellappa, Bloomberg Distinguished Professor in electrical and computer engineering and biomedical engineering.
“My current research is broadly in the area of pose-based action recognition, video understanding, self-supervised learning and multimodal learning. My research tries to make fundamental contributions to these research areas, obtains new insights and pushes the state of the art. My interests closely align with AI2AI’s focus in areas of interactive AI technologies specifically in the areas of computer vision and multimodal AI.”
Jeya Maria Jose Valanarasu is pursuing a PhD in electrical and computer engineering, studying under Vishal M. Patel, associate professor of electrical and computer engineering.
“Deep learning methods for computer vision have made remarkable progress in field visual recognition. One major reason for its success is the amount of data these models are trained on. Annotating new ground truths for every new problem or application is very inefficient. Also, current vision systems perform poorly on data distribution that it has not seen during training. This problem is called domain adaptation and is important to solve for deploying models in real-time. Also, when the model is adapted to new data during inference, the adaptation needs to be fast and it does not make sense to train the model at test-time. Thus, we need to focus on few-shot or better zero-shot learning for adaptation.”
Faculty research awards
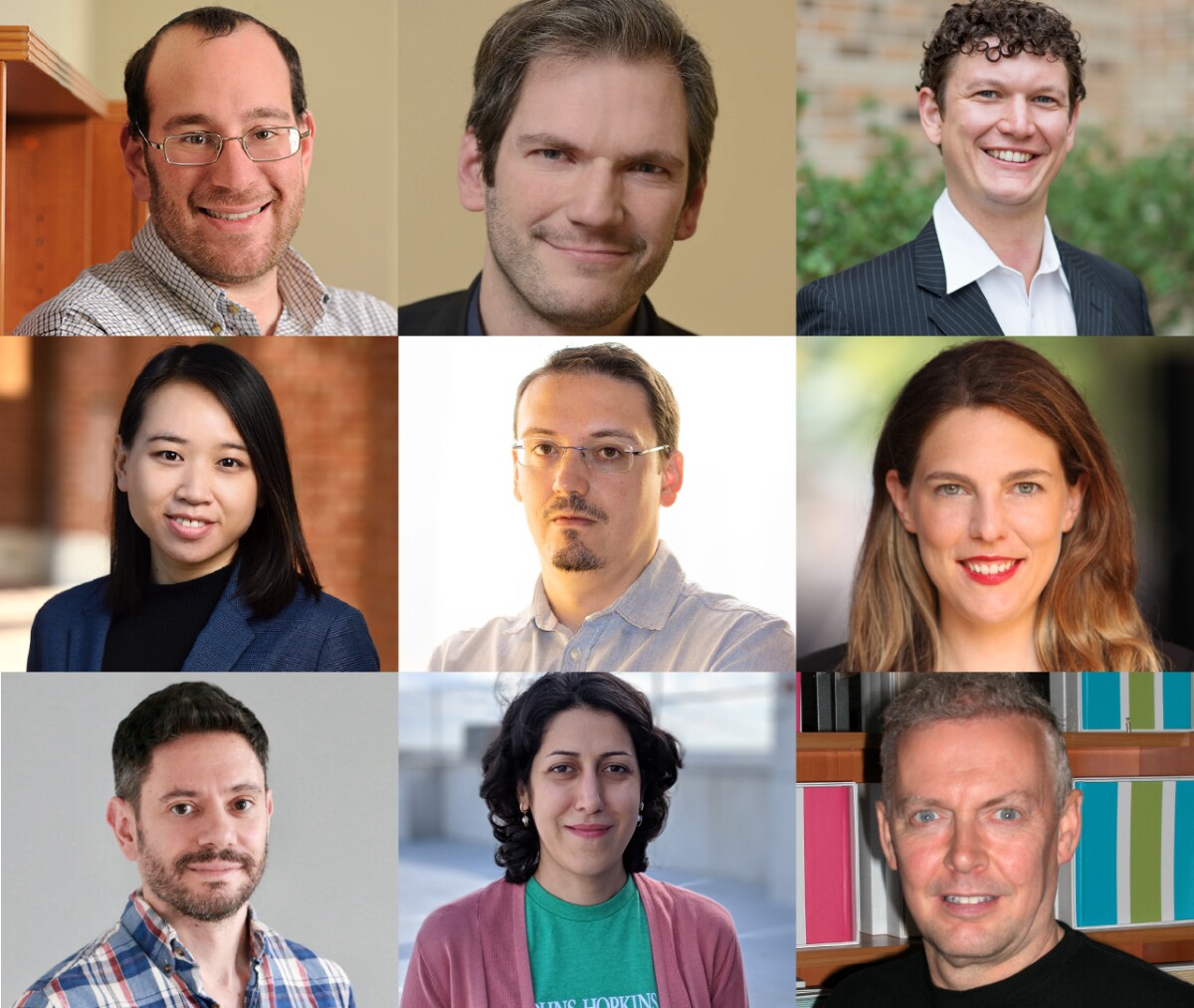
Top row, Mark Dredze, Philipp Koehn, and Kenton Murray; second row, Anqi Liu, Jesus Antonio Villalba López, and Soledad Villar; bottom row, Laureano Moro-Velazquez, Mahsa Yarmohammadi, and Alan Yuille
Mark Dredze, John C. Malone Associate Professor of Computer Science: “Integrating Knowledge Representation of LLMs with Information Extraction Systems”
“In the past few years, new types of AI models that capture patterns in language have become very good at learning information from language. This project explores how we can use information learned by these models to inform practical applications on language data, such as identifying important features or characteristics of products in product reviews. This award will allow us to push the limits of language modeling by exploring how we can use recent advances to help improve various applications of language technologies.”
Philipp Koehn, professor of computer science, and Kenton Murray, research scientist in the Human Language Technology Center of Excellence: “Evaluating the Multilinguality of Multilingual Machine Translation”
“The proliferation of deep neural networks into artificial intelligence has allowed researchers and engineers to build systems that can automatically translate between large groups of languages without having to build separate models. However, the limitations of having one large, general model are not well understood. We aim to investigate the cutting-edge frontiers of this class of AI models.”
Anqi Liu, assistant professor of computer science: “Online Domain Adaptation via Distributionally Robust Learning”
“This project aims to enable fast and robust adaptation for AI algorithms via modeling uncertainty. This award makes it possible for me to work on fundamental research questions that have the potential for real-world impact.”
Jesus Antonio Villalba López, assistant research professor of electrical and computer engineering, “Generalist Speech Processing Models”
“This project will investigate how to efficiently extract the information contained in speech using large-scale AI models. The outcome will be a generalist model able to transcribe speech into text, and determine the speaker’s identity, language, and emotional state, among others.”
Soledad Villar, assistant professor of applied mathematics and statistics: “Green AI: Powerful and Lightweight Machine Learning via Exploiting Symmetries”
“In this project we investigate the use of symmetries and low-dimensional structures in the design of machine learning models. Enforcing these mathematical structures will allow us to reduce the energy consumption, time, and amounts of data required for training and evaluating machine learning models while preserving (or even improving) their performance.”
Laureano Moro-Velazquez, assistant research professor, Center for Language and Speech Processing: “Improving Spoken Language Understanding for People with Atypical Speech”
“In this project we will create a new dataset and develop new speech technologies meant to improve the lives of individuals with atypical speech and speech impairment. There are almost no publicly available datasets containing atypical speech, and these are necessary to create new assistive technologies for the affected population. This award will allow us to create such dataset which will be useful for us and for many other groups researching atypical speech.”
Mahsa Yarmohammadi, assistant research scientist, Center for Language and Speech Processing: “Rapid Multilingual Dataset Creation with Automatic Projection and Human Supervision”
“Artificial intelligence in general, and natural language processing in particular, require a massive scale of data to learn strong models. Such data might not be available in languages other than high-resource ones such as English. In this project, we study the rapid creation of multilingual datasets by automatically translating and aligning an available dataset in one language into multiple other languages. We will also study the impact of human supervision in improving data quality. Once we have created these resources, we intend to use them to co-train single multilingual models for cross-lingual NLP tasks.”
Alan Yuille, Bloomberg Distinguished Professor of Cognitive Science and Computer Science, “Weakly-Supervised Multi-Modal Transformers for Few-Shot Learning with Generalization to Novel Domains and Fine-Grained Tasks”
“Self-supervised and weakly supervised transformers have been shown to be highly effective for a variety of vision, language, and vision-language tasks. This proposal targets three challenges. First, to improve performance on standard tasks, particularly on fine-grained tasks (e.g., object attributes and parts), which have received little study. Second, to develop tokenizer approaches to enable few-shot, and ideally zero-shot, learning. Third, to adapt these approaches so that they are able to generalize to novel domains and to out-of-distribution situations. We propose five strategies to achieve these goals which include extending the tokenizer-based approaches, modifying the transformer structure, increasing the text-annotations to help these difficult tasks, and techniques for enabling the algorithms to generalize out-of-domain and out-of-distribution.”