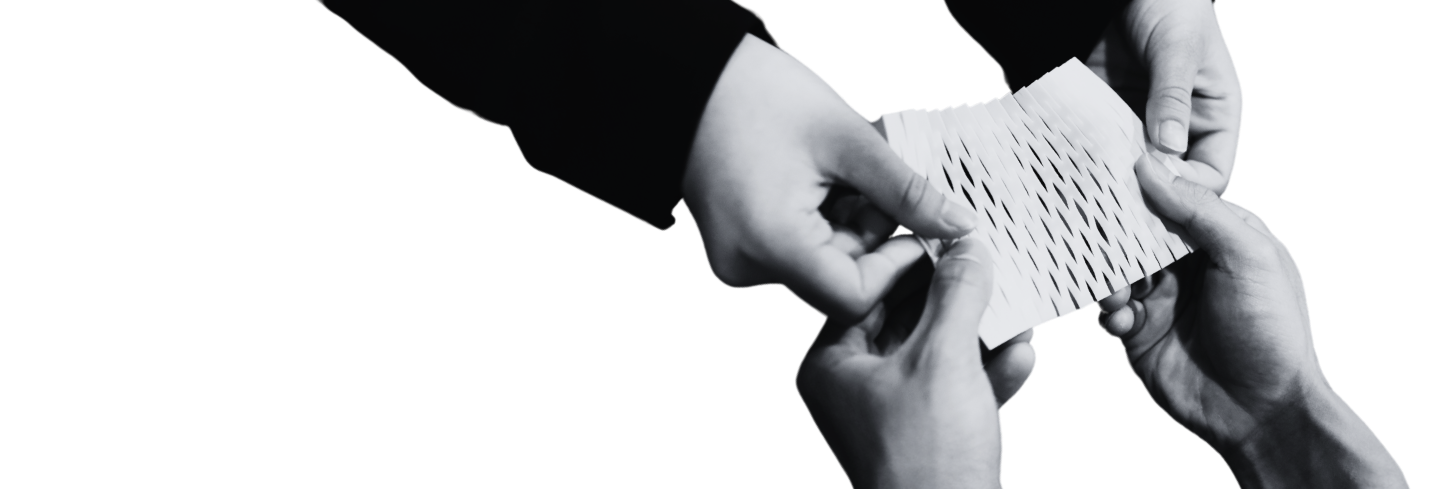
Create
Design Project Gallery
From Prompt to Silicon: Verifying Spiking Neural Networks Synthesized by LLM
- Program: Electrical and Computer Engineering
- Course: EN.520.440/640 Machine Intelligence on Embedded Systems
- Year: 2025
Project Description:
This project showcases the functional verification and live demonstration of a custom silicon chip implementing a Recurrent Spiking Neural Network (RSNN)—a bio-inspired architecture designed entirely through natural language prompts to a Large Language Model (LLM), specifically ChatGPT-4.
The RSNN was synthesized from Verilog code generated by ChatGPT, validated on tasks like XOR, IRIS classification, and MNIST, and ultimately fabricated through the open-source TinyTapeout ASIC shuttle using SkyWater’s 130nm process.
To verify the fabricated chip, our team developed a complete hardware-in-the-loop test framework using microcotb, a Python-based cocotb-like environment adapted for embedded systems. We also created custom MicroPython firmware for the Raspberry Pi Pico to enable direct communication with the chip for streaming neuron parameters, injecting spike inputs, load test scripts and reading output spike trains in real time.