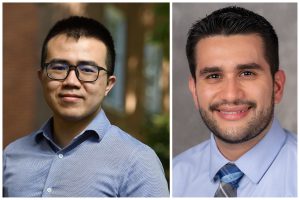
Corey Oses stepped onto the Johns Hopkins campus in August with a four-year postdoc earned at Duke University and an undergraduate degree from Cornell under his belt. His research focus lies in a newer discipline in the field of materials science—one that deals more in the theoretical than the experimental. He uses computational methodology to study high-entropy materials, with a goal of exploring the role they can play in sustainable energy, such as in the recycling of waste heat into electricity and in producing hydrogen. High-entropy materials, which strike that much-needed balance between hard and tough, also are needed for a variety of applications, including hypersonic vehicles.
“Historically, a lot of materials science research has been experimental and not computational. Materials have always been around, but computers haven’t,” Oses explains.
Machine learning and computational software like that used by Oses have allowed researchers to analyze massive data sets and, with a few lines of code, reveal trends. But Oses asserts that computational engineering can enable much more than that. “We run calculations to try to understand the correlation between what we observe macroscopically,” he says, rapping his hand on his desk to demonstrate its material properties, “and what we can calculate microscopically. That’s really the whole goal.”
Using computers to help calculate the mechanical properties of the materials that encompass the built environment was a luxury 20 years ago; now, it is a necessity, Oses believes. He compares it to cooking: trying to understand what ingredients are necessary, how they will interact, and how we process them to get the desired result. (At this level, it is probably more like cooking by way of Wolfgang Puck than what you’re whipping up at home on a busy work night.) Enter AFLOW, a database that Oses helped develop, and which is a massive compendium of more than 3 million material compounds and hundreds of thousands of calculated properties. AFLOW is designed to handle the basic computational work, leaving humans free to play a more creative role.
“Regardless of our computational advances, there will always be need for humans in this equation. Just as there are machines that can make meals in 30 seconds, but you want to go to a restaurant and have food cooked by a good chef,” he says.
He is excited to join the department, with its strong history of collaboration and innovation.
“I’m here to be a part of a strong community of computational work. We can make this department into a powerhouse of materials science and computation,” he says.
While computation promises to enhance our understanding of materials, another new addition to the department is using materials to better understand the human mind. Dingchang Lin arrived at Hopkins this July by way of Stanford, where he received his doctorate in neuroengineering, and a post-doc stint at Harvard. His research focuses on methods of gathering data on the brain—a daunting task considering that organ’s massively complex, nonlinear, three-dimensional nature. Conventional devices for measuring neural activity range from functional MRI technology which can pinpoint only specific sub-brain regions to invasive neural probes inserted directly into the subject’s brain tissue that delineate small areas of the brain. Lin’s team is developing molecular tools and electronics that can map the brain and gather data to understand how it changes over time and under myriad conditions. “With these tools, we will be able to cover the brain’s circuit with higher spatiotemporal resolution by orders of magnitude,” Lin says.
As a post-doc, Lin experimented with methods of recording signals from a number of neurons across the brain that were not possible with conventional technology. He concluded that the only way to achieve this goal is to find a way to temporarily store the neural activity information locally within the cells: a task that has to be approached on a molecular basis, he says. Essentially, this means tagging brain cells so that they can be studied retrospectively, the way tree rings tell us about climate change in centuries past. To this end, Lin’s team developed a tool called the Genetically-Encodable Protein Ticker Tape: an engineered protein that can assemble in cells to record cellular events. Basically, it works as a ticker tape in molecular form that cells can produce themselves to record their own history of neural activity.
“This nanostructure in cells and produced by cells would encode the neural activity information that can be read by imaging like reading a barcode,” Lin says, noting that this information has the capability to further our understanding of degenerative disease, mental illness, and the very nature of knowledge. Additionally, in the process of this research, the team discovered that this protein as some unique properties. “We want to repurpose it for other applications in cell biology,” Lin said “Based on this, we would be able to resolve cell signaling networks in space and time within intact tissue in a live animal. We hope this can be widely used in neuroscience and cell biology to help further the understanding of our fundamental knowledge.”