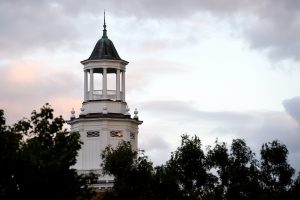
The Johns Hopkins University’s Discovery Awards provide grant awards to cross-divisional teams, comprised of faculty and/or non-faculty members from at least two schools or affiliates of the university, who are poised to arrive at important discoveries or creative works. The expectation is that these awards will spark new, synergistic interactions between investigators across the institution and lead to work of the highest quality and impact.
The awardees for this year’s Discovery Awards were recently announced, and the Department of Electrical and Computer Engineering had two primary faculty members named: Associate professor Amy C. Foster, and assistant professor Archana Venkataraman, who is involved in two Discovery Award projects.
See below for the titles, abstracts, and brief blurbs about the projects.
Preparation of Ultra-high Optical Quality Silicon Photonic Waveguides using Confined VLS Growth– Amy Foster (ECE) & Thomas Kempa (Arts & Sciences)
Abstract: For decades, the preparation of silicon photonic elements has relied on traditional top-down microfabrication methods originally developed for CMOS technology. This top-down approach enables high-confinement photonic devices with sophisticated layouts in the planar chip geometry. However, the inherently rough surfaces generated from the requisite reactive-ion-etching limit the silicon photonics devices to losses that are 6 orders of magnitude larger than standard single-mode optical fibers. These losses are prohibitively high and preclude wider adoption of silicon photonic devices for next-generation communication applications. Here we will pursue a completely new bottom-up process to grow crystalline silicon photonic waveguides with ultra-high-quality surfaces that can approach the fundamental loss limit. This has the potential to revolutionize silicon photonics research with devices whose losses are 1-2 orders of magnitude lower than the State-of-Art.
Harnessing Machine Learning to Optimize Stroke Critical Care Resource Use– Roland Faigle (Medicine) & Archana Venkataraman (ECE)
Blurb: Patients with ischemic stroke undergoing mechanical thrombectomy (MT) constitute the majority of stroke-related ICU admissions. While these patients are at risk for clinical deterioration and life-threatening complications, there is little evidence to support a one-size-fits-all approach for ICU care. This results in high health care costs from unnecessary ICU admissions and increased risk of in-patient complications like infections. Moreover, resource allocation has become particularly strained due to the COVID pandemic. Taken together, there is a critical need for better predictive and triaging models for stroke patients. The goal of this project is to develop an automated platform to continuously predict the risk of critical care needs for post-MT stroke patients by combining patient history with time series data acquired during the MT procedure. Our project will leverage recent advancements in deep learning along with a large multimodal database of stroke patients curated from the Johns Hopkins Hospital.
Using Motor Imagery and Machine Learning-Based Real-Time fMRI Neurofeedback to Improve Motor Function in Cerebellar Ataxia– Cherie Marvel (Medicine), Archana Venkataraman (ECE) & Linda Rosenthal (Medicine)
Blurb: Cerebellar ataxia is a movement disorder that results from degeneration of the cerebellum. People with cerebellar ataxia experience poor balance (leading to falls), difficulty walking (often needing walker or wheelchair), severe hand tremors, abnormal eye movements, and slurred speech. Current treatments try to counteract symptoms of the disorder via palliative drugs, and physical/speech therapy. While existing treatments can help slow the rate of motor loss, they focus on physical manifestations and do not target the underlying neural mechanisms. This project will use a groundbreaking form of neuroimaging called real-time functional magnetic resonance imaging neurofeedback to train people with cerebellar ataxia to “exercise” their motor network. Using machine learning, we will extract patient-specific neural activations of improved task performance and use these patterns to guide at-home imagery exercises for rehabilitation.