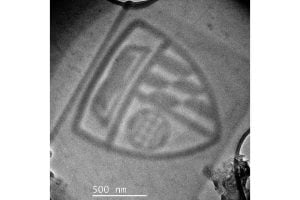
An interdisciplinary team of Johns Hopkins engineers developed an innovative machine learning tool that enables scientists to monitor and assess atomic-scale changes in materials as they happen: a breakthrough that has the potential to accelerate the discovery and development of new materials for a wide variety of applications, including energy storage and electronics. The team’s results appear in Nature Scientific Reports.
“Using this advanced microscope and our innovative technique, we can generate a substantial amount of high-speed data from multiple sensors. Our machine learning tool quickly analyzes the data and matches the microscopic samples with computer simulations and scientific literature,” said team member Jon Hollenbach, a PhD student in the Whiting School of Engineering’s Department of Materials Science and Engineering, who worked with Mitra Taheri, a professor of materials science and engineering and director of its Materials Characterization and Processing (MCP) facility, and graduate students on the project.
“This tool is a breakthrough for us, as this process used to be time-consuming for researchers. Now, we can perform it in real-time, predicting atomic-scale changes as they happen,” he said.
The team developed its approach using a Transmission Electron Microscope (TEM) and applying a method called Electron Energy Loss Spectroscopy (EELS). EELS detects changes in the electric signature produced by the bonding of atoms within the visualized material. The researchers found the combination of the new machine learning tool and advanced microscopy techniques automatically categorized and analyzed data, eliminating what is usually a tedious process.
The tool overcomes a key challenge in this kind of analysis: even the slightest change in a material’s composition and structure (such as losing a fluorine or oxygen atom) can drastically alter how it performs. Previously, scientists faced the challenging task of classifying these microscopic materials’ changes by matching individual experimental data points with their corresponding computationally generated points, resulting in a time-consuming data decoding endeavor.
To simplify this process, the team taught a computer how to organize the data.
“We made the framework for the model and classification training scheme based on sample data of MXenes, a material that can be potentially used for energy storage,” Hollenbach said.
He explains that this rapid classification and processing of data will enable scientists to swiftly refine their experiments and identify the optimal MXenes composition. Traditionally, discovering the best material for energy storage is a slow trial-and-error process.
“By applying machine learning, we can improve how materials for batteries, electronic or magnetic devices, or even coatings for aerospace materials are made by tracking their building blocks in real time,” Taheri said. “We want to work with manufacturing companies and integrate this technology within the microscope itself. We hope that as the TEM scans, this technology can analyze simultaneously,” she said.
The project was supported by multiple agencies and laboratories, including the Department of Energy, Office of Basic Energy Sciences, the Air Force Research Laboratory, the Office of Naval Research, the National Science Foundation, and Pacific Northwest National Laboratory.