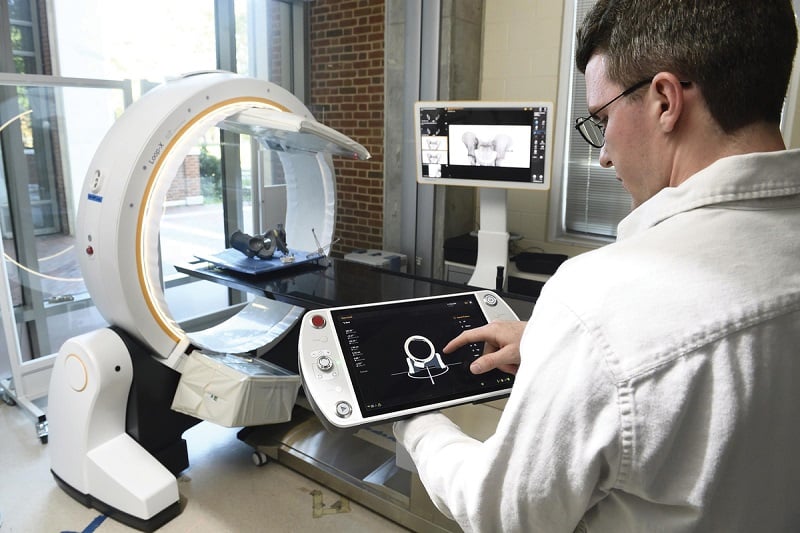
While artificial intelligence continues to transform health care, the tech has an Achilles’ heel: Training AI systems to perform tasks requires annotated data that engineers sometimes just don’t have or cannot get. In a perfect world, researchers would be able to digitally generate the exact data they need when they need it.
“We demonstrated that models trained using only simulated X-rays could be applied to real X-rays from the clinics, without any loss of performance.”— MATHIAS UNBERATH
In reality, however, even digitally generating this data is tricky because real-world data, especially in medicine, is complex and multifaceted. But solutions are in the pipeline. Researchers in the Laboratory for Computational Sensing and Robotics have created software that realistically simulates the data necessary for developing AI algorithms that perform important tasks in surgery, such as X-ray image analysis.
The researchers found that algorithms built with the new system, called SyntheX, performed as well as or even better than those built from real data in multiple applications, including giving a robot the ability to detect surgical instruments during procedures. The results appeared in Nature Machine Intelligence.
“We show that generating realistic synthetic data is a viable resource for developing AI models and much more feasible than collecting real clinical data, which can be incredibly hard to come by or, in some cases, simply doesn’t exist,” says senior author Mathias Unberath, an assistant professor of computer science.
Take X-ray guided surgery, for instance. Say you want to develop a new surgical robot and the related algorithms that will ensure that it puts instruments in the correct places during an X-ray guided procedure. But the training dataset needed—in this case, highly specific X-ray images—doesn’t exist.
The answer? Generate the data needed through simulation, say the researchers. To test this approach, the team performed a first-of-its-kind study in which the team members created the same X-ray image dataset both in reality and in their simulation platform.
First, they took a series of real X-rays and CT scans, acquired from cadavers. Next, they generated “synthetic” X-ray images that precisely recreated the real-world experiment. Both datasets were then used to develop and train new AI algorithms capable of making clinically meaningful predictions on real X-ray images. The algorithm trained on the simulated data performed as well as that trained on real data.
“We demonstrated that models trained using only simulated X-rays could be applied to real X-rays from the clinics, without any loss of performance,” Unberath says.
The system appears to be one of the first to demonstrate that realistic simulation is both convenient and valuable for developing X-ray image analysis models, which paves the way for all sorts of novel algorithms.