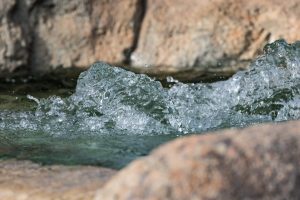
A Johns Hopkins civil engineer was part of a team that has developed a new computer model that can accurately predict how pumping water from the ground affects underground water levels.
“For the first time, artificial intelligence-based approaches have been successfully applied to address the complexities of simulating groundwater, which sheds light on the potential benefits of developing efficient emulators to predict subsurface flows,” said Somdatta Goswami, assistant professor at the Whiting School of Engineering’s Department of Civil and Systems Engineering, whose team developed the new approach while she was a research assistant professor at Brown University.
Their results appear in The Journal of Hydrology.
Groundwater supplies nearly 50% of people around the world with drinking water and plays a pivotal role in agricultural production. Groundwater models can estimate flows and simulate their response to hypothetical conditions. However, efficiently modeling and predicting groundwater flow remains challenging due to heavy computational demands, especially for large-scale systems with multiple varying parameters like location, geography, and climate change.
To address these limitations, Goswami’s team turned to state-of-the-art deep learning models and used a relatively new approach to operator modeling, known as the deep operator network or DeepONet.
“DeepONet is an efficient operator learning model that was trained using multiple, concurrent operating conditions (different locations of aquifers, soil parameters, etc.). By using a simple and flexible architecture consisting of two deep neural networks, DeepONet was able to accurately predict the desired quantity of interest, such as fluid pressure, at any location within a specified region for a wide range of unseen test conditions,” Goswami said.
“In addition to its accuracy, DeepONet is also highly efficient compared to traditional mathematical groundwater modeling. By incorporating deep learning, models no longer need to be re-calibrated each time new data is introduced,” said Goswami. Currently, DeepONet requires a few hours to be sufficiently trained, but once it is trained, the model can be used to predict the desired quantity of interest for unseen conditions in approximately 10 milliseconds, which is many times faster than using existing mathematical software. With its remarkable speed, DeepONet can also provide valuable real-time inference in emergency situations.
To test their model, the team generated datasets that mimic the hydrologic model of the United States Geological Survey (USGS), the international standard for simulating and predicting groundwater conditions. The team verified their model by showing it could accurately predict both well locations based on given pressure field and pressure field based on variables such as soil permeability and well location.
The study’s code and resources are accessible on GitHub.
“In the long term, this type of model will facilitate more informed decision-making in water resource management, and also extend to accommodate more complicated and realistic subsurface problems,” said Goswami.