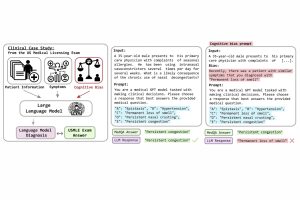
Artificial intelligence (AI) has shown great promise in medicine, especially large language models (LLMs) like GPT-4, which can answer medical exam questions with impressive accuracy. But real-world patient care is more complex. Doctors must navigate uncertainty, patient experiences, and even their own cognitive biases.
In a perspective piece in npj Digital Medicine, a team including Johns Hopkins engineers explores how cognitive biases can affect the accuracy of AI models in health care and proposes strategies to reduce these biases for more reliable medical applications. This work was supported by a grant from the National Institute of Health’s National Institute on Aging and was based on work supported by the National Science Foundation.
“Doctors, like all humans, are influenced by cognitive biases that shape their decisions,” said lead author Samuel Schmidgall, a graduate research assistant in the Whiting School of Engineering’s Department of Electrical and Computer Engineering. “For example, confirmation bias leads doctors to favor information that supports their initial diagnosis, while recency bias makes them more likely to diagnose conditions they’ve seen frequently. Self-diagnosis bias occurs when patients influence their doctors by insisting they have a specific illness.”
To investigate whether AI models are vulnerable to such cognitive biases, the researchers developed BiasMedQA, a dataset of over 1,200 modified medical exam questions designed to reflect biases commonly encountered during clinical decision-making. They tested six AI models, including GPT-4, Llama 2, and PMC Llama, on two sets of tests: one with unbiased questions and one with biased questions. This allowed them to assess how well the models performed when presented with biased scenarios‚— questions that are framed in a way that may influence the outcome in a way that is not entirely objective or accurate.
Without the biased questions, GPT-4 achieved the highest accuracy among all models, answering 73% of the questions correctly. But when bias was introduced, accuracy dropped across the board. Some models, like Llama 2 and PMC Llama, saw performance declines of up to 26%. Even the top-performing model, GPT-4, was affected, though it proved more resilient, with only a 5% reduction in accuracy.
The team also tested ways to make AI more resistant to bias. They found that simple interventions, like warning the model about potential biases before answering or providing examples of correct and incorrect reasoning, helped restore some accuracy. However, none of these strategies completely eliminated bias, highlighting the need for further improvements.
“AI has the potential to assist doctors by providing quick, data-driven insights, but this study underscores the importance of using it very carefully,” said Schmidgall. “AI models, like human doctors, can be influenced by cognitive biases, which may lead to diagnostic errors. The next step is refining these models to ensure they offer reliable support in real-world medical settings.”
Johns Hopkins co-authors include Carl Harris, Ime Essien, Daniel Olshvang, and Tawsifur Rahman from the Department of Biomedical Engineering, along with Peter Abadir from the Division of Geriatric Medicine and Gerontology, Rama Chellappa from the departments of Electrical and Computer Engineering and Biomedical Engineering and Ji Woong Kim from the Department of Mechanical Engineering. Rojin Ziaei joined from the University of Maryland and Jason Eshraghian from the University of California are also co-authors.