Equimolar CoCrNi is driven towards a long-range structure with transformation characteristics similar to that of a strain glass alloy due to the specific stoichiometry and applied aging conditions. This work illustrates the frustrated and kinetically arrested state of this alloy, which develops nano-size, single-phase, isostructural ordered domains at temperatures above 1273 K within a matrix of solid solution. Upon aging at lower temperatures, both atomistic simulation and TEM investigation demonstrate the chemical sensitivity of the matrix by localized symmetry changes which suppress any long-range transformation, mirroring the kinetics observed in strain-glass alloys. Careful quantification of experimental and simulated diffraction patterns from various aging conditions reveal the degree of order in CoCrNi to increase given longer aging times, with achievement of longer domain length scales only when subjected to temperatures below 873 K. This evidence indicates a kinetically constrained, chemically sensitive transition from a disordered fcc to a partially ordered, lower symmetry structure given adequate aging time and temperature. Magnetic effects on the transformation are dictated on the specific alloy stoichiometry and aging temperature, which act to amplify any effects of the glassy kinetics.
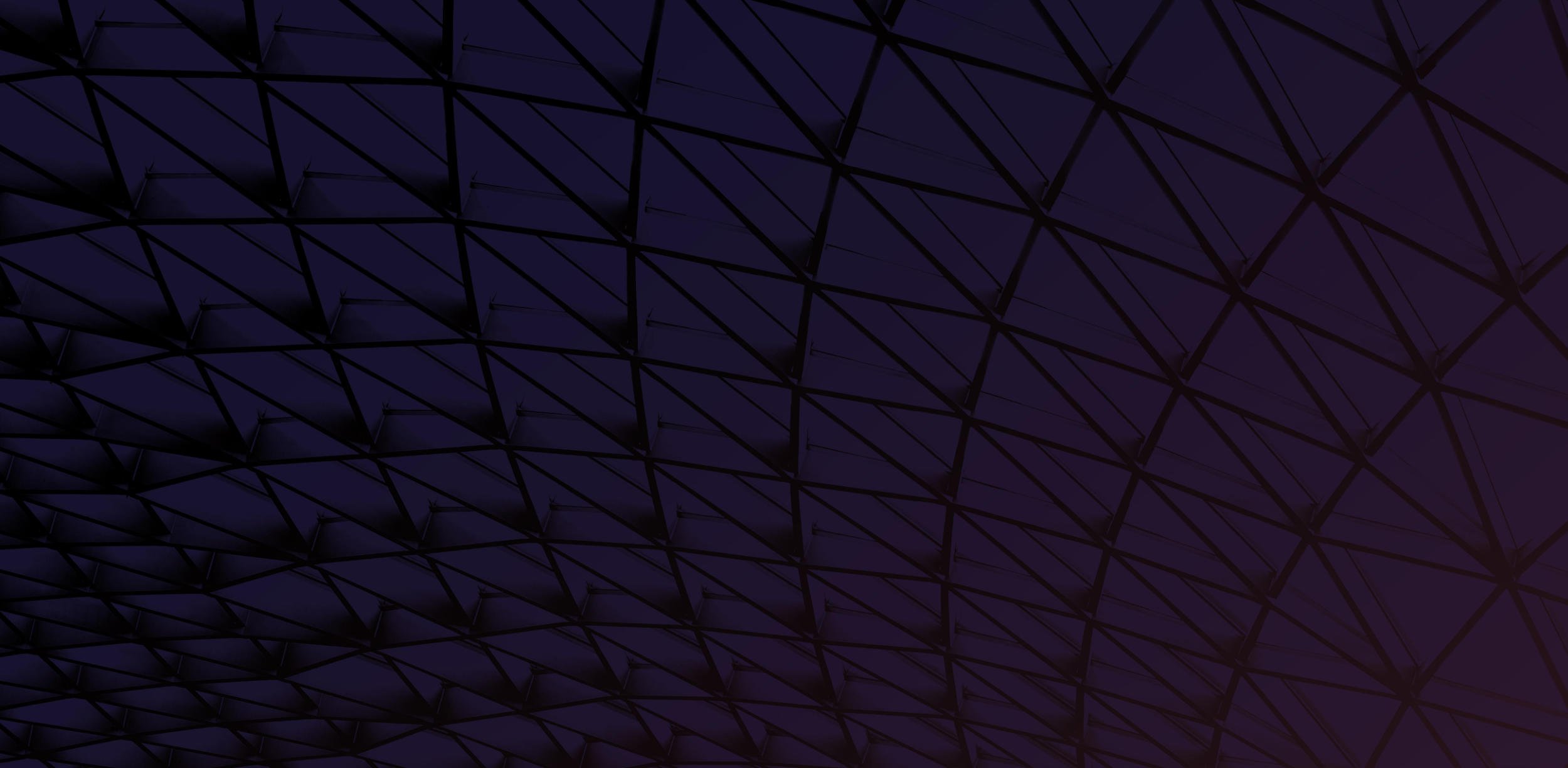
Research Highlights
- Home
- Research Highlights
Elaf A. Anber, David Beaudry, Charlie Brandenburg, Sebastian Lech, Lavina Backman, Daniel L. Foley, Emily L. Wang, Michael Joseph Waters, Loic Perriere, Jean- Philippe Couzinie, James M. Rondinelli, Elizabeth Opila, Mitra L. Taheri
Refractory high entropy alloys (RHEAs) hold the promise of superior mechanical properties at high temperatures but are plagued by a lack of oxidation resistance. In this work, the role of Al additions (4.8, and 13 at.%) to HfNbTaTiZr is explored as a means of improving protective oxide scale formation in RHEAs. Oxide formation was resolved using STEM-EDS/EELS, precession electron diffraction, and computational predictions of formation energy. Our results show that oxides with large negative formation energies (i.e., HfO2/ZrO2) always formed near the metal/oxide interface while Al2O3 and oxides with less negative formation energies formed near the surface. The addition of Al prevented pesting in both alloys and formed Al2O3 with 13 at.% Al. While the Al2O3 formed was not continuous, we report the lowest threshold yet to prevent pesting with only 4.8 at.% Al added. These findings provide guidance to future alloy development of Al2O3-forming alloys.
Jonathan D Hollenbach, Cassandra M Pate, Haili Jia, James L Hart, Paulette Clancy, Mitra L Taheri
In-situ Electron Energy Loss Spectroscopy (EELS) is an instrumental technique that has traditionally been used to understand how the choice of materials processing has the ability to change local structure and composition. However, more recent advances to observe and react to transient changes occurring at the ultrafast timescales that are now possible with EELS and Transmission Electron Microscopy (TEM) will require new frameworks for characterization and analysis. We describe a machine learning (ML) framework for the rapid assessment and characterization of in operando EELS Spectrum Images (EELS-SI) without the need for many labeled training datapoints as typically required for deep learning classification methods. By embedding computationally generated structures and experimental datasets into an equivalent latent space through Variational Autoencoders (VAE), we effectively predict the structural changes at latency scales relevant to closed-loop processing within the TEM. The framework described in this study is a critical step in enabling automated, on-the-fly synthesis and characterization which will greatly advance capabilities for materials discovery and precision engineering of functional materials at the atomic scale.