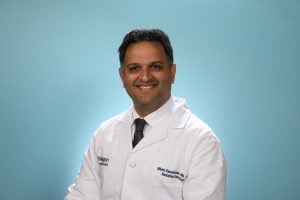
Nikhil Rammohan, Engr ’09, arrived at Johns Hopkins set on studying chemical engineering. But then came a statistics class—and a research opportunity with Carey Priebe, professor in the Whiting School of Engineering’s Department of Applied Mathematics and Statistics and director of the Mathematical Institute of Data Science—that nudged him in a different direction. What started as a shift in academic interest turned into something much bigger: a passion for data, problem-solving, and ultimately, medicine. He went on to earn both a medical degree and a doctorate at Northwestern, applying data science to medical imaging along the way.
We caught up with Dr. Rammohan, who is now a radiation oncologist at Washington University in St. Louis, to talk about how statistics opened the door to oncology, the mentor who helped guide him, and how research continues to fuel his work.
What was it about a statistics class that led you to shift from chemical engineering to applied math and statistics?
The required statistics class I took during my freshman year immediately resonated with me in a way my engineering courses didn’t. I was also introduced to research opportunities with Professor Carey Priebe, and that practical application of statistics sealed the deal. While chemical engineering felt disconnected from my personal interests, statistics had both theoretical richness and real-world impact—especially in the context of medical imaging. That immediate relevance to human health and decision-making really pulled me in. It wasn’t just about equations; it was about translating data into understanding that could make a difference.
You’re now a radiation oncologist whose work draws heavily on statistical concepts. How has your early foundation in applied mathematics and statistics shaped your career in oncology?
That foundation has been critical. I was learning about machine learning and deep learning in 2008—well before AI became mainstream. The skills I developed at Hopkins, especially under Dr. Priebe’s mentorship, directly inform my current research. For instance, the same morphometric techniques I used to study brain surface changes in patients with depression or Alzheimer’s are now helping me identify radiation-induced changes in cancer patients’ brains. The continuity between those two periods, separated by more than a decade, is striking. It’s a testament to how forward-thinking our work at Hopkins was, and how essential that skill set remains in modern medicine.
Can you describe one of your current research projects that connects to your work at Hopkins?
One project I’m proud of is an ongoing study that analyzes how brain surfaces change after radiation therapy in cancer patients. Interestingly, I’m using the same statistical surface mapping techniques from my undergraduate and master’s theses, which focused on patients with depression and Alzheimer’s. Back then, we showed that certain brain surface patterns correlated with cognitive and emotional symptoms. Now, we’re finding that radiation can induce similar patterns, potentially explaining some of the cognitive side effects seen in patients post-treatment. In fact, I cited my early work in a recent paper, which was a very full-circle moment.
What are the biggest challenges you face in radiation oncology, and how does your research tackle them?
One of the major challenges is the limitation of medical imaging itself. As radiation oncologists, our treatment decisions are only as good as the imaging data we rely on. So, improving the interpretation and quality of those images is central to improving outcomes. That’s where my research comes in—leveraging machine learning and statistical modeling to extract more meaningful information from imaging data. The goal is to enhance precision: to know exactly what and where to treat, and what to spare. In many ways, my work is about bridging the gap between raw data and clinical decision-making.
How did Professor Carey Priebe’s mentorship shape your path, and how do you now mentor others?
Carey wasn’t just a brilliant academic—he was incredibly charismatic and passionate, which struck me as a young, impressionable student. But beyond that, he was supportive through every high and low. Whether I was stuck on a problem or doubting my path, he always made time for me. That unwavering support was key in helping me navigate towards an MD/PhD path and beyond. Now, as a mentor myself, I try to embody those same principles: be passionate, be available, and most of all, believe in your students even when they don’t yet believe in themselves.
What advice do you offer students in applied math or statistics who are interested in pursuing a career in medicine or medical research?
First and foremost, follow your passion. Don’t feel limited by traditional career paths—there’s room for creativity, especially in interdisciplinary spaces like medicine and data science. Make sure to get a solid foundation in applied statistics, machine learning, and data analysis—they are more relevant now than ever. Also, seek out mentors who inspire you and challenge you. The right mentor can open doors and guide you through critical decisions. If you stay curious and grounded in both technical skill and human-centered goals, you’ll find your place.