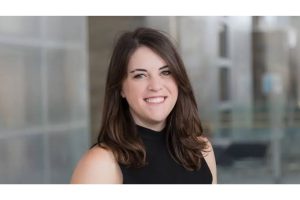
Join the Department of Materials Science and Engineering on April 2nd for a seminar from Rose Cersonsky, who is an assistant professor at the University of Wisconsin-Madison. Her talk, “Chemistry As Data: How We Can Generate Chemical Understanding From Data-Driven Modeling,” will take place in Maryland Hall Room 110 at 3pm.
Abstract: Chemistry as Data: how we can Generate Chemical Understanding from Data-Driven Modeling
In recent years, machine learning (ML) methods have transformed computational chemistry and materials research. In ML algorithms, we rely on machine-learning representations to serve as a “mathematical proxy” for our underlying chemistry. Molecular featurization—how we transform atoms and molecules into mathematical signals appropriate for machine-learning thermodynamic quantities—has an important role in our ability to learn material properties and observable quantities. There are many ways to encode raw chemical data, notably string-based representations such as SMILES or SELFIES, and the suitable choice largely depends on the problem at hand and the architecture of our models. However, in thermodynamic contexts, where the chemistry and connectivity remain largely unchanged, such as in molecular simulation, it is more typical to use configuration-dependent features, which transform molecular coordinates into a range of suitable numerical representations.
In this talk, I will primarily focus on how we assess and interpret models built on such molecular representations, focusing on how to do so using shallow, simple machine-learning models. I’ll first start on the idea of thermodynamic fingerprints as order parameters for complex phenomena, contrasting technologies built through the machine-learning potentials community with traditional analyses, as well as extending these ideas into new methods for bottom-up coarse-graining. From here, I will focus on how to extract actionable chemical and physical principles from models built on chemical data, a task traditionally achieved through unsupervised analyses such as principal components analysis or t-stochastic neighborhood embeddings. However, these methods only ask, “What makes these data points similar?” not “In what ways does my model see these points as similar?” The latter question, particularly in the context of supervised ML models, is more powerful and informative for structure-property relationships. Our results show that this multi-objective framing, with its inherent interpretability, reveals underlying trends across many ML tasks, from materials classification to machine-learning potential building to non-linear regression tasks.
Bio: Rose Cersonsky
Rose K. Cersonsky is the Michael and Virginia Conway Assistant Professor of Chemical and Biological Engineering at the University of Wisconsin-Madison. She received her Bachelor of Science degree in Materials Science and Engineering with a minor concentration in Computer Science from the University of Connecticut in 2014. She went on to obtain her Ph.D. in Macromolecular Science and Engineering from the University of Michigan in 2019 alongside Professor Sharon C. Glotzer, focusing on the self-assembly behavior and optical properties of colloidal nanoparticles. Following her doctoral work, she collaborated with Prof. Michele Ceriotti as a postdoctoral researcher at École Polytechnique Fédérale de Lausanne in Lausanne, Switzerland, working on developing and applying hybrid supervised-unsupervised machine learning models for data-driven studies of molecular design. Rose’s research group at UW-Madison, established in 2023, centers on developing techniques for and using data science and machine learning to unify our understanding of molecular motion and interactions across length scales. She and her group lead the development of scikit-matter, a scikit-learn-affiliated package for quantitative structure-property relations in materials research, and are core developers of chemiscope, an interactive visualizer for data-driven analyses of molecular datasets. Rose’s work has been recognized with a number of awards, including being named one of Matter’s “35 under 35” in Materials Research, the Victor K. LaMer Award from the Colloids Division of the American Chemical Society, the Biointerfaces Institute Innovator Award, and the Charles G. Overberger Award for Excellence in Research.
In addition to research, Rose has devoted herself to scientific service, leading and coordinating multiple outreach programs and publishing work in educational journals on community engagement and gender equity. Recently, she released the commentary “Not yet defect-free: the current landscape for women in computational materials research,” in npj Computational Materials, highlighting the persisting inequities for women in her field.