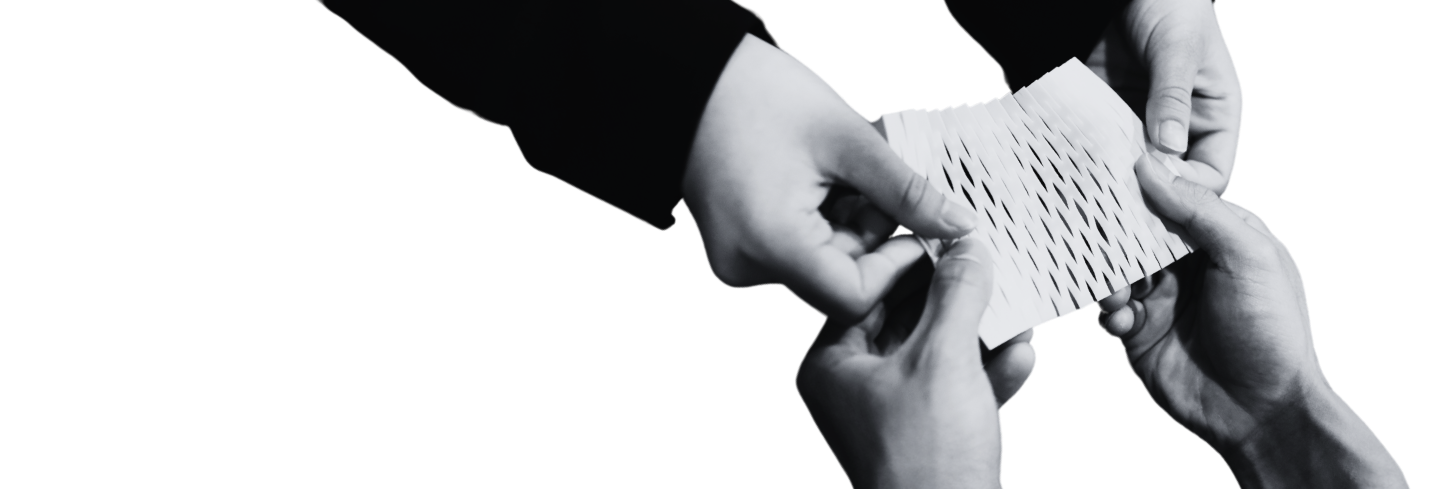
Create
Design Project Gallery
Decoding Physiological Waveforms for Early Prediction of Sepsis
- Program: Biomedical Engineering
- Course: EN.580.480 Precision Care Medicine
- Year: 2025
Project Description:
Sepsis is a life-threatening condition characterized by a dysregulated host response to infection. Early prediction is crucial for improving outcomes but remains a challenge using traditional models. Leveraging data from 11,325 adult ICU patients in the MIMIC-III dataset, we developed a transformer-based model to predict sepsis onset and outcomes using clinical features and high-frequency physiological waveforms (e.g., ECG, PPG, ABP). Clinical and waveform data were tokenized into learnable embeddings, enabling the model to capture temporal dynamics through cross-attention mechanisms. Performance evaluation showed strong predictive ability at shorter lead times, with reduced accuracy at longer horizons, highlighting the need for richer input features. To address this, we built a waveform tokenization pipeline to distill informative segments from raw signals. Token importance analysis revealed distinct physiological and clinical markers associated with sepsis. Future work will focus on fusing clinical and waveform embeddings to improve accuracy and interpretability for early, actionable sepsis prediction in critical care.