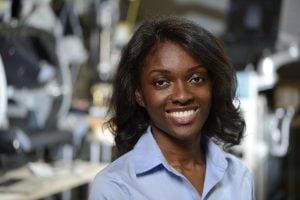
ECE Assistant Professor Muyinatu Bell is conducting pioneering research that integrates deep learning with ultrasound image formation to provide interventional radiologists with a fundamentally new type of ultrasound image. These novel images only display structures of interest, such as a needle tip as it is inserted into the kidney and the corresponding kidney target location, to address traditional and often problematic ultrasound image quality issues. Bell is collaborating with Austin Reiter, PhD and Kelvin Hong, MD to pursue this research with a NIH Trailblazer Award from the National Institute of Biomedical Imaging and Bioengineering.
The proposed approach is the first of its kind to use data-driven methods to replace the traditional ultrasound image formation process, which is often referred to as beamforming. Several aspects of the traditional beamforming process cause ultrasound images to be plagued with multiple confusing artifacts that complicate the completion of interventional tasks, such as the removal of painful kidney stones. These artifacts exist because of assumptions that are not always true for each patient. Examples of incorrect assumptions include a uniform sound speed for all patients, the absence of reverberations and reflection artifacts, and single direct pathways for both transmitted and reflected sound waves to and from a target of interest. In addition to multiple possible variations between patients, there are also multiple variables within the same patient that contribute to poor ultrasound image quality.
This work challenges existing paradigms by completely bypassing the traditional beamforming process, which heavily relies on the faulty, model-based assumptions described above. Instead, the recorded raw ultrasound channel data is converted into human-readable images by learning from examples and recreating features learned during training. One eyebrow-raising, yet scientifically proven, component of this research is that the examples for training can be created with physically realistic ultrasound simulations. Success has already been achieved when transferring the learned parameters from simulated data to real experimental data. The ultimate goal is to develop the framework required to apply this same process to patient data.
The three-year, $624,000 award from the NIH is titled “A Machine Learning Alternative to Beamforming to Improve Ultrasound Image Quality for Interventional Access to the Kidney.”