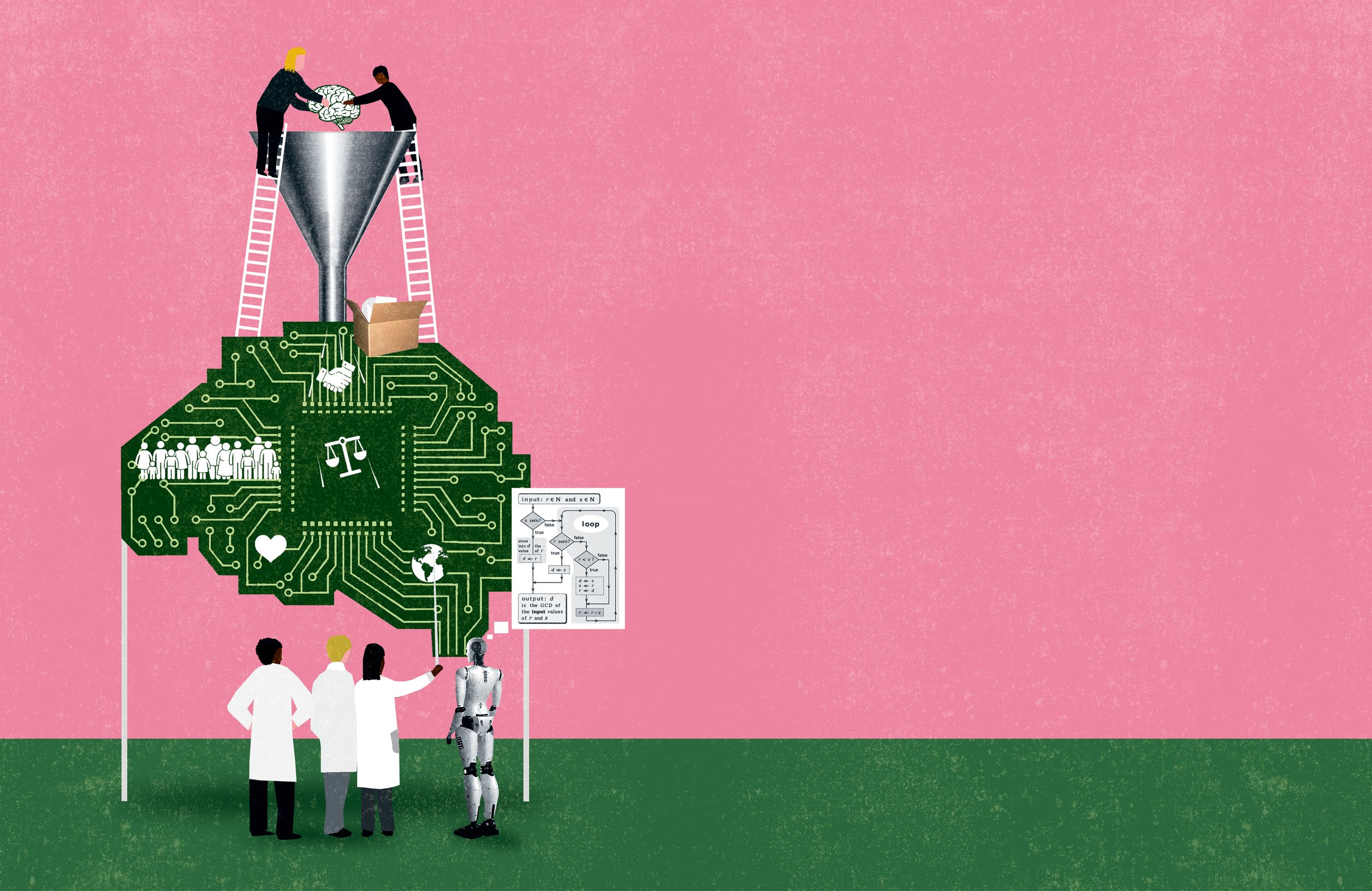
Harnessing advances in data science and AI, Whiting School researchers are working closely with clinicians to improve care for a broad array of debilitating conditions.
When arriving at diagnoses and treatment plans, physicians often must look past an individual patient’s particulars to find the commonalities that are at the root of the disease— in other words, to what makes patients the same. Yet this one-size-fits-all approach can lead to suboptimal care, with drugs and other therapies not working as well as expected for many individuals.
To enhance disease diagnosis and treatment, researchers at the Whiting School of Engineering are instead embracing what makes every patient different. Through advances in data science and AI, reams of patient information can increasingly be gathered by sophisticated yet subtle sensors, both in the clinic and out, and then parsed by algorithms for new insights. In this way, through the development of novel software and hardware, Whiting School researchers are delivering on the promise of precision or personalized medicine—where therapies are tailored to a patient’s unique biology.
Here are five ways that data science and AI capabilities are making precision medicine a reality.
Making Imaging Equitable
To improve outcomes for patients with cancer, Muyinatu “Bisi” Bell figured that proactive detection of the disease in its earliest stages, when it is treatable, was the way to go. She accordingly studied medical imaging, assuming it worked identically for all patients. What Bell quickly learned, though, is that some common imaging methods fail to account for normal human variations in skin tone, tissue type, and tissue density.
“I went into imaging thinking this is one solution that benefits everyone equally, but that is not the case,” says Bell, the John C. Malone Associate Professor of Electrical and Computer Engineering, Biomedical Engineering, and Computer Science.
Take ultrasound and photoacoustic imaging, for example. Both these imaging modalities visualize internal anatomy via the detection of sound waves by sensors in contact with the skin. Ultrasound directly bounces sound off internal tissues, while the photoacoustic method shines laser pulses through the skin, and as bodily tissues absorb this laser energy, they subtly expand and generate ultrasound waves.
![]()
“I went into imaging thinking this is one solution that benefits everyone equally, but that is not the case.” —Muyinatu “Bisi” Bell
For both methods, the timing of the receipt of sound wave signals paints a picture of anatomical locations and physiological features, courtesy of data-processing algorithms. Traditionally, these algorithms are premised on a uniform skin tone and standard ratios of tissue components, such as fat to muscle. Yet the skin of darker-complected individuals absorbs greater amounts of light than that of paler individuals, which scrambles photoacoustic imaging results. Likewise, sound moves differently through the tissue layers of patients with varying fat-to-muscle ratios or different densities of breast tissue. The upshot: Pathological features, such as tumors, can be significantly harder to discern and characterize in Black and brown patients, as well as patients with high or low body mass indexes (BMIs) and tissue densities.
Bell is a pioneer in using data science and AI to remove these biases. She devised a new algorithm rooted in the physics of ultrasound wave propagation that instructs sensors to “talk to their neighbors,” she says. The sensors compare signal receipt timing and cross-correlate data instead of just lumping signals together based on some impersonalized standard. In this way, Bell’s medical imaging algorithm not only constructs a more accurate representation of patients’ internal anatomy, regardless of skin tone, BMI, or tissue density, but also offers greater imaging precision for all patients.
One hitch the new algorithm presents is that its complexity requires additional computing resources. As a solution, Bell has leveraged AI to efficiently speed up processing time by training the algorithm on a wide variety of patient data. “For more individualized imaging that is sharper, clearer, accurate, and faithful to ultrasound physics, these more complex algorithms benefit from AI,” she says.
Overall, the AI-honed algorithm is inexpensive to deploy and still makes imaging results available in real time—both critical elements from a health care delivery perspective, enabling clinicians in rural and remote areas worldwide to immediately inform patients and arrange appropriate follow-up care.
With continuing development, Bell and her colleagues expect to see imaging systems incorporate their approach in coming years. “We’ve shown that it is possible to make imaging technology equitable,” Bell says.
A Better Way in Epilepsy
Epilepsy, or seizure disorder, ranks among the most common neurological diseases, impacting upward of 60 million people globally. Nearly one in three patients does not respond to drugs, leaving brain surgery as the only mainstream treatment to suppress their seizures. The high-stakes surgery aims to cut out the seizure source, known as the epileptogenic zone (EZ)—a localized area of neural tissue where overactive neurons trigger cascading electrical storms. Yet about half of surgery patients still do not find relief and end up suffering again from seizures just six months post-procedure. This high failure rate stems from how notoriously difficult it is to nail down the exact locations and dimensions of EZs.
Enter the aptly named EZTrack (“easy track”), a software tool developed by Sridevi Sarma and colleagues. EZTrack uses AI—alongside some old-fashioned calculus—to map an epilepsy patient’s brain more precisely and uniquely reveal troublesome EZs.
“We’ve found a way to better identify an epileptogenic zone and carefully understand its boundaries,” says Sarma, professor of biomedical engineering and director of the Neuromedical Control Systems Lab. “EZTrack can help clinicians achieve the delicate balance of leaving enough of a patient’s brain alone so as not to impair the individual, but still taking out enough of the brain to stop the seizures.”
“EZTrack can help clinicians achieve the delicate balance of leaving enough of a patient’s brain alone so as not to impair the individual, but still taking out enough of the brain to stop the seizures.” —Sridevi Sarma
EZTrack capitalizes on voluminous, though presently discarded, data gathered during traditional searches for EZs. For patients with elusive EZs, physicians implant violin-string-like electrical wires, called intracranial electroencephalogram (EEG) sensors, directly into the brain. Patients are then monitored for days to weeks in a hospital setting, where the sensors can trace any bouts of seizure to a distinct brain region.
EZTrack takes a contrary approach by also parsing the massive data haul collected when patients are not seizing. With this information, EZTrack builds a dynamical model of a given patient’s brain. That model comprises an interconnected network of nodes, or groups, of neurons. Sarma and colleagues have reasoned that when patients are not experiencing seizures, any fragile, seizure-prone nodes are kept in check by the normal-functioning nodes. With this virtual brain model in hand, EZTrack can simulate stimulation to various nodes, thus zeroing in on fragile nodes as likely EZ candidates. “You can see where a little perturbation caused a huge reaction that never died down, creating a seizure,” says Sarma.
Across retrospective studies involving dozens of patients, EZTrack correctly predicted surgical success about 75% of the time—some 25% better than standard methods. Critically, EZTrack can predict surgical failure with near-100% accuracy, meaning the tool could help clinicians and patients avoid procedures that pointlessly remove healthy brain tissue.
An additional advantage of EZTrack, Sarma notes, is that it does not pose any “black-box” problems for patients or clinicians. In AI circles, a black box refers to a program whose inner workings are not readily interpretable by users, or even by the program’s developers (read more on p. 23). The issue comes up frequently when AI programs employ machine learning across training sets of data to arrive at efficient, yet inscrutable means of achieving high accuracy. But because EZTrack does not rely on machine learning and is instead a patient-specific computational approach rooted in upper-level math, Sarma says “we can know exactly what the AI is doing.”
Having secured a patent for EZTrack along with Food and Drug Administration clearance, the researchers are now working on commercializing the technology. Their AI-powered, brain network mapping could also advance understanding of a range of other conditions including autism, major depressive disorder, and frontal temporal dementia, using data collected by noninvasive EEG sensors placed right on the scalp.
Aging Well
While treating older patients at a Veteran Affairs medical center, Peter Abadir realized he had found his calling in clinical geriatrics. “You fall in love with the stories these patients bring, their camaraderie,” says Abadir, an associate professor of medicine at Johns Hopkins School of Medicine. “I’m drawn to their history and getting to know the real person behind a name in a chart.”
Abadir came to Johns Hopkins in 2007 because it’s one of the very few centers in the U.S. that supports both clinical practice and research in geriatrics. In recent years, this intertwining has reached a new level via the Johns Hopkins–based Human Aging Project (HAP). The transdisciplinary initiative is looking toward engineering and biological solutions to lengthen the time that cognitive or functional decline can be kept at bay for older adults.
One of HAP’s key pillars is the Artificial Intelligence & Technology Collaboratory for Aging Research (AITC), which Abadir serves as co-principal investigator. Started in November 2021 with $20 million in funding from the National Institute on Aging, the goal of the AITC at Johns Hopkins—along with collaboratories at other hosting institutions worldwide—is to engineer novel tech solutions that boost independent living and reduce physical and cognitive frailty, improving lives of older adults and their caretakers.
Abadir is accordingly helping pool the deep resources on the clinical side of Johns Hopkins with those on the engineering side at the Whiting School. “It’s very rare to find such a large number of geriatricians that you can match with engineers,” says Abadir. “That’s the blessing of being in an ecosystem that has expertise in aging and also engineering.”
The day-to-day work of AITC involves funding technology development pilot projects. One core AITC area is Alzheimer’s disease and related dementias. An example project in this space is the use of sensing devices in homes and assisted living facilities that can nonintrusively monitor patient activity. That data can power intelligent risk prediction models for common adverse events, such as urinary tract infections and falls, alerting caregivers so they can render earlier, more effective interventions. A second AITC core area is healthy aging. An example project there involves wearable devices to gather mobility, balance, and gait data to screen for patients’ physical functioning far more comprehensively than time-limited human physicians could ever do.
“It’s very rare to find such a large number of geriatricians that you can match with engineers. That’s the blessing of being in an ecosystem that has expertise in aging and also engineering.” —Peter Abadir
Overall, Abadir sees “tremendous promise” for AI and data science in geriatrics. Reflecting this potential, the Johns Hopkins University School of Medicine and the Whiting School are planning to jointly open a new 10,000-square-foot hub at the site of a former gymnasium on the Bayview campus in fall 2024, where AITC-affiliated engineers can more readily rub shoulders with clinicians and other HAP groups.
Najim Dehak, an associate professor of electrical and computer engineering and the 2021 Whiting School of Engineering/HAP Scholar, is eager to see the fruits of this synergy with Abadir and colleagues. “The new space offers an exciting opportunity for our engineers to work together with clinicians, nurses, older adults, and their caregivers to come up with technology-driven solutions to some of the biggest challenges older people face, such as social isolation or mobility issues,” Dehak says.
Abadir likewise looks forward to seeing the broader endeavor continue to make rapid progress. “AI and other fields are moving at a speed that is unprecedented,” says Abadir, “and while that comes with challenges and ethical concerns we’ll need to iron out, I think the future will be very different when we’ll be using these technologies to enhance our care for older adults.”
“The new space offers an exciting opportunity for our engineers to work together with clinicians, nurses, older adults, and their caregivers to come up with technology-driven solutions to some of the biggest challenges older people face.” —Najim Dehak
Deciphering Neurodegenerative Diseases
The emphasis on innovation in geriatric care at Johns Hopkins reflects the skyrocketing rise of this patient demographic. By 2050, the number of people over the age of 60 is forecasted to jump 70% in developed countries—a figure that pales in comparison to an expected quadrupling in less developed regions.
Amid the accompanying spike in diseases of aging, arguably the hardest to adequately treat will be neurodegenerative conditions, which rob patients of their cognitive and physical abilities. “We’re likely looking at an epidemic of neurodegenerative diseases,” says Laureano Moro-Velazquez, an assistant research professor at the Center for Language and Speech Processing. “It’s a tough problem to solve and we need new tools.”
To this end, Moro-Velazquez is harnessing AI techniques to assist doctors in more efficiently and effectively diagnosing these devastating diseases. Even though Alzheimer’s and Parkinson’s impact distinctly different neural circuits in the brain, patients can experience similar early symptoms, including trouble communicating, walking, and sleeping. Such overlap complicates precise diagnosis, meaning patients might not get medications matched to their actual pathology, especially in the case of Parkinsonian syndromes.
Moro-Velazquez’s work has initially focused on AI-driven analyses of patient speech and language. The models he is developing parse the complexity of a patient’s vocabulary and even track pauses— details that can help in disambiguating the underlying neurodegenerative processes. “This level of analysis would take a human hours and hours,” says Moro-Velazquez. “With our system, it can take seconds.”
“We’re likely looking at an epidemic of neurodegenerative diseases. It’s a tough problem to solve and we need new tools.” —Laureano Moro-Velazquez
In this effort, Moro-Velazquez is working closely with Dehak, and together, the researchers are bringing additional modalities to bear that capture more of the patient’s total physical presentation. Besides microphones for speech, high-speed cameras track eye movements as patients read, and other sensors track how they move their hands while writing, and their gait when ambulating.
“We track the whole body, not just speech production,” says Dehak. “It’s a unique approach that we think can better differentiate one disease from another.”
Logging all this information quantitatively further allows for objective comparisons of how patients are doing at follow-up visits even years later—in marked contrast to current practices, where physicians typically consult subjective notes from past patient encounters. Data for AI-enhanced evaluations could also be collected wherever the right hardware is available, better enabling continuity of care should patients [be treated] at different locations, or even conveniently right in their own homes.
Ultimately, the researchers hope their platform will be able to identify telltale biomarkers for neurodegenerative diseases and serve as a protocol for evaluation. “The way you sound, the way you speak, the way you move your eyes—neurodegeneration can affect these things in distinct ways that our models can distinguish, helping doctors establish a diagnosis,” says Moro-Velazquez.
Genetic On/Off Switch
Beyond screening and diagnosis, data science and AI may also revolutionize the treatment of disease at the genetic level. The research of Michael Beer and colleagues gets to the heart of the matter by investigating enhancers—short regions of DNA that bind certain proteins needed for expressing genes. In this way, enhancers essentially function as genetic on/off switches, playing critical roles in development and health.
“Every cell has the same genetic instruction set, but enhancers allow cells to perform specific functions by reading pieces of that genome out,” says Beer, a professor of biomedical engineering at the Whiting School of Engineering.
Despite their importance and ubiquity—hundreds of thousands are predicted to exist within the roughly three billion “letters” of the human genome —enhancers are elusive entities. Unlike other genomic features, enhancers do not seemingly have any explicit markers. Making matters worse, the specialized snippets do not necessarily appear next to the genes they regulate.
To combat this challenge of enhancer identification, Beer has been developing machine learning programs over his nearly 20-year career at Johns Hopkins. Continued honing of algorithms and experimental methods has recently led to significant advances. In a paper published in Nature Genetics last summer, Beer and colleagues focused on the brief but intense periods of activity when cells transition from one cell type to another. Such bursts prominently occur during embryonic development for cell fate decisions, when undifferentiated stem cells transition into distinct cell types.
“Every cell has the same genetic instruction set, but enhancers allow cells to perform specific functions by reading pieces of that genome out.” —Michael Beer
In their study, Beer’s group examined the rise of endodermal cells, which go on to specialize further as gut-lining cells and other cellular populations. As a first step, the researchers constructed a network model of gene regulation. They then used a technique based on the gene-editing tool called CRISPR to block the activity of suspected enhancers in stem cells cultured in the lab. Next, the researchers monitored for any effects on subsequent gene expression as the stem cells morphed into endodermal cells.
With a resulting wealth of data in hand, the researchers modeled the physical state of the DNA to look for anything that might better reveal furtive enhancers. One big takeaway: Even though enhancers can lie distantly upstream or downstream in DNA from genes they act upon, physical looping of DNA back in on itself usually places enhancers and genetic correspondents in close proximity. “We saw that enhancers were clustered inside DNA loops,” says Beer. Detecting and digging down into these loops could therefore prove fruitful in enhancer discovery.
A second, even bigger takeaway: Many enhancers strongly influence gene expression only during the brief transition phase, but not afterwards during the bulk of a cell’s life. This finding helps solve a key mystery about how previous genome-wide studies have highlighted thousands of disease-associated enhancers that, when tested, show perplexingly little effect on gene expression. “Our results explain a lot about why enhancers are so hard to study,” says Beer. “Their effect is only at the right time.”
Thanks to Beer’s computational approach, geneticists can hope to track down which enhancers potently contribute to disease, and from there pursue enhancer-targeted therapies to control gene expression. Diabetes and cancer are just two of the conditions that could become more tractable if dealt with via fundamental genetics—especially at the personalized granularity of enhancer errors. “I think cancer is a disease of genetic regulation,” says Beer. “Genetic mutations are clearly needed, but what mutations are doing at some level is regulated by the state of the cell. And the state of cell is controlled by enhancers.”