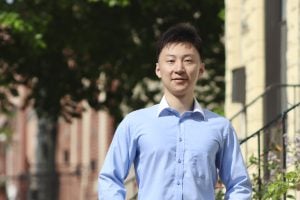
An applied mathematics and statistics major in the Whiting School of Engineering used mathematical modeling to address a critical challenge in the field of sustainable energy: making clean, reliable, and affordable energy accessible to people in remote or underdeveloped areas.
Raymond Gong found that with the right policy incentives, localized energy networks known as hybrid microgrids can produce fewer carbon emissions, use more renewable energy, and cost less overall. For communities that lack reliable power, his work offers a data-backed strategy to help these underserved regions invest in cleaner, more reliable power systems.
“Smart policies can really make a difference in shifting toward cleaner energy,” Gong said. “You don’t have to give up reliability or affordability to go green.”
He will present his project on April 29 at the Whiting School of Engineering’s Design Day, an annual event showcasing students’ solutions to real-world challenges.
To identify the most cost-effective and sustainable microgrid setups, Gong first developed a simulation-based model that tested different combinations of energy sources and policy incentives. His goal was to find configurations that balanced affordability, reliability, and clean energy use.
His simulations revealed that with well-designed policy incentives, microgrids could significantly increase their reliance on renewables while lowering overall system costs, without sacrificing performance and reliability. Systems that paired moderate carbon reduction incentives (like a small tax on fossil fuels) with strong renewable energy subsidies (such as rebates for installing solar panels) consistently outperformed others in both cost and emissions.
To support those findings, he ran two advanced simulation models focused on solar and wind power: one predicted production using historical weather data, accounting for the ups and downs of natural variability, while the second used Markov Chain Monte Carlo (MCMC) to model the performance of key microgrid components–such as solar panels and wind turbines–perform over time, factoring in wear, failure risk, and maintenance needs.
“Microgrids have to handle a lot of uncertainty,” he said. “Weather changes, machines break—it all adds up. I wanted the model to reflect that.”
Next, Gong developed a tool that calculates total expenses, which considers a variety of financial factors, including the upfront investment needed to build and install a hybrid microgrid system, ongoing operating costs, and the economic impact of power outages and carbon emissions. He also factored in two key policy levers: incentives for using renewable energy and for reducing carbon emissions.
With that foundation, he applied Mixed-Variable Simultaneous Perturbation Stochastic Algorithm (MSPSA), an optimization technique designed for complex, unpredictable systems). He compared its performance to Particle Swarm Optimization (PSO), a method used in energy planning to find the most efficient solutions by mimicking the collective behavior of birds or fish.
“What surprised me was how much better MSPSA performed compared to PSO—not just in speed, but also in its ability to identify cleaner and more cost-effective solutions,” he said. “PSO isn’t really built for noisy or unpredictable systems like those in renewable energy planning, yet it’s still widely used in academic studies without much scrutiny. MSPSA, on the other hand, is specifically designed to handle randomness, which likely explains why it performed so much better in this context.”