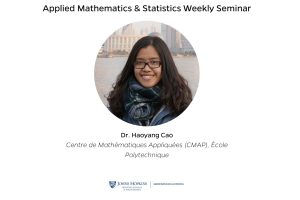
Location: Gilman 132
When: February 16th at 1:30 p.m.
Zoom Link: https://wse.zoom.us/j/95738965246
Title:Bridging GANs and Stochastic Analysis
Abstract: In this talk, we mainly focus on a bidirectional bridge between generative adversarial networks (GANs) and stochastic analysis.
The motivation to build such a bridge stems from the empirical success of GANs in fields of artificial intelligence such as computer vision, as well as the need of viable tools in financial mathematics to either simulate testing data or solve the mathematical problems. Many of these problems in financial mathematics are deeply rooted in stochastic analysis. The model of meanfield games (MFGs) is one of the examples. It studies the decision making problem under weak interactions among large population of market participants. To leverage the empirical success of GANs to tackle the well-known challenge in deriving analytical solutions to generic MFGs, we first draw the conceptual analogy between GANs and MFGs. Then, based on this analogy, we propose an algorithm called MFGAN that solves MFGs with high accuracy with relatively less computational time.
As the financial industry quickly embraces GANs as well as other machine learning tools, there are still mysteries in the theoretical understanding of these tools. Take GANs as an example, how do we interpret the adversarial nature in its formulation and its training process? Is there anything we could do to better understand the stability issue occurred in its training? To this end, stochastic analysis, which we often use to model financial mathematics problems, can be used to deepen our theoretical understanding of GANs. For its mathematical formulation, we notice that, apart from being an obvious two-player minimax game between generator and discriminator, GANs can also be seen as mean-field collaborative game where the “mean-field” effect comes from “big data”. This new perspective tells us the limit in GANs performance. For the training of GANs, we can use a system of stochastic differential equations (SDEs) to establish a proper approximation. In particular, the adversarial nature of GANs can be embedded in the drift term of the SDEs. With the help of such an SDEs approximation, we can also characterize the longrun behavior of GANs training via the notion of invariant measure and this characteristic prompts us to properly schedule the learning rate for a better training result. The establishment of SDEs approximation as well as the characterization of long-behavior of GANs helps us understand, from a mathematical point of view, some of the common practices in GANs training including the choices of loss functions, the structure of the neural networks, the penalty applied to the gradient and so on.
This talk is primarily based on “Connecting GANs, mean-field games, and optimal transport” by Cao, Guo and Lauri`ere, and “SDE approximations of GANs training and its long-run behavior” by Cao and Guo.
Biography: Haoyang Cao received her Ph.D. (2020) from the Department of Industrial Engineering and Operations Research at the University of California, Berkeley, under the supervision of Prof. Xin Guo. Her thesis was on mean-field games, generative adversarial networks and the interplay between them. Later she joined the Alan Turing Institute (ATI) in the UK as a machine learning in finance research associate. During her stay at ATI (2020-2022) she worked on the identifiability issue in inverse reinforcement learning jointly with Prof. Lukasz Szpruch from the University of Edinburgh and Prof. Samuel N. Cohen from the University of Oxford. She is currently a postdoctoral researcher at Centre de Math´ematiques Appliqu´ees, Ecole Polytechnique. With Prof. Mathieu ´ Rosenbaum, she is studying the mathematical framework of transfer learning and in particular the transferability issue.
Her research interests span two major directions. The first one is about stochastic controls, stochastic differential games and mean-field games. Along this direction, she is especially interested in the modeling problems with impulse controls and singular controls. The other direction is on the study of machine learning. Her interest in machine learning was motivated by the need of developing computational tools to solve for explicit solutions to high-dimensional stochastic games. In return, her studies on stochastic controls and games enriched the theoretical understanding of many machine learning paradigms including generative adversarial networks, inverse reinforcement learning, meta learning and transfer learning. In addition, she is working on applying her skill set of stochastic analysis, modeling and machine learning techniques to applications in financial mathematics.