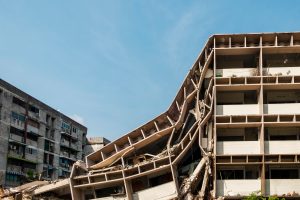
In collaboration with researchers at Idaho National Laboratory, a team including lead researcher and civil and systems engineering doctoral student Promit Chakroborty has devised a faster and more cost-effective way to estimate the likelihood of failure in large, complex, real-world systems: a crucial aspect of ensuring the safety of various infrastructure systems, such as nuclear reactors, electrical networks, as well as bridges, high-rises and other large-scale structures. The team’s results appear in the American Society of Civil Engineers’ Journal of Engineering Mechanics.
The Challenge
The researchers’ goal was to enhance the accuracy of failure probability estimates through more efficient computational methods. Obtaining precise estimates is often a resource-intensive process, involving significant computation time, experimental costs, and lengthy system redesigns.
“In real-life systems, we grapple with inherent randomness and imperfect information when trying to predict system reliability and potential system failures. Estimating failure is critical for ensuring the safety of engineering systems, but the associated computational time and costs are often prohibitive,” said Chakroborty, who is advised by Michael Shields, associate professor in the Whiting School of Engineering’s Department of Civil and Systems Engineering and principal investigator on the project.
The Method
The researchers approached the problem using multi-fidelity modeling, which utilizes information from different models of the same system. They then used a machine learning technique known as active learning to combine the models, allowing them to incorporate new information without restarting the overall process of estimating system failure.
Chakroborty said the active learning approach was crucial because, without it, multi-fidelity modeling alone can result in less accurate response prediction, depending on the models used. He also noted that most machine learning techniques create models that do not adhere to physical laws but that by combining both multi-fidelity modeling and active learning, the researchers theorized that they could create models that predict the system response with high accuracy while also providing insights about the physics at play.
“Our ultimate goal was to correct less accurate models and select the optimal model for each set of input parameters, thereby achieving highly accurate failure probabilities for specific systems while drastically reducing time and cost,” he said.
Key Findings
The team members say that their research demonstrates that combining multi-fidelity modeling with active learning is a viable strategy to improve the overall efficiency of failure probability estimation. They say this approach not only provides accurate predictions of system responses but also offers insights into the underlying physics governing these systems to prevent future catastrophic failures of infrastructure systems.
“Ultimately, the integration of machine learning techniques with traditional mathematical models proved to be a powerful tool in achieving both accuracy and interpretability, shortening the modeling and estimation process from weeks to just days or hours,” Chakroborty said.
What’s Next?
Chakroborty plans to continue to work on the problems of efficiency and robustness related to failure probability estimates and the team is working on a new framework for applying multi-fidelity modeling to estimate failure probabilities. Chakroborty explains that “while there’s more work to be done on improving the interpretability of machine learning models, combining active learning with physics-based mathematical models is a promising avenue of research to improve our physical environment and reinforce infrastructure systems essential for modern society.”
Project Funding
This work has been supported by the Lab Directed Research and Development Program under Department of Energy Idaho Operations Office Contract DE-AC07-05ID14517 with Som Dhulipala at Idaho National Laboratory and Shields as PI at Johns Hopkins University Whiting School of Engineering.