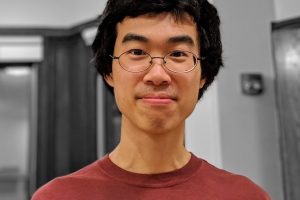
Holden Lee joined the Department of Applied Mathematics and Statistics this summer from Duke University, where he was a postdoctoral fellow. In this Q&A, he describes his research and shares a bit about himself.
Tell us a little about yourself.
My family is from Taiwan, but I was born in Maryland and grew up in College Park. I received my undergraduate degree in mathematics at Massachusetts Institute of Technology (MIT). I later moved to the UK for my graduate studies, where I received my master’s in mathematics from the University of Cambridge. I then relocated to New Jersey to earn my PhD at Princeton University, followed by a postdoctoral fellowship at Duke University. In addition to my academic and research work, I enjoy visiting national parks and have learned to speak Esperanto, a constructed language.
Can you give us an overview of your research?
My main research focus is to build theoretical foundations for probabilistic methods in modern machine learning with a view towards designing more efficient and reliable algorithms. This includes understanding deep learning-based generative models, as well as proving convergence guarantees for sampling algorithms such as Markov Chain Monte Carlo. I aim to develop theory to compare different generative models, understanding what works and under what setting, rather than simply relying on practical experience. This theoretical analysis helps shed light on practical shortcomings such as mode collapse and ill-conditioning to suggest ways to overcome them.
What drew you to this field and focus area?
My background was in pure math, but I later grew interested in computer science, and artificial intelligence. When I was in graduate school, deep learning was really beginning to take off. It is empirically successful and has great potential to change the world, but we still do not have a nuts-and-bolts understanding of how it works. It was a wonderful opportunity to come in with a mathematical point of view, to build a theory and understand how things work from the ground up. Probability theory brings a lot of useful ideas for designing and analyzing machine learning algorithms. For example, stochastic differential equations are an integral part of the recently successful score-based generative models and can also model deep neural networks to suggest optimal settings of hyperparameters.
What excites you about bringing this work to Johns Hopkins?
Johns Hopkins is a great environment with a lot of intellectual freedom and the opportunity for collaborations between different departments. For example, many people work on medical applications or in the Applied Physics Lab, and this offers researchers the opportunity to branch out. People here are very intellectually curious and not just narrowly focused within one research area. Finally, I really enjoy the friendly atmosphere in our department.
Tell us about the real-world applications of your research.
Machine learning and AI algorithms are being woven into the fabric of our society, and it is important to have a better understanding of how they work. We want to have control over the algorithms we are using in the world, make sure they are robust and safe in various circumstances—particularly important for applications such as self-driving cars—and they capture the metrics we care about—such as diversity in real-life distributions. It is important to understand what the algorithms are trying to optimize, because it may be different from what we intended. Having a better mathematical understanding is crucial, so that we have more options for designing these algorithms and understand what guarantees they have and do not have.
What are some of your goals for this first year at JHU?
I would like to start collaborating with those from within the department or from adjacent fields. A substantial portion of my work has been more theoretical, such as analyzing convergence of algorithms. I think it will be great for me to start more applied collaborations so that I can understand issues that come up in practice.
Anything else we should know?
One: I have visited 48 states in the U.S. Two: I love hiking and being outdoors, and three: I enjoy writing science fiction and fantasy stories.