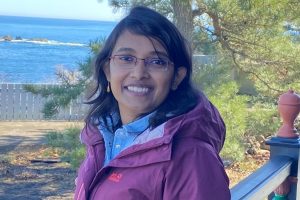
Somdatta Goswami, assistant professor in the Whiting School of Engineering’s Department of Civil and Systems Engineering (CaSE) and member of the Johns Hopkins Data Science and AI Institute, is part of an interdisciplinary team that received a 2024 Johns Hopkins Discovery Award. The team’s project, Data-Driven Deep Learning Architectures for Multiscale Modeling of Biological Systems, or 3D-LAMMBS, aims to develop advanced computational tools to assess lung injuries caused by explosive blast waves and high-impact trauma (known as blast and blunt injuries) for military and civilian populations in war zones.
The Discovery Awards encourage faculty from various disciplines to collaborate in addressing multifaceted challenges. This year, Discovery Awards were given to 44 interdisciplinary faculty teams across 11 units of Johns Hopkins. The award for the 3D-LAMMBS project is one of two projects co-funded by Hopkins’ Data Science and AI Institute.
The 3D-LAMMBS team includes Vicky Nguyen, professor and Marlin U. Zimmerman Jr. Faculty Scholar in the Whiting School’s Department of Mechanical Engineering, and Michael Lapera and Melissa Yates from the Johns Hopkins Applied Physics Laboratory.
“To create computational tools for immediate lung injury assessment, we face a few significant challenges. We must understand biological structures’ behavior at different scales, along with the damage mechanisms, achieve high temporal resolution to compare chronic and acute injuries, and manage increased data flow from war-fighter wearable sensors and environmental feedback,” said Goswami.
To overcome these challenges, the team is using machine learning frameworks that integrate different types of data and then identify correlations among the combined dataset.
Goswami says that “traditional modeling methods used to evaluate and predict risk, like the finite element method, are extremely limited when applied to this type of complex multiscale multiphysics system, but by combining machine learning with the finite element method, we can accelerate real-time injury assessment and enhance predictive risk modeling for sustained injuries.”
The models will be used to determine the need for clinical evaluation and injury risk.
“By understanding injury risk in real-time, medical staff can begin to provide effective treatment more quickly, potentially saving lives and improving outcomes for those who have been injured,” she said.