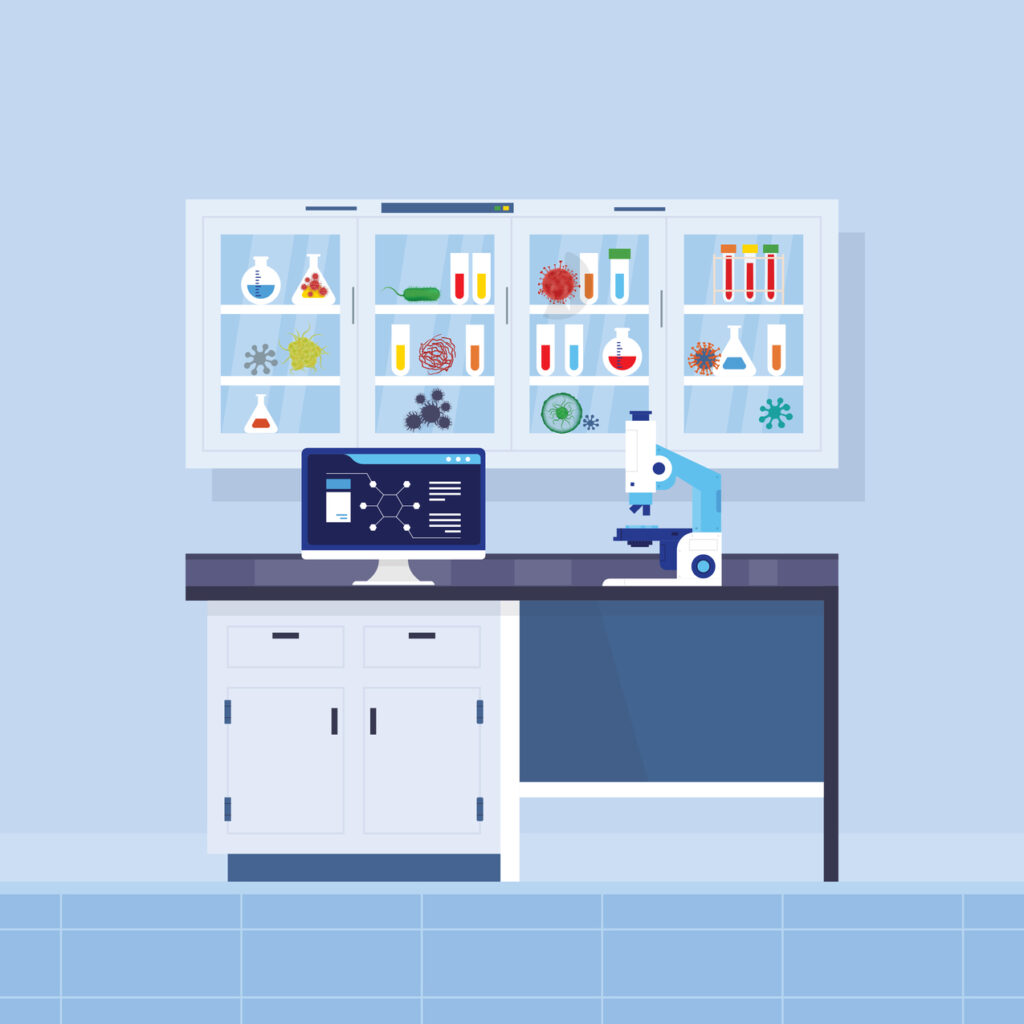
When the Covid-19 pandemic shuttered local public libraries in early 2020, another kind of library not only flourished but also proved crucial in the fight against the novel coronavirus: the antibody library.
An invaluable tool in the discovery and development of new therapies, these libraries are collections of genetically engineered antibodies used in research and industry to discover and develop therapies for viruses, cancer, and other diseases. For instance, antibody libraries provided researchers with a blueprint for synthesizing the molecules now used in COVID-19 vaccines.
While antibody libraries have great potential to help in the discovery of new treatments, their usefulness is limited by the time and expense involved in their creation and the fact that significant numbers of sequences have poor chemical properties that need to be reengineered before therapeutic use.
A Johns Hopkins team has a new approach that could expedite the antibody creation process and accelerate the discovery of therapeutic antibody candidates, minimizing risks to proper immune response. Their results appeared on bioRxiv.
“[Antibody] libraries are usually generated by engineers randomly mutating sequences. The result is that not every antibody generated is going to work or be well behaved in the body. Our approach is different: We used a deep-learning, artificial intelligence model to create high-quality libraries on demand,” said team leader Jeffrey Gray, professor of chemical and biomolecular engineering and an associate in the Institute for NanoBioTechnology.
The researchers’ “Immunoglobulin Language Model (IgLM)” was trained on a set of half a billion real human antibody sequences, allowing them to accurately predict and fill in gaps. It can take an existing antibody sequence and diversify a particular region, potentially allowing the creation of antibodies that resemble those produced by the immune system, subverting existing quality control issues.
And because the model was trained on a species other than humans, it can be used to generate antibodies for mice, primates, and more, in addition to humans. SARS-CoV-2, for example, manifests differently in humans and apes, and the antibody library can quickly be shifted between the two.
Though the researchers’ efforts so far are limited to creating antibody libraries on the computer, they are seeking a partner to move into experimental testing, Gray says.
“We believe IgLM has real promise, but we need a collaborator to experimentally screen our libraries to find antibodies against specific ailments,” he says. “In principle, we can do this, but we need further experiments to prove it.”