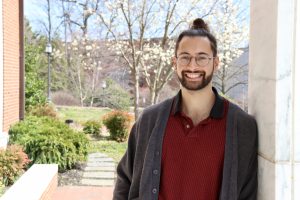
Multilayer network research seeks to understand and model complex multilevel systems, from human social connections to transportation systems and more. The key to successful network analysis is to use all the system’s dynamic layers to uncover and elucidate structures, according to Joshua Agterberg, a doctoral candidate in the Department of Applied Mathematics and Statistics, whose research focuses on network science.
In a recent study published on ariXiv, Agterberg and his team propose a new method of analyzing complex network datasets containing multiple layers that change over time. They found that their “multilayer degree-corrected stochastic blockmodel,” which groups individuals or nodes into communities based on their connectivity patterns and considers the number and strength of connections each node has, easily and efficiently identified patterns in those networks.
In the paper, the team tested its technique on a real-world example, US airport data, revealing trends in airport popularity from January 2016 to September 2021, when the COVID-19 pandemic was underway.
“We observed these networks for each month, tracking how flights change over time, to see the impact of seasonal travel and the pandemic on flight patterns,” he says. “Though the overall number of flights within and between communities decreased after the pandemic started, the impact on the airport traffic was not the same across the system. For instance, airports in the New York City area were more negatively affected than were the airports in Atlanta, Dallas-Ft. Worth, and Chicago-O’Hare, possibly due to pandemic dynamics and related closures.”
The team concludes that their method is “simple and efficient,” and allows the most expensive computations to be performed in parallel, meaning that the method to be scaled to handle large datasets.
Agterberg is also interested in delving into what he calls a ‘’finer-grained analysis for algorithms.’’ In most cases, algorithm results do not provide enough information to understand why an algorithm works or does not work, he says. He plans to analyze these problems statistically, and then study problems related to hypothesis testing
“High-dimensional matrix, tensor data, and multilayer networks are becoming increasingly more common in applications such as neuroscience and image analysis, and through my research I hope to develop a general statistical framework that allows us to characterize the uncertainty inherent in these problems, paving the way towards a better understanding for these types of data and algorithms,” says Agterberg, who will soon become a post-doctoral fellow at the University of Pennsylvania, where he will focus on theoretical analysis of matrix and tensor data.
Agterberg says he will miss many things about his time at Johns Hopkins, including his experience using the wizarding world of Harry Potter to teach Introduction to Network Science, offered each fall since 2021.
In the class, he teaches linear algebra and statistics to undergraduate math and non-math majors, demonstrating how to analyze data from the Harry Potter dataset – an online trove of facts and statistics about the friendships and rivalries of the characters at Hogwarts – without getting too “scientifically involved.”
By the end of the course, he says his students have mastered the fundamentals of basic network analysis.
“I tried to convey to the students that if I give them this dataset and tell them to find and identify some communities, can they do so in a meaningful and principled way. The goal is to show them a step-by-step process of doing this in a logical way, which will ideally produce results that are easier to understand,” he says.
Following his postdoc work, Agterberg will join the faculty at University of Illinois at Urbana-Champaign, where he will continue his research in network analysis—particularly in multi-layer network analysis, as well as analyzing various complex procedures to better understand algorithm output.