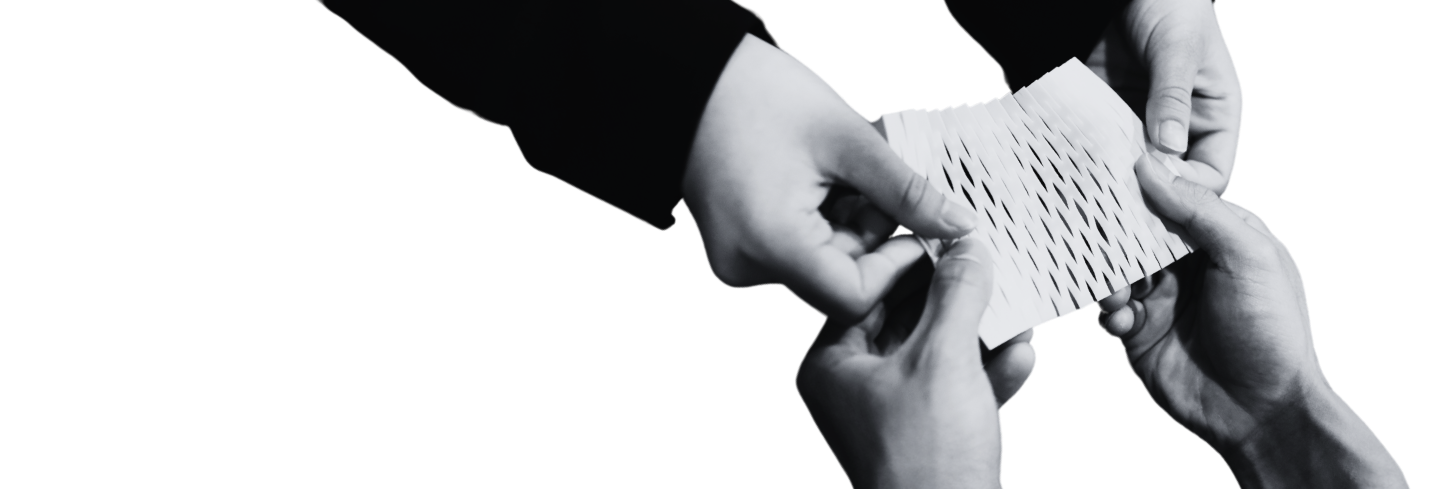
Create
Design Project Gallery
Developing Machine Learning Models to Predict for Pediatric Mechanical Ventilation-Associated Events (PedVAE) in Patients
- Program: Biomedical Engineering
- Course: EN.580.480 Precision Care Medicine
- Year: 2025
Project Description:
Pediatric ventilator-associated events (PedVAEs) are critical complications in the PICU, linked to increased mortality, prolonged hospital stays, and higher resource use. Yet, existing prediction models are primarily based on adult data, limiting their effectiveness for pediatric care. Our project utilizes high-resolution electronic health records (EHR) and continuous physiological time-series data from over 1,200 ventilated pediatric patients at Johns Hopkins. We developed several machine learning models to enable early PedVAE detection, refining clinical definitions to improve labeling and model performance. The XGBoost model achieved the highest accuracy (77.6%) and precision (76.7%) among the models evaluated. These results demonstrate the potential for pediatric-specific predictive analytics to support early clinical decision-making and improve outcomes for critically ill children.