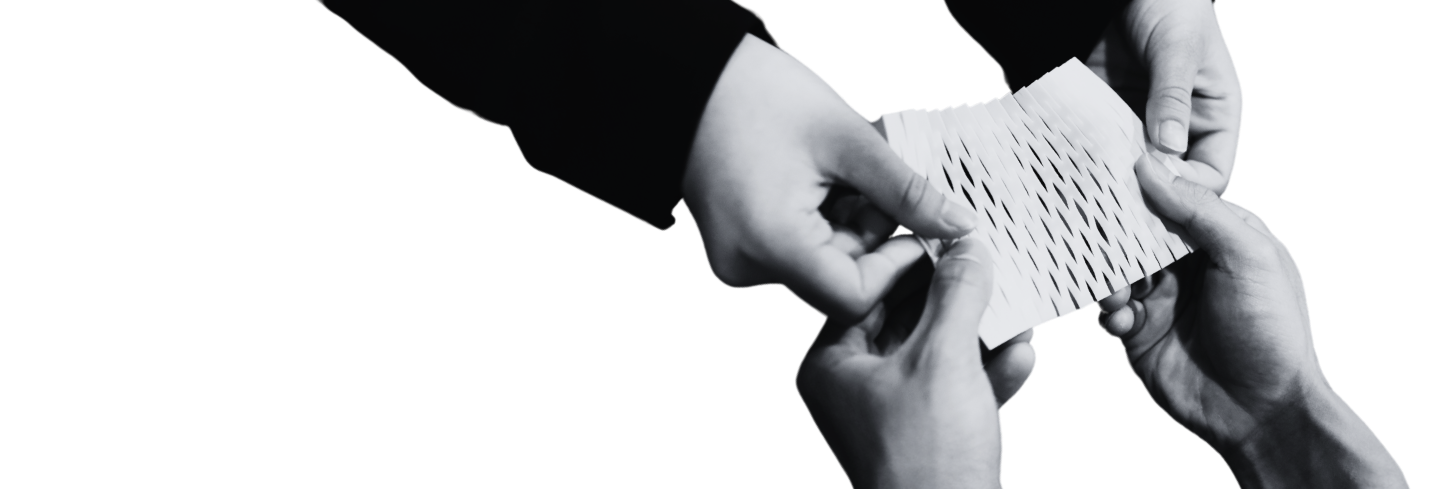
Create
Design Project Gallery
Genomic Subgrouping of ALS Patients to Investigate Differences in Clinical Progression
- Program: Biomedical Engineering
- Course: EN.580.480 Precision Care Medicine
- Year: 2025
Project Description:
Amyotrophic lateral sclerosis (ALS) is a fatal, progressive neurodegenerative disorder marked by significant clinical and genetic heterogeneity, making diagnosis, prognosis, and treatment personalization difficult. Current subgrouping methods often overlook the underlying biological mechanisms driving disease variability.
This project applies unsupervised machine learning to cluster iPSC-derived gene expression data focused on TDP-43 loss of function and identify molecular subgroups of ALS patients from the Answer ALS dataset. The genetic clusters are then analyzed against clinical measurements using linear mixed-effects models to evaluate differences in the way the disease presents itself in a clinical setting across subgroups.
Clustering revealed distinct patient subgroups with statistically significant variations in clinical progression, including differential decline muscle function, and genetic analysis further identified key genes with distinct expression patterns across clusters. These findings demonstrate that ALS patient stratification based on iPSC data can uncover clinically relevant subtypes, allowing for precision diagnostic and subgroup-specific therapeutic development.