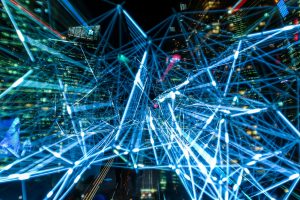
The Data Science Master’s program at the Johns Hopkins University is a fully residential program which will provide the training in applied mathematics, statistics and computer science to serve as the basis for an understanding, and appreciation, of existing data science tools. Our program aims to produce the next generation of leaders in data science by emphasizing mastery of the skills needed to translate real-world data-driven problems in mathematical ones, and then solving these problems by using a diverse collection of scientific tools.
The final Capstone Experience in Data Science (EN.553.806) is a research-oriented project which must be approved by the research supervisor, academic advisor and the Internal Oversight Committee. The Capstone Experience can be taken in multiple semesters, but the total number of credits required for successful completion is six (6). The goal of the final course and written paper is to allow the student to apply data analysis techniques learned in the program, and possibly to extend those ideas to more general settings or to new application areas. Lastly, the paper will be summarized in a poster session organized at the end of each semester.
Your Path to Success: Inside JHU’s Master’s in Data Science Program
Other Data Science Program Links: