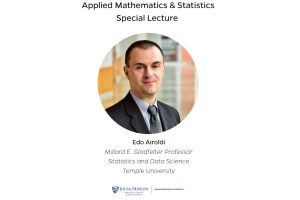
Title: Designing experiments on social, healthcare, and information networks
Abstract: Designing experiments that can estimate causal effects of an intervention when the units of analysis are connected through a network is the primary interest, and a major challenge, in many modern endeavors at the nexus of science, technology and society. Examples include HIV testing and awareness campaigns on mobile phones, improving healthcare in rural populations using social interventions, promoting standard of care practices among US oncologists on dedicated social media platforms, gaining a mechanistic understanding of cellular and regulation dynamics in the cell, and evaluating the impact of tech innovations that enable multi-sided market platforms. A salient technical feature of all these problems is that the response(s) measured on any one unit likely also depends on the intervention given to other units, a situation referred to as “interference” in the parlance of statistics and machine learning. Importantly, the causal effect of interference itself is often among the inferential targets of interest. On the other hand, classical approaches to causal inference largely rely on the assumption of “lack of interference”, and/or on designing experiments that limit the role interference as a nuisance. Classical approaches also rely on additional simplifying assumptions, including the absence of strategic behavior, that are untenable in many modern endeavors. In the technical portion of this talk, we will formalize issues that arise in estimating causal effects when interference can be attributed to a network among the units of analysis, within the potential outcomes framework. We will introduce and discuss several strategies for experimental design in this context centered around a useful role for statistics and machine learning models. In particular, we wish for certain finite-sample properties of the estimator to hold even if the model catastrophically fails, while we would like to gain efficiency if certain aspects of the model are correct. We will then contrast design-based, model-based and model-assisted approaches to experimental design from a decision theoretic perspective.
Biography: Edo Airoldi is the Millard E. Gladfelter Professor of Statistics and Data Science, and a Professor of Finance (by courtesy), in the Fox School of Business, at Temple University. He also serves as Chair of the Department of Statistics, Operations, and Data Science, and as Co-Director of the Temple Data Science Institute.
His research focuses on statistical methodology and theory for designing and analyzing randomized experiments and observational studies in complex settings, and on modeling and inferential issues that arise in analyses that leverage network data. Airoldi also developed methodology to generate insights from genomics and proteomics data, and to generate signals from unstructured text data. He enjoys working on applications in systems biology, healthcare, the tech industry, and finance.
Current projects include methodology for designing and analyzing experiments, and identification strategies for causal analyses, with applications to: (1) to social healthcare in rural Honduras in collaboration with the Yale Institute for Network Science; (2) search and display ad auctions in collaboration with the Market Optimization Algorithms group at Google in New York City; (3) ML algorithms to improve aspects of systematic trading in collaboration with the Global Equities Division at Wells Fargo Securities; and (4) improved randomizations and analysis strategies in multi-sided markets collaboration with Amazon Supply Chain Optimization Technology.
Airoldi has received several honors and awards for his research, including the Outstanding Statistical Application Award from the American Statistical Association, a Sloan Fellowship, the Shutzer Fellowship from the Radcliffe Institute of Advanced Studies, an NSF CAREER Award, and an ONR Young Investigator Program Award. He has delivered a plenary talk at the National Academy of Sciences Sackler Colloquium on “Causal Inference and Big Data,” in 2015, and he has given an IMS Medallion Lecture at the Joint Statistical Meetings, in 2017. He is an elected Fellow of the Institute for Mathematical Statistics, since 2019, and of the American Statistical Association, since 2020.
Airoldi earned his PhD in Computer Science from Carnegie Mellon University, where he also received Master of Science degrees in Statistics and Statistical and Computational Learning. He earned a Bachelor of Science in Mathematical Statistics and Economics from Italy’s Bocconi University. Before and after his PhD, Airoldi has worked in industry in a variety of roles, for companies ranging from early-stage start-ups to established industry leaders.