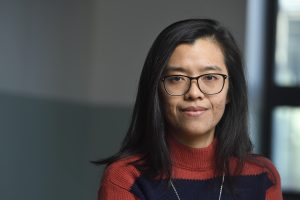
Haoyang Cao is a new assistant professor in the Department of Applied Mathematics and Statistics. Her research focuses on using machine learning to solve high-dimensional stochastic games with large populations. Cao joins Hopkins from the Centre de Mathématiques Appliquées, École Polytechnique, where she was a postdoctoral researcher.
Tell us a little about yourself.
I was born and raised in Beijing, China. After high school, I went to the University of Hong Kong to complete my undergraduate studies in mathematics. During that time, I also visited the University of Toronto for a semester of exchange study. Later, I went to the University of California at Berkeley to pursue my PhD study in operations research. Afterward, I joined the Alan Turing Institute in London as a research associate in machine learning for finance. Later on, I took up a postdoc position at the Centre de Mathématiques Appliquées, École Polytechnique. Outside of work, my biggest passion would be music. I learned to play the piano and the clarinet when I was young.
Describe your research.
My research interests can be roughly divided into two categories. One is about stochastic differential games and mean field games, together with related topics of applied probability, stochastic analysis, and partial differential equations. The other is machine learning, especially generative models, transfer learning, and Inverse reinforcement learning. A majority of the problems in the above areas arise from the fields of finance, economics, and operations research and it is equally fascinating to see how analytical and computational tools can help solve practical problems in these fields.
What are some real-world applications of your research?
Oftentimes, the research problems that I am working on come from finance and operations research. In return, the mathematical models developed in my research not only enhance our understanding of observed phenomena but also offer practical strategies to address the underlying issues. For instance, by studying stochastic games with impulse controls, we could better solve the optimal investment problem with the presence of transaction costs; the MFGAN algorithm could help solve high-dimensional decision-making problems among large populations of agents; and the transfer learning framework and the associated risk measure could benefit the portfolio optimization problems arising from newly emerged markets.
What drew you to this field and focus area?
During the first year of my undergraduate study at HKU, I took a seminar course. The first piece of reading material was selected from the book Mathematics and Politics: Strategy, Voting, Power, and Proof by Alan D. Taylor and Allison M. Pacelli. While not a single commonly seen mathematical symbol appeared in the material, the meticulous logical deduction cracked open my old view of math. For me, math began to transform into a way of seeing the world, not just about computations, theorems, and proofs. This marked the beginning of my interest in general game theory and mathematical modeling as well.
What excites you about bringing this work to Johns Hopkins?
During my PhD study, I took upon problems in stochastic differential games and mean-field games, combined with various forms of controls. These are interesting and powerful analytical tools to explain human behaviors observed in modern economic and financial activities. Empowered by the fast development of machine learning techniques, we are looking at more efficient solutions to decision-making problems in real life. I believe that I joined the JHU community at an exciting point in time when the university is expanding its impact on data science and artificial intelligence. I am really looking forward to collaborations with researchers from different fields.
What are some of your goals for this first year at JHU?
I hope that I can adapt to the working rhythms within the school and the department as soon as possible. I am offering a new course, Machine Learning in Finance, in the spring of 2024. Hopefully, through this course, I can attract talented students to this rapidly growing field of research. In addition, I hope to connect with fellow faculty members who share similar research interests and start to create more Machine Learning in Finance research opportunities.
Anything else we should know? Any fun facts?
Though far from being fluent, French is now seriously influencing my English vocabulary and accent after staying in Paris for almost two years. But I would like to continue learning French, so if anyone needs a language companion, please let me know.