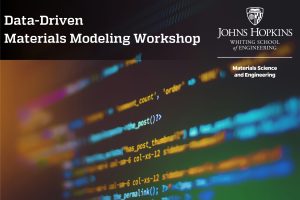
The Institute for Data-Intensive Engineering & Science (IDIES), The Ralph O’Connor Sustainable Energy Institute (ROSEI) and the Advanced Research Computing at Hopkins (ARCH) Facilities are sponsoring a 3-day workshop on data-driven materials modeling at the Homewood Campus in Baltimore, MD. Registration is $20 and will cover lunch and snacks for each day.
Wednesday, May 29th 2024 – Friday, May 31st 2024
Daily overview:
Join us for 3 full-day sessions with themes that explore the intersection of simulation with machine learning and artificial intelligence algorithms. Each session will feature a morning keynote from an expert in the field and a hands-on afternoon covering relevant software and running through exercises.
Note to attendees: Please bring a laptop to each session and create a Google Drive account if you do not already have one. The team will be using Google Colab for many of the exercises.
Wednesday, May 29, 10am – 5pm: “High-Throughput Thermodynamic Modeling”, with keynote speaker Olexandr Isayev from Carnegie Melon University.
- 10-10:15am: Workshop welcome
- 10:15-11:15am: Keynote Seminar with Dr. Olexandr Isayev
- 11:15-12pm: Seminar by Dr. Corey Oses
- 12-1pm: Lunch
- 1-5pm: Workshop led by Drs. Guotao Qiu, Yuxiang Liu, Tianhao Li, and Xiao Xu on high-throughput density functional theory calculations with aflow++, thermodynamic analysis, structural prototypes, and modeling disordered materials.
Thursday, May 30, 10am – 5pm: “Quantum Chemistry and Catalysis”, with keynote speakerJohn Keith from the University of Pittsburg.
- 10-11am: Keynote Seminar with Dr. John Keith
- 11-12pm: Seminar by Dr. Brandon Bukowski
- 12-1pm: Lunch
- 1-5pm: Workshop led by Dr. Brandon Bukowski on running density functional theory calculations with GPAW, ab initio molecular dynamics, and using NequIP to generate machine learning potentials for temperature-dependent properties.
Friday, May 31, 10am – 5pm: “Monte Carlo and Molecular Dynamics”, with keynote speaker Erik Luijten from Northwestern University.
- 10-11am: Keynote Seminar with Dr. Erik Luijten
- 11-12pm: Seminar by Drs. Michael Falk and Tine Curk
- 12-1pm: Lunch
- 1-4:30pm: Workshop led by Drs. Vicente Munizaga and Turash Haque Pial on predicting fracture with molecular dynamics, Monte Carlo elements for alloy equilibration, and random forest classification and regression.
- 4:30-5pm: Workshop conclusion
Keynote speakers:
Day 1:
Artificial Intelligence (AI) Solutions for Computational and Materials Chemistry Tasks
Olexandr Isayev
Department of Chemistry, Carnegie Mellon University
Abstract:
Deep learning is revolutionizing many areas of science and technology, particularly in natural language processing, speech recognition, and computer vision. In this talk, we will provide an overview of the latest developments in machine learning and AI methods and application to the problem of molecular design at Isayev’s Lab at CMU. We identify several areas where existing methods have the potential to accelerate computational chemistry research and disrupt more traditional approaches. First, we will present a deep learning model that approximates the solution of the Schrodinger equation. Focusing on parametrization for organic and element-organic molecules, we have developed a single ‘universal’ model, which is highly accurate compared to reference quantum mechanical calculations at speeds 10^6 faster. These models accurately represent the underlying physical chemistry of molecules through various test cases, including chemical reactions (thermodynamics and kinetics), thermochemistry, structural optimization, and molecular dynamics simulations. The results presented in this talk will provide evidence of the universal applicability of deep learning potentials to various chemistry problems involving organic molecules.
Second, we proposed a novel ML-guided materials discovery platform that combines synergistic innovations in automated flow synthesis and automated machine learning (AutoML) method development. A software-controlled, continuous polymer synthesis platform enables rapid iterative experimental–computational cycles that result in the synthesis of hundreds of unique copolymer compositions within a multi-variable compositional space. The non-intuitive design criteria identified by ML, accomplished by exploring less than 1% of overall compositional space, upended conventional wisdom in the materials design. We are currently enhancing this platform with Reinforcement learning (RL) agents. Under the RL paradigm, an agent(s) is trained to select actions that maximize the cumulative sum of rewards, which, in the context of chemical discovery, is often consistent with a target property, structural feature, or function. By training via value-based or policy-based iterative schemes, RL agents can learn to suggest synthesis protocols, potential reactants, and experimental conditions.
Day 2:
Physical (and AI/ML amenable) paradigms for computationally interrogating atomic scale reaction mechanisms
John A. Keith
Department of Chemical and Petroleum Engineering, University of Pittsburgh
This talk will provide an overview of our group’s recent work in understanding and developing computational chemistry methods to elucidate atomic scale chemical reaction mechanisms across chemical compound space. I will show work describing calculation schemes that use quantum alchemy for computational catalysis, atomic properties, and bond energy predictions. I will also describe progress toward the development of Bond Energy from Bond Orders and Populations (BEBOP) methods, classes of semi-empirical methods that can provide a) approximate atomization energies based on interatomic bond energy decompositions and b) approximate zero-point energies, and more, all based on just one single quantum chemistry calculation for high quality orbital populations. Outlook for improving all these methods’ accuracy, lowering their computational cost, and extending their usefulness across chemistry and materials science will be discussed.
Day 3:
Combining Advanced Simulations and Experiments: A Path Toward Understanding Collective Phenomena
Erik Luijten
Department of Materials Science and Engineering, Northwestern University
In this lecture, I will describe how advances in computational materials science permit new insights into collective phenomena. Notably, I will discuss close collaborations between simulation and experiment that have resulted in significant progress in nanoparticle crystallization, tunable supramolecular hierarchical self-assembly, and colloidal aggregation phenomena.
REGISTER HERE
For questions, please reach out to Dr. Corey Oses at [email protected].