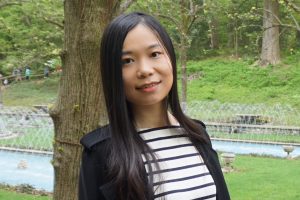
Note: This is a virtual presentation. Here is the link for where the presentation will be taking place.
Title: MRI image analysis with multiple modalities using deep learning methods
Abstract: Magnetic resonance imaging (MRI) has been widely used in scientific and clinical research. It is a non-invasive medical imaging technique to reveal anatomical structures and provides useful information for investigators to explore aging and pathological processes.
Different MR modalities offer different useful properties. T1-weighted (T1-w) MR images have good contrast in the human brain and can be acquired at high resolution. Diffusion weighted imaging (DWI) can quantify the anisotropic diffusion of water in biological tissues. Tagged MRI and cine MRI can be used to measure internal tissue motion.
Automatic MRI analysis algorithms have been developed to address problems in many applications such as classification, segmentation, and disease diagnosis. Segmentation and labeling algorithms applied to brain MRIs enable evaluations of the volumetric changes of specific structures in neurodegenerative diseases. Reconstruction of fiber orientations using diffusion MRI is beneficial to obtain better understanding of the underlying structures.
In recent years, deep learning—especially as implemented by convolutional neural networks—has been widely used for MR image analysis algorithms and achieved superior performance over classic machine learning methods in accuracy and speed. The deep network learns the non-linear mapping from the input to the output automatically based on the training data, providing a powerful way to learn useful representations of the images.
This thesis aims to develop a set of deep learning methods for MRI analysis using
different image modalities. Specifically, we are focusing on MR image segmentation with applications to brain ventricles with ventriculomegaly and the thalamus under mild traumatic brain injury. Some widely-used brain segmentation software packages work on normal anatomical structures and do not generalize well on on subjects with neurodegenerative diseases. We also investigate muscle fiber reconstruction and strain analysis in the human tongue using multiple MRI modalities. The muscle fiber reconstruction algorithm is designed for the tongue and accounts for some special properties of the crossing fibers in the tongue.
The thesis has three specific aims:
- Develop an end-to-end deep learning method for ventricle parcellation of brains with enlarged ventricles using T1-w MRI.
- Develop a deep learning method to automatically parcellate the thalamus into a set of thalamic nuclei using T1-w MRI and DWI.
- Develop a deep learning method to reconstruct the tongue muscle fiber orientations using DWI. Propose a workflow of methods for tongue strain analysis using DWI, tagged MRI, and cine MRI.
Committee Members
- Jerry Prince, Department of Electrical and Computer Engineering
- Archana Venkataraman, Department of Electrical and Computer Engineering
- Vishal M. Patel, Department of Electrical and Computer Engineering