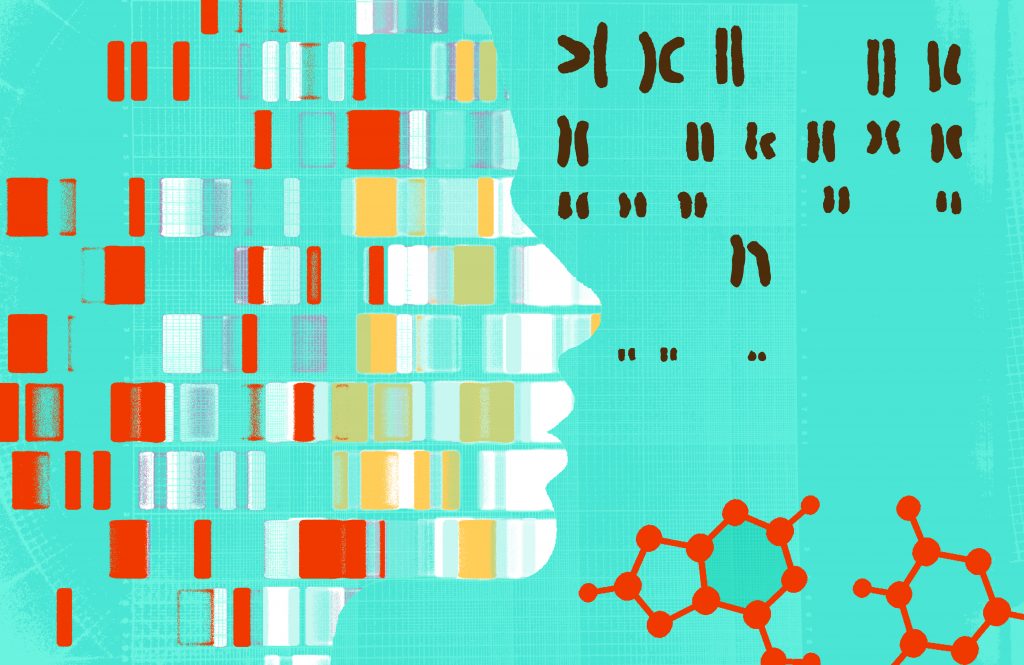
It’s enough to make your head spin. Virtually every cell in the body contains a complete copy of the approximately 3 billion DNA base pairs, or letters, that make up the human genome. Thanks to dizzying advances in technology, scientists are poised to unlock the secrets of the genome in an ambitious effort to transform the diagnosis and treatment of disease.
Meet four computer scientists here at the Whiting School who are on the front lines of this 21st-century quest.
Reaching for the Moon
Think of genomics as astronomy turned inside out. Instead of looking out into the infinite vastness of space to grasp the workings of the universe, the field is pointed inward at depths of biology, where genes, proteins, and molecules operate amid their own brand of cosmos.
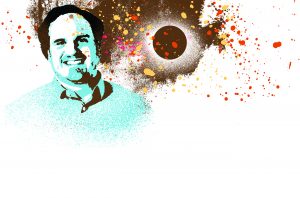
Both fields produce volumes of data at a rate that is, well, astronomical. A few years back, Michael Schatz published a fun paper along these lines, arguing that before long, we might end up having to replace that space-oriented adjective with some variation of the word genomical.
“We think there is currently somewhere between 30 and 50 petabases of sequencing data being produced every year,” says Schatz, a Bloomberg Distinguished Associate Professor of Computer Science and Biology. The petabyte is 1 million times bigger than the gigabyte, so that puts this annual output at 30 to 50 million billion bytes of information.
If all the sequencing data collected to date in genomics were loaded onto razor-thin DVDs, the stack of disks would stand nearly 1 mile high. This is the mass of data that Schatz mines by way of complex computer algorithms and machine learning strategies. He is in search of genetic factors at work in medical conditions as varied as cancer, autism, and bipolar disorder.
Consider autism, for example. “If we look at the data from any one individual in isolation, there’s almost nothing in there that’s recognizable as potentially important,” he says. “It’s only through the power of hundreds and then thousands and then hundreds of thousands of families that we start to see patterns emerge.”
Researchers in Schatz’s lab already have sorted through sequencing data from parents and children in more than 3,000 families where one sibling has autism and another does not. Along the way, they have assembled a list of some 500 genetic mutations that may be involved in the disease. The mix of mutations varies widely from individual to individual in ways that may help determine where any one case lands along the spectrum of mild to severe.
One groundbreaking aspect of this work is the way Schatz’s lab is scouring the whole of the genome for these clues. To date, the hunt for genetic culprits in human disease has focused mostly on the genes that carry codes to help the body produce proteins. But those genes make up just 2 or 3 percent of the genome’s physical material.
Schatz compares the story here to a Broadway show, with the traditional coding genes taking on the starring roles of actors on stage.
But there is an endless array of important things going on behind the scenes with a Broadway production—costumes, lighting, direction, script writing, and marketing among them.
“We’re trying to better understand how the big actors on stage inside the gene are regulated from behind the scenes,” Schatz says.
This same line of questioning drives another category of work in Schatz’s lab, where the study of various agricultural species takes up as much time as work on the human genome. “You can do experiments in plants that you couldn’t possibly do in humans,” Schatz explains. “You can breed them in certain ways. You can stress them in certain ways.”
Sugarcane is one plant of special interest. Because farmers have been tinkering with its makeup for centuries through breeding experiments, the modern-day incarnation of the plant has an exceptionally complex genetic character. Where human cells carry copies of two strands of chromosome source material, one from each parent, sugarcane cells carry between nine and 14 copies of every chromosome.
Interestingly, similar complexities can also be seen in some genetic disorders, especially human cancers. Schatz’s lab is now looking at a type of breast cancer, for example, where certain stretches of code in the DNA are copied out 14 times instead of two. They also have identified a previously unrecognized set of mutations working in the control room of pancreatic cancer cells.
“We see this kind of interplay a lot,” Schatz says. “We can develop methodologies to see what’s happening in some of these more complicated plant species, and then we find ourselves back in the human genome, looking at this similar kind of complicated behavior in the context of cancer and other diseases.”
Taking questions about these similarities back into the human genome puts Schatz and his colleagues right back in that astronomical mass of data stretching a mile into the sky. He is looking forward to the day when 50 petabytes a year seems like small potatoes, and he is happy to report that that day is coming sooner rather than later.
“Everyone in engineering knows about the famous Moore’s law and how computers double in power every 18 months or so,” Schatz says. “Well, in genomics, the rate of growth is even faster—the amount of sequencing data being collected is actually doubling every nine to 12 months.”
Next year, then, that stack of DVDs will reach 2 miles high, and then 4, and then 8. And somewhere in the range of 10 to 15 short years from now, that stack will reach to the moon.
“When we get to the moon, it’s going to give us amazing power to see all these different patterns and make all the connections we’d like to make,” Schatz says. “It’s just such an exciting time to be working in this field.”
The Microbiome Moment
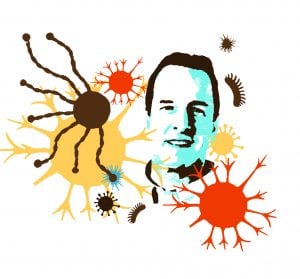
The notion that genomics might spur the development of novel diagnostic tools useful in the fight against human illness is as old as the field itself. Until now, that work has focused mostly on the hunt for disease-causing glitches inside human DNA.
Steven Salzberg is moving this hunt onto nonhuman terrain. The Bloomberg Distinguished Professor of Biomedical Engineering, Computer Science, and Biostatistics is looking to use genetic sequencing as a tool to identify the pathogens at work in infections.
A much-decorated pioneer in the field, Salzberg worked in the 1990s on one of the key teams involved in the race to sequence a human genome for the first time. He describes his latest work as a variation on microbiome analysis, which involves conducting a genetic sweep through a batch of organic material. The studies along these lines that tend to receive attention in the popular press involve finding various scary bacteria lurking on the surfaces of everyday life, such as cellphone screens or automated bank teller keypads.
Salzberg is conducting those sweeps in tissue samples taken from patients with infections of the brain, the eye, or other body parts. The goal is to pinpoint the pathogen behind the trouble in any given case and give physicians a quicker, more accurate way to identify the best treatment option.
“One reason I’m interested in infectious disease diagnosis is that we have treatments that work [for them], right now,” he says. “It’s different with genetic defects. There, identifying a causative mutation is a critical first step, but the treatment part—how do you treat a genetic defect? That’s a much harder question.”
Using genomics to identify infectious pathogens is a needle-in-the-haystack affair. It involves scouring through tens of millions of DNA sequences in search of as few as 20 sequences that represent the culprit.
“If someone tried 10 years ago to do what we’re trying now,” Salzberg says, “people would have been like, ‘You’re going to spend all this money, and then you’re just going to throw away 99.9 percent of the data?’”
That’s exactly what Salzberg is doing in a pair of partnerships with faculty members in the School of Medicine. He is looking at infections of the brain with the neurologist and pathologist Carlos Pardo-Villamizar and at infections of the eye with the neuropathologist Charles Eberhart. If the concept works in these two areas, it will almost certainly work in infections elsewhere in the body.
“I’ve been thinking about this work for a long time,” Salzberg says, “but I had to wait for technology to catch up and make it possible.” A pair of recent developments helped move this project to the top of Salzberg’s priority list. First, researchers have now sequenced the vast majority of bacteria and viruses that might pop up in his sweeps. That gives him a reasonably complete reference library so that he can properly identify the few needles in that haystack.
“If there’s a pathogen that affects people with any frequency at all, we’ve not only sequenced it, we’ve probably sequenced multiple strains of it,” he says.
The second development is the astonishing rate at which DNA sequencing has become faster and cheaper over time. In the late 1980s, when scientists first set their sights on deciphering a human genome, sequencing was running about $10 per base pair. Experts back then had their fingers crossed that it would come down to $1 per base pair by the turn of the 21st century.
“So we were hoping it was going to get 10 times more efficient—a big number, right?” Salzberg says. “By the time we actually sequenced the genome in 2001, we were doing 800 base pairs for a dollar—that’s 8,000 times cheaper.”
The pace has only accelerated since then. Today, a single machine can sequence an entire genome in a day for about $1,500. The sweeps that Salzberg is conducting on tissue samples run about $1,000 each, but he sees them getting down in the range of a few hundred dollars before long.
The first proof-of-concept paper based on this work appeared last year in the journal Neurology: Neuroimmunology & Neuroinflammation. Salzberg expects that to be just the first in a run of papers as the microbiome project moves from concept to clinical reality.
His lab is now identifying infectious agents in eye tissue with 80 percent accuracy. In brain infections, where the work is much more complicated by a number of factors, including uncertainty about whether an infection is present at all, the rate of positive detection is running at 25 percent.
Salzberg expects that number to improve, and quickly. He predicts that within two or three years, tests like this will be conducted on a regular basis at Johns Hopkins and other academic medical centers that are strong in genomics. Soon thereafter, the tests could scale up to the point where they are available to community physicians—the genetics equivalent of sending a blood sample to the lab.
“I really think that this has the potential to transform the way we diagnose infections in this country and around the world,” he says.
Still Counting
At times, the science of genomics looks to be advancing at breakneck speed toward a future where it realizes its enormous potential to boost our understanding of the human body and help treat its ailments. At other times, the field looks to be still in its infancy. Case in point: Scientists don’t know yet how many genes there are in the human body.
Over the last 20 years, estimates have ranged from 50,000 to 100,000 genes, then 25,000 to 40,000, then down another notch, to 20,000 to 25,000. Seven years ago, Steven Salzberg and his colleague Mihaela Pertea, MS ’98, PhD ’02, in the Johns Hopkins University Center for Computational Biology conducted a review of all the data sets then in existence and came up with a number just north of 23,000.
“I think it’s about time that we finally got an answer, at least to two significant digits,” Salzberg says. He and Pertea are now returning to this question from a fresh angle. Rather than look through the lens of the genome of a single individual, they are looking at RNA sequencing data involving more than 500 individuals and 30-plus different tissue types housed in the Genotype-Tissue Expression database maintained by the National Institutes of Health.
The two hope to have a new paper out by the end of the year that gets the field closer to a final number. That result may prove more than a matter of academic curiosity. Currently, three different genomic centers maintain “lists” of human genes, each numbering somewhere under 20,000 genes. These are the libraries that clinical geneticists consult when looking for genes that might be involved in rare inherited diseases. Cancer geneticists turn to these same libraries when looking for rare mutations at play in their field. A more complete library based on the work of Salzberg and Pertea might well lead to better results in both areas.
The Power of Prevention
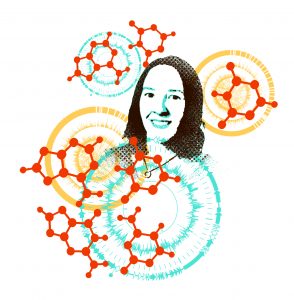
One after another, the reports pop up in the media. This genetic flaw has been linked to breast cancer, that one has been tied to diabetes, and another has been associated with depression. Factual one and all, the headlines can still create a misleading impression about the progress scientists have made to date in unraveling the mysteries of the human genome.
“This is still a vast, unknown space in many ways,” says Alexis Battle, an assistant professor of computer science. Her specialty is developing computational biology tools and machine learning strategies that can sort through masses of sequencing data for clues that help predict the consequences of genetic variation—the differences each of us carries in our individual genetic sequence—including potential health risks.
“If you got sequenced today, they might identify a few interesting-looking genetic changes,” she says. “But the truth is, for most of the genetic variants they found, we would have no idea at all what they might mean for your health.”
In Battle’s lab, one focus is on pinpointing risks tied to rare genetic variants. These are just what they sound like—genetic glitches that are few and far between in the human population. But rare as any individual variant might be, the variants in a collective sense are really quite common.
“If I sequenced your genome, I would find somewhere around 40,000 and 50,000 variants that are present in less than 1 percent of the population,” Battle says. “It’s quite likely that you would have some number of variants we’ve never seen before” while sorting through scientific databases with sequencing data from tens of thousands of other people.
What are these rare variants doing? Are they disrupting the function of your cells? Can they cause diseases to arise, and if so, how often, and under what circumstances? No one has reliable answers to these questions yet.
To make matters more complicated, rare variants are most likely to reside in the estimated 98 percent of the genome that is referred to as “noncoding.” Unlike the traditional genes described in high school textbooks, these stretches of DNA do not contain instructions for producing proteins.
“What that means is that I can’t pick up a biology textbook and look up how that genetic change will affect a particular protein or how it might be tied in to some sort of health risk,” Battle says.
Her lab is working to develop novel ways to help clinicians make reliable predictions about these rare variants. The machine learning strategies developed for this work integrate sequencing data with measures of what’s going on at the molecular level in the same person.
The molecular data can help to identify what, if anything, is out of kilter in a biological sense. For example, evidence might indicate that a body is producing one important protein or another at just 10 percent of the level found in most other individuals. That sort of clue creates a trail that leads back into the mass of sequencing data, pointing to variants located in certain places or possessing certain qualities that might be linked to future health risks.
“These rare variants may be in noncoding regions of the genome, but it turns out that they can sometimes have an influence on nearby protein-coding genes by, for example, causing them to increase or decrease how much protein is produced,” Battle explains.
The end goal for Battle’s new textbook: a prioritized ranking for all of the 40,000 rare variants in any individual’s genome. The top of the list will feature the variants that are not only functioning but also might well carry health risks down the line.
So far, so good: Tests to date on this approach show that variants already known to be associated with disease do, in fact, end up on the high-priority list. Further refinements are on the way as Battle’s lab gets access to more patient data and a more precise picture of the shapes, structures, and other properties in place at various positions in the genome.
“Going forward, we’d like to take our method and apply it in cases where we’re looking for the cause of a particular disease and not just looking to understand these molecular changes,” Battle says. “Showing that this can be reliable and predictive in a disease context—that’s where I would like to see us go next. We’re definitely getting there.”
The Pathfinder
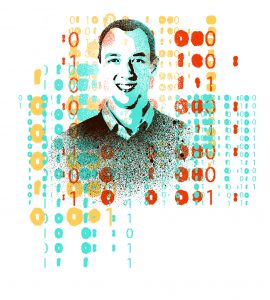
Benjamin Langmead found his way to genomics by way of a wrong answer. As a young computer scientist in the early 2000s, he had no particular interest in the field until hearing about how the then-latest generation of DNA sequencing machines were spitting out data at speeds beyond the ability of computer software to keep up.
The problem seemed familiar. Then a graduate student at the University of Maryland, Langmead had recently worked on a project for the U.S. Department of Energy where the goal was to bolster the security of a specialized computer network by developing high-speed tools to recognize dangerous snippets of text associated with spam and malware. Could he rework the solution he devised for that problem with an eye toward reading snippets of DNA coding at similarly high speeds?
“The idea didn’t work,” Langmead confesses with a laugh, “but that’s how I fell in love with the field.”
He found his way to the right answer soon enough. Working with faculty mentor Steven Salzberg and fellow graduate student Cole Trapnell, now at the University of Washington, he developed a software tool called Bowtie, which was released in 2009 and made innovative use of time-honored concepts from the computer science specialties of text indexing and approximate matching in order to bring the sorting of raw sequencing data up to speed. Bowtie is now part of an array of open-source software options known as the Tuxedo Suite. The tools it contains have been cited more than 20,000 times in scientific literature.
Langmead, now an assistant professor of computer science at the Whiting School, remains focused on the need to clear out informational logjams that pop up as the field he loves matures and grows.
The logjam currently in his sights centers on improving access to the vast array of sequencing data from patients stored in various databases, especially one called the Sequence Read Archive, which is a joint project of public science agencies in the United States, Europe, and Japan.
“Some of these data are really valuable stuff, and it’s only going to get more valuable over time,” Langmead says. “There is stuff in there from people with rare diseases. And there are data that contain multiple tissue samples from the same person—that’s a really hard data set to find if you’re a researcher.”
One problem with accessing this data trove is reproducibility. It’s one thing to set up a software package that can analyze this data on a computing cluster at Johns Hopkins. But it’s often quite another matter to then repeat that same run on a cluster somewhere else.
“We get kind of stuck right now,” Langmead explains. “If I give a large data set to a colleague at, say, Princeton, we’d probably need to send 20 emails back and forth trying to figure out how to get it to work in exactly the same way on their cluster.”
Langmead’s starting point here is big-data computing concepts from the worlds of finance and technology, especially variations on a programming model known as MapReduce. One key asset of these tools is scalability—the software involved is a seamless affair, whether the job at hand is a small one that could run on a single desktop unit or a gargantuan one requiring hundreds of larger computers. Langmead is layering DNA sequencing software on top of that foundation in ways that make it easier to set up the whole package on different clusters in different places.
“One thing I’m really advocating for here, too, is commercial cloud computing,” he adds. “Too often, people still think those services are just for big businesses. But they are actually a great fit for science too.”
Privacy rules are a key area Langmead has his eye on here. About half of the data in the Sequence Read Archive is governed by computing protocols designed to protect the identities of donors to the archive. The protocols are commonly referred to in the field as dbGaP because of their association with the Database of Genotypes and Phenotypes at the National Institutes of Health.
Langmead has developed a version of this new software that gives users working in these commercial cloud platforms the ability, with a few simple keystrokes, to abide by all of the dbGaP rules concerning encrypted data and network privacy.
“Basically, this is a big step toward making this whole ordeal into a push-a-button kind of thing,” he says. “If the science community can agree that doing this kind of configuration in a commercial cloud cluster is sufficient to adhere to the privacy rules, it’s going to make getting into this data much easier and less time-consuming.”
A Search Solution
Once it gets easier to get into the data, says Benjamin Langmead, researchers will also need new tools to help them find what they’re looking for. Langmead compares the current state of affairs in these databases to the earliest days of the internet, before the invention of search engines.
“This is an analogy that doesn’t work too well with undergrads, right?” he says with a laugh. “They don’t remember the days before Google.”
The analogy that works better with the younger set involves Wikipedia. He sometimes asks his students to imagine a situation where looking something up necessitated downloading the entire encyclopedia onto your computer in a compressed file and then decompressing it—and then guessing as to which mix of search terms might turn up relevant material.
“In some ways, it’s still early days in this field,” Langmead says. “We need something that lets people leverage subsets of this data in easier ways.”
The idea Langmead is working on isn’t fully conceptualized yet, and the end product won’t be nearly as seamless as Google is for laypeople. The strategy he is looking at would organize sequencing data into hubs built around the most popular and important topics researchers are exploring.
“It’s probably going to end up where there is one hub where people can go ask questions about differential gene expression and then maybe there is another one where people can go and ask about rare genetic variations,” he explains. “It needs to be something that can be used by the kind of person who’s wearing a lab coat and doesn’t have any special computational training. We need to make these archives easier for those typical biologists to use.”