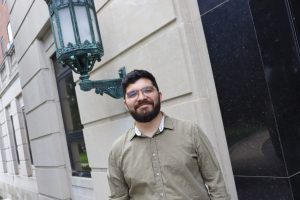
Mateo Díaz is a new assistant professor in the Department of Mathematics and Statistics with a secondary appointment in the Mathematical Institute for Data Science. Díaz‘s research sits at the intersection of continuous optimization, geometry, and statistics, with a focus on applications in data science, machine learning, and signal processing. He joined Hopkins from a post-doctoral fellowship at the California Institute of Technology.
Tell us a little about yourself.
I am originally from Colombia, South America. I grew up in the border villages of Tabatinga and Leticia, between Brazil and Colombia. I relocated to Bogota, Colombia’s capital, to pursue undergraduate degrees in mathematics and in systems and computing engineering, as well as a master’s degree in mathematics at the Universidad de Los Andes. I later moved to the United States to pursue a PhD in applied mathematics at Cornell University. Following that, I worked as a postdoctoral scholar at Caltech for two years. Outside of work, I like to go riding, hiking, and play video games with my buddies.
Describe your research.
I am broadly interested in the mathematics of data science, with a distinct focus on continuous optimization and high-dimensional statistics. On the one hand, I work on designing and analyzing optimization algorithms to solve problems arising from data-science applications. In the past, I have worked on theoretical guarantees and practical implementations of iterative algorithms for convex and nonconvex large-scale problems. In the meantime, I’m working on high-dimensional statistics, specifically estimation problems like low-rank matrix recovery and clustering. I’m curious about questions like, “Can we solve a problem given our data constraints even with limitless computational resources?” What is the bare minimum number of samples required? Which algorithm should we use? I’m fascinated by the intersection of optimization and statistics, particularly the interaction of computational and statistical resources.
What are some of the real-world applications of your research?
Optimization problems appear across the sciences and engineering. For instance, they are used when training large language models, when planning the logistics of a delivery company, or when controlling robots and drones. These applications often involve problems with too many variables and constraints, making them difficult to tackle using traditional optimization methods such as the simplex and interior point methods. My research focuses on efficient methods that can handle much larger problems. On the other hand, I have done work on core statistical problems, such as clustering. That is the problem of classifying the elements in a data set into two or more groups. Clustering has a wide range of applications, including compression, computer graphics, and network analysis. My work focuses on provable algorithms that can tackle clustering problems that are challenging for standard methodologies, e.g., k-means or the principal component analysis.
What drew you to this field and focus area?
Early on, I realized that optimization and statistics offer foundational tools that allow people to tackle problems in several domains, and this fascinated me. I can point to two undergraduate classes that ignited this idea in my head. The first one was Linear Programming. I remember that on the first day of class, the TA asked us to minimize the cost of groceries while satisfying certain constraints. The cause of the problem was not mathematical; it was simply a “real world” problem. We were asked to solve it by hand (with the help of a spreadsheet if we wanted). A friend of mine and I spent the rest of the class trying to figure out how to solve the problem, and I remember being puzzled because I did not know how to ensure we found the best possible answer. That class got me excited about algorithms, theoretical guarantees, and the idea of optimality.
Later, I took a Nonparametric Statistics course. The class was beautiful; it completely changed my conception of statistics. My first introduction to statistics gave me the idea that statistics focuses on a handful of distributions we understand well. But this class taught me that it was much more general, as I learned about distribution-free methods for hypothesis testing, estimation, and regression. The professor motivated most ideas with applications, and the assignments blended programming and theory. Afterward, I fell in love with understanding the statistical nature of data.
What excites you about bringing this work to Johns Hopkins?
I am incredibly excited about joining Johns Hopkins University, an institution I deeply admire for its interdisciplinary nature. Hopkins is renowned for its exceptional contributions to the medical domain. However, its excellence extends beyond medicine, as evidenced by its recent strides in other areas of study. The recently established Mathematical Institute for Data Science and SNF Agora Institute are just some examples of these efforts. As someone who envisions himself as a tool builder for engineers and scientists, I see Hopkins as the perfect incubator for fostering collaborations. Equally exciting to me is the idea of joining the department, as it offers a fantastic mix of seasoned academicians and vibrant new hires. I look forward to contributing to and learning from such an intellectually stimulating environment.
What are some of your goals for this first year at JHU?
Transitions often take time, so I plan to learn and adapt to the inner workings of Hopkins. I will teach a graduate optimization class in the fall, and I hope to do justice to the beauty of the field and get students excited about it. I am also looking forward to working with students. Lastly, I plan to start collaborating with researchers in applied domains that could benefit from some of the methodologies I have been thinking about.
Anything else we should know? Any fun facts?
I was raised in a small town at the heart of the Amazon jungle. My hometown lies on the border with Brazil, so I picked up Portuguese early on. Now, after living in the US for many years, I find English words often sneaking into my Portuguese conversations. I enjoy geeky activities such as coding and tinkering with mechanical keyboards and reproducible operating systems.