In 1849, prospectors began flocking to the California hills in search of golden treasure, digging, panning, and blasting their way through mounds of gravel and silt in search of a few of those elusive shiny flakes that could turn their dreams of riches into a reality. And while often portrayed as dirty, bearded, and somewhat crazed, gold-rush prospectors were in fact frequently skilled engineers, using their knowledge of geology, hydraulics, and metallurgy to build sophisticated machinery to help them separate out the gold from the silt. Even though most came away empty-handed, it did not deter a host of others from pursuing their dreams against such enormous odds. The potential reward was just too great.
[David Audley (left) makes use of principles like feedback and resistance to codify financial networks. The key elements to tease out from this continual back-and-forth are the cycles-whether day-to-day or decade-spanning.]
Today, 160 years later, prospectors of a different sort are converging on a new source of untapped wealth: information. It’s everywhere; vast and interconnected information networks permeate every aspect of today’s society, whether it’s the global network of financial markets, the global network of the World Wide Web, or even the global network of our body’s genes and proteins. And within these networks, well hidden and encoded, lies the gold: patterns. Locating and identifying these patterns could have repercussions ranging from the individual (doctors may be able to determine exactly how a cancer patient will respond to chemotherapy) to the international (security agents could quickly identify suspicious transactions that may have links to terrorism) level.
Of course, finding the needles in these information haystacks is a tremendously difficult endeavor, especially considering that every day our capacity for information expands. But, like the ’49ers of times past, today’s prospectors have developed their own sophisticated techniques to mine this immense data repository. Hailing from engineering, mathematics, and computing disciplines, these Whiting School data miners are initiating a new gold rush. Only this time, everyone can get rich.
The current economic situation may not be well suited for riches. But while most people view the global downturn in the financial markets and institutions with trepidation and anxiety, David Audley, PhD ’72, sees excitement and potential-from an academic perspective at least. “We’ve had a financial Big Bang,” Audley says. “The financial universe as we knew it has exploded, and now we have to figure out what shape the new universe will take.” As executive director of the Whiting School’s recently formed Financial Mathematics Master of Science in Engineering, Audley is one of the people looking into this brave new financial world.
Just don’t ask him which stocks will make a big rebound, though. “Prediction is a fool’s game in finance,” he says. “The forces governing the creation and movement of capital are just too complicated.” And Audley has a perfect perspective on this; after earning his PhD in electrical engineering here in 1972, he spent 16 years in the Air Force as a test pilot, then followed that up with 20 years as a Wall Street analyst and portfolio manager, before returning to his alma mater two years ago to take up his current post.
While both professions had their heartwrenching moments, they differ in one critical regard, Audley says. “In the Air Force, I always knew that the principles of aerodynamics were governed by the laws of physics; tangible and ultimate truths. Then I came to Wall Street and discovered that in finance, there is no truth.” Audley is not implying that everyone on Wall Street is a crook, but instead that the intangibility around global finance stems from the factors that control it government regulatory laws, the ebb and flow of political power, and a host of other continually shifting elements.
Even though Audley deals with a nontangible asset like global capital, he still very much feels like an engineer, or as he says, a practitioner (as opposed to economists who usually deal with the theory of finance). The interconnected world of finance is not much different from an electrical circuit; industries that produce capital act like nodes, with the goods and services produced by these industries traveling back and forth on the circuits between these nodes. And much like in electrical engineering, Audley makes use of principles like feedback and resistance to codify these financial networks.
The key elements to try and tease out from this continual back-and-forth, Audley says, are the cycles-whether they are day-to-day fluctuations in the stock market, or decade-spanning growths and recessions. Identifying the point at which a certain sector of the financial market may be in both its short- and long-term cycle, and where it might be 10 years in the future, can help to minimize the risks in planning a pension portfolio.
Notes Audley, “We have always had modeling concepts to accommodate the extreme and rare behavior of markets and the consequential, but obscure correlations between financial variables. The events we’ve seen over the past couple of years with the ‘credit crisis’ has provided a trove of data so that those concepts can be converted into models that can now be effectively parameterized; thereby providing useful guidance in the future to accommodate the balance of risk and return.”
To that end, Audley does make use of the instant data available from financial networks like Bloomberg, though even as he checks his computer to get the latest Treasury update, he stresses that he tries to limit his news intake: “These financial networks can be a little addictive,” he says. “It makes it hard to get my work done.”
Audley notes his current studies are very similar to his days on Wall Street as an investment manager, primarily focusing on maximizing the risk versus reward ratio, examining all possible scenarios to achieve some gain no matter how the economy turns out. “It’s analogous to driving down a long and bumpy road, knowing a stoplight is coming up in a few miles,” Audley says. “There’s no way to know when we get there whether that light will be red, yellow, or green, but our hope is no matter the color, when we reach the light we will know how to react, so we won’t have to slam on the brakes and spill our coffee.”
The aging Baby Boomers do more than present a financial dilemma for analysts like Audley; they also present a growing health care concern. With a graying population come age related diseases, most notably cancer but also neurodegenerative disorders like Alzheimer’s. Both disease types can be a huge burden to the patients, their families, and hospitals because they require years of continual treatment. To minimize the clinical burden and improve patient survival, it would be ideal to find people at risk for these diseases, diagnose them early, and determine which therapies might work best.
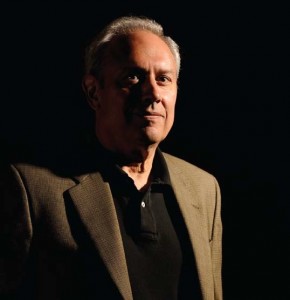
[For mathematician Donald Geman, the key is to identify patterns or trends, potentially involving several thousand genes, which differentiate healthy and diseased tissue, or suggest that patient A wil respond to the latest cancer drug but patient B won’t.]
Mathematicians like Donald Geman know that many of the answers to these problems are encoded in the 3 billion bases that make up our DNA and the circumstances under which pieces of DNA, called genes, are activated. The only hitch is trying to extract that information. The technology to see what’s going on inside our cells is available, in the form of microarrays, or gene chips-small wafers typically not much bigger than a credit card that can contain anywhere from a handful to a million tiny “dots” of DNA, usually corresponding to a specific gene. When any sample, like say an extract of a tumor biopsy, is passed over these chips, the dots light up like a Christmas tree to reveal the relative abundance of those genes. However, it’s never so simple as to find a single target that can determine the presence of cancer; “As in many connected systems, genes operate under complex dependency,” says Geman, a professor in the Department of Applied Mathematics and Statistics and member of Hopkins’ Institute for Computational Medicine. “You turn one gene off, it alters the levels of several other genes, which in turn branch out and affect some more.
“So the key is to identify patterns or trends, potentially involving several genes acting in concert, which differentiate healthy and diseased tissue, or suggest that patient A will respond to the latest cancer drug but patient B won’t. Geman notes that specialized computer programs called “machine learning algorithms” have been adapted from other fields like speech recognition (i.e., computers that can speak back what you type because they’ve learned the sounds made by specific letter combinations) to uncover these genetic patterns. The programs scan through samples where the outcome is known, like cancer or no cancer, trying to find a rule that can make subsequent predictions.
Herein lies a rub, however: sample size. While programs for speech analysis can pore over millions of sound bytes to train and identify vocal patterns, computational biologists usually only have tens, occasionally hundreds, of tissue samples available. One may think this would be a good thing-that fewer data would make life easier for these algorithms. But, notes Geman, “when trying to extract information from data sets, more samples is always better. And given the level of complexity within genetic data, we would probably need trillions of samples to learn the interactions among many genes at once. So classical statistics is telling us: Don’t touch this, work on something easier.”
Left: Gene expression values (mRNA counts) are shown for 72 leukemia patients for two of the 7,129 genes on a particular microarray: SPTAN1 in red and CD33 in black. For the first 47 patients, who have acute myeloid leukemia (AML), SPTAN1 is usually expressed more highly than CD33, whereas for the second 25 patients, who have acute lymphoblastic leukemia (ALL), the reverse ordering is usually true. This allows AML and ALL to be well-separated based on a two-gene interaction.]
Geman and others aren’t fazed by such warnings. Their response to this number problem is to have the programs identify a handful of linked genes that really stand out in their expression levels and then to make them representatives of the whole, just as election polls can provide reliable results using only a small subset of the general population. Much like those polls, these gene analyses are highly accurate, but not completely so; that +/- 3% hovers in the background.
At that point the biologists take over, to confirm whether the genes identified by the microarrays and programs are genuine and could be considered disease biomarkers. This validation is necessary not just because of that small percent inaccuracy but also to weed out a second major hurdle in computational biology: overfitting. “It’s like the monkeys at a typewriter,” Geman says. “Researchers are willing to entertain so many possible rules for that limited amount of data that they’re bound to find something by chance.” Unfortunately, this problem may not be overcome by advances in computing because it often arises from having too few samples to learn from. While researchers try and eliminate all bias in their search for genetic biomarkers, the highly competitive biomedical field-and its “We found it first!” mentality-makes it difficult. “In your zeal to find something, you’ll find something,” he says.
Civil engineering professor James Guest is also a fan of the wonderfully complex world of biology, though as a civil engineer, he gravitates toward the amazing structures found in nature. Take bone, for example. At the surface, bone appears ordinary enough: smooth, sturdy, and white; but farther down, at a microscopic level, bone is not simply a solid mass, but a dense and richly layered honeycomb that gives it a fair degree of flexibility (as those of us who have played with wishbones at Thanksgiving can attest to) and porosity. “The solid material and the gaps are interspersed in just the right places,” Guest says, “making bone perfectly designed for its biological purpose.”
Achieving that same high standard-optimal design-is what Guest hopes to do with other structures. Emerging in the 1990s as a progression from the field of structural design, structural topology optimization seeks the ideal design for structures-whether entire buildings and vehicles or the individual materials used in construction-for any given parameters.
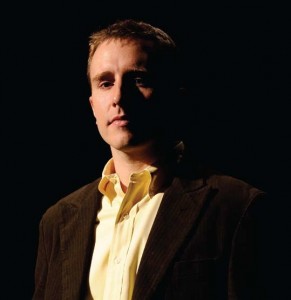
[Jamie Guest’s (left) interests lie in optimizing micro-scale structures-necessary for developing biomedical structures, like tissue scaffolds or integrated drug delivery devices.]
The field’s main applications lie in mass-produced items, where optimization can reduce the materials, cost, and construction time needed. Though the field is still in its early stages (current applicability is limited to straightforward structures like trusses), Guest and other optimizers are hoping to improve their programs for more complicated systems, whether mundane (optimizing the strength and springiness of micro-grippers) or exotic (optimizing the weight and thermal conductivity of spacecraft heat shields).
Guest’s particular interests lie in optimizing micro-scale structures, like the stacked layers of bone tissue. He believes improving microdesigns will be necessary to develop effective biomedical structures, like tissue scaffolds or integrated drug delivery devices-an area that both he and Hopkins are keenly interested in.
For a simpler example, Guest notes a product commonly used in many areas like medicine, industry, and even domestics: filters. To the eye, most filters appear like plain paper or other simple material, but under a microscope, they’re a complex meshwork of fibers that enables liquid to separate from particulates. Topology optimization – which happened to be Guest’s dissertation work – would attempt to identify the mesh pattern that can maximize liquid flow while retaining enough strength to withstand the pressure of the flow. Even a simple filter, like a coffee filter for example, can arrange itself in nearly limitless conformations that provide various levels of strength and permeability. How to find the one golden arrangement? Unfortunately, unlike evolution, Guest does not have hundreds of millions of years to work with. So powerful computing technology will have to suffice.
“Conceptually, it’s not too difficult,” Guest says, bringing over his laptop computer and indicating a green oval on the screen. “Imagine this as our starting structure; now, I’m going to apply two forces, pulling on the left and right side, like you were trying to rip it in half.” Guest then presses a key, and instantly patches of yellow, red, and blue appear at the oval edges, gradually moving inward toward the center. Essentially, the optimization program proceeds from point to point, analyzing what should occupy that spot for the best force response-red equals material, blue equals empty, and green equals don’t know. Eventually, the forces reach the center, completing an elegant snowflake-like structure. Depending on how much force is applied and at which locations, the optimal design can change, and in a few moments, Guest has produced a virtual kaleidoscope of different designs.
Left: These images provided by Guest depict the design of engineered materials with optimized properties. The algorithm begins with a non-discrete topology (left) and converges to a clear microstructure that yields an optimal combination of stiffness and fluid flow properties (middle). This pattern is repeated to form the bulk material (right).
As Guest proceeds to unveil a few more of his models, for example a cube filled with protrusions and crevices to the point it resembles a well-played Jenga tower, one might wonder whether Guest is really a civil engineer or in fact an aspiring artist. “What I most enjoy about structural optimization is that every design starts as a blank canvas; once I set up the optimization program I can sit back and watch the structure unfold,” he says.
Of course, in the real world, finding the ideal micro-scale design for systems like synthetic bone or ligaments, which are influenced by several competing properties, is near impossible; so like Geman, Guest makes some concessions. Potential structural designs map out much like an egg carton, filled with peaks (undesirable) and valleys (desirable), while theoretically there is one valley deeper than all the rest (a global minimum). Guest notes the best hope is uncovering a near-optimal local minimum. “After I find an initial design, I tweak some parameters and run the program again, and see if it improves at all; I keep doing that until I can’t say, ‘I’ve done better than before’ anymore.” Guest also notes that structural design considers non-quantifiable constraints as well, like cost or aesthetics, so the most optimal design may not be the best-suited anyway.
When Guest has refined his optimization programs a little more, computer science professor Andreas Terzis might be one of the many clients eager to step up. For the past several years Terzis, along with Alex Szalay in Physics and Astronomy at the School of Arts and Sciences (who helped develop the Sloan Digital Sky Survey, an enormous astronomical database), has been working on a set of devices that could certainly benefit from some optimization: wireless sensor networks.
[“Each piece of data by itself is not spectacular,” says Andreas Terzis (left), “but now, (with sensor net technology), we can put all the pieces together from different parts of a forest and begin to see trends emerge.”]
Portable battery-powered devices not much bigger than a cell phone (and getting smaller every year), motes are tiny computers that can be fitted with a variety of sensory devices, such as thermometers, pH meters, or humidity sensors, to transmit real-time sensory information from multiple locations simultaneously. Sensor nets, which are collections of mote devices, were originally developed around a decade ago for military applications (as many advances seem to be) but have now reached a point where scientists can begin to use them for largescale applications
One area where sensor nets can make a significant impact is in environmental monitoring, as their small, wireless design enables them to collect synchronous data in places people cannot easily do so (forests, mountains, etc.). “Each piece of data by itself is not spectacular,” Terzis says, “but now we can put all the pieces together from different parts of a forest and begin to see trends emerge that we didn’t know about.” Terzis and Szalay, for example, have teamed up with Katalin Szlavecz in Earth and Planetary Sciences at the School of Arts and Sciences to examine the contribution of soil to carbon dioxide flux. “On land, soil acts as both a major pool and source of carbon dioxide,” Terzis says. “But there is growing concern that with global warming, the processes that generate carbon dioxide in soil are accelerating; so we’re going to place these sensor nets in the ground and study long-term carbon dioxide flux.”
Sensor nets can be just as valuable in urban settings; they can greatly improve patient monitoring in hospitals, allowing staff to keep track of patients at all times, and city planners can use them to monitor traffic patterns throughout the day to identify congestion hot-spots. And in a perhaps fitting twist, these sensor nets are even finding a home in the information technology arena world.
“As a whole, data centers consume enough power for 5.8 million average households (as of 2006), and this figure is expected to double in five years,” Terzis says. Only half of this energy usage comes from the actual computers storing all our valuable data, however; the rest comes from the air conditioning required to prevent the servers in the large data centers at companies like Microsoft and Google from overheating. “These companies don’t often know exactly how much cooling they need,” Terzis says, “so they’re often being liberal and overcooling, which wastes energy.” By placing sensor nets throughout these data centers, the IT people can set up real-time 3-D heat maps, allowing them to optimize server cooling and identify possible heating concerns immediately.
The potential uses for sensor nets, in fact, are so numerous that they do raise a significant obstacle, one that researchers in many of these data mining fields have had to tackle. “This technology is being used in previously untapped areas, meaning there are no standard programs or formulas that we can use,” Szalay says. “So the emerging challenge for us is inventing the next generation of algorithms for this wide array of new applications.”
Such challenges do not faze a prospector like Terzis; he relishes them. As a graduate student at UCLA, Terzis had worked on developing Internet technology, but as this tool was shifting from a research program to a commercial product, he found his options becoming limited. “And that’s what drew me into this field, because wireless sensor networks are ready to explode, I think,” he says. He adds a thought likely on the minds of all the data miners: “As a scientist, you like to go in an area where things are happening fast.”